Diverse Video Generation from a Single Video
CoRR(2022)
摘要
GANs are able to perform generation and manipulation tasks, trained on a single video. However, these single video GANs require unreasonable amount of time to train on a single video, rendering them almost impractical. In this paper we question the necessity of a GAN for generation from a single video, and introduce a non-parametric baseline for a variety of generation and manipulation tasks. We revive classical space-time patches-nearest-neighbors approaches and adapt them to a scalable unconditional generative model, without any learning. This simple baseline surprisingly outperforms single-video GANs in visual quality and realism (confirmed by quantitative and qualitative evaluations), and is disproportionately faster (runtime reduced from several days to seconds). Our approach is easily scaled to Full-HD videos. We also use the same framework to demonstrate video analogies and spatio-temporal retargeting. These observations show that classical approaches significantly outperform heavy deep learning machinery for these tasks. This sets a new baseline for single-video generation and manipulation tasks, and no less important -- makes diverse generation from a single video practically possible for the first time.
更多查看译文
关键词
single video,generation
AI 理解论文
溯源树
样例
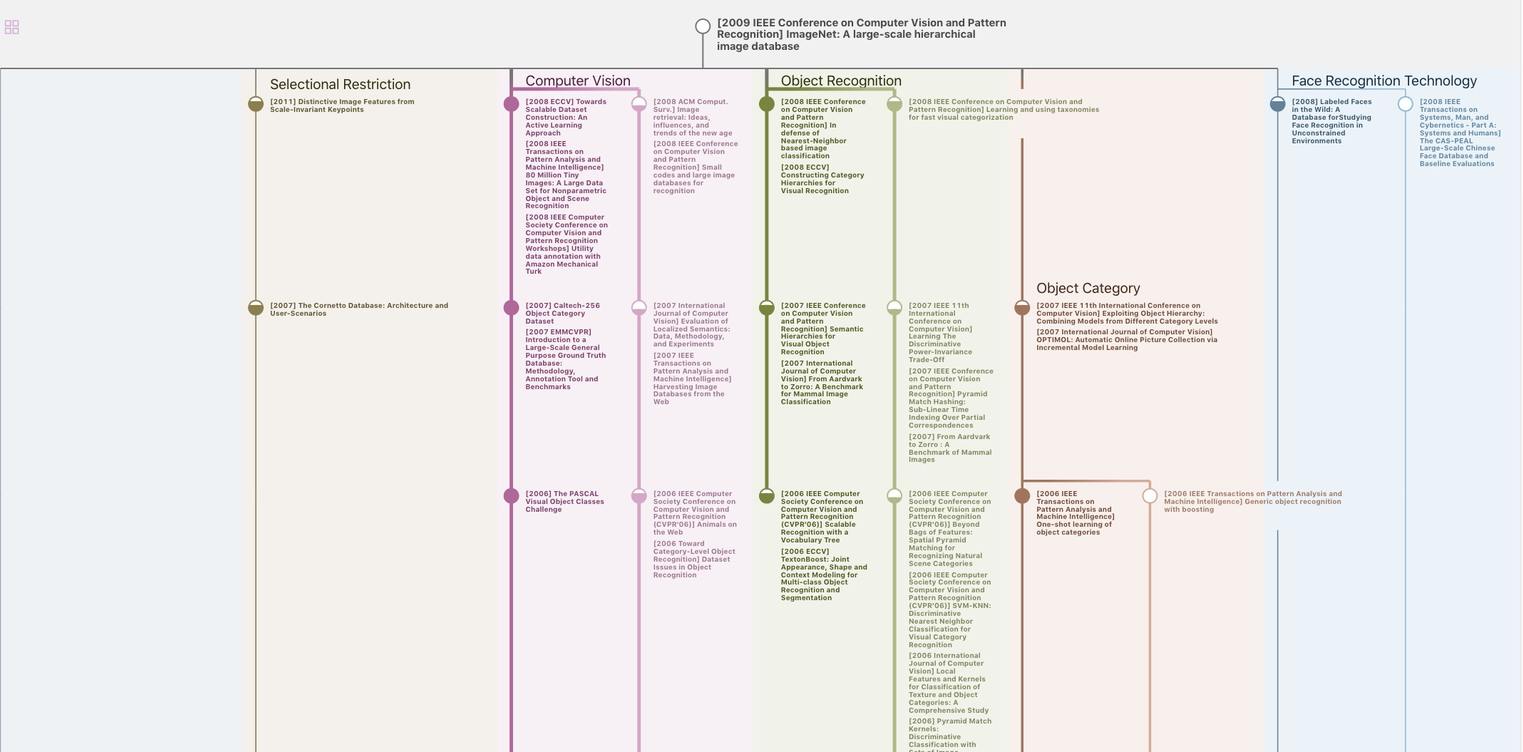
生成溯源树,研究论文发展脉络
Chat Paper
正在生成论文摘要