Nonintrusive Load Monitoring Using an LSTM With Feedback Structure
IEEE TRANSACTIONS ON INSTRUMENTATION AND MEASUREMENT(2022)
摘要
Many non-intrusive load monitoring (NILM) studies use high-frequency data to classify the device's ON/OFF state. However, these approaches cannot be applied in real-world situations due to increased network traffic and database capacity issues. For these reasons, when trying to perform NILM with low-frequency data, the power usage pattern that changes over time disappears and features cannot be properly obtained to classify devices. In this article, we propose a novel NILM model that can learn datasets with imbalanced data classes. The model extracts features using long-short term memory (LSTM) and improve the feature representation ability of LSTM through the feedback of predictions. The experiment is conducted using the REDD dataset and the Living-lab validation dataset. In the REDD dataset, the proposed method outperforms conventional methods 10%-20% on the Majority device and 50%-60% on the Minority device. Living-lab validation results show that the performance of the proposed method outperforms other previously proposed NILM systems in low-frequency data and can be applied to real-world NILM situations.
更多查看译文
关键词
Encoding,Feature extraction,Performance evaluation,Power demand,Data models,Computer architecture,Time series analysis,Appliance identification,energy disaggregation,load management,long short-term memory (LSTM),natural evolution strategies (NES),nonintrusive load monitoring (NILM)
AI 理解论文
溯源树
样例
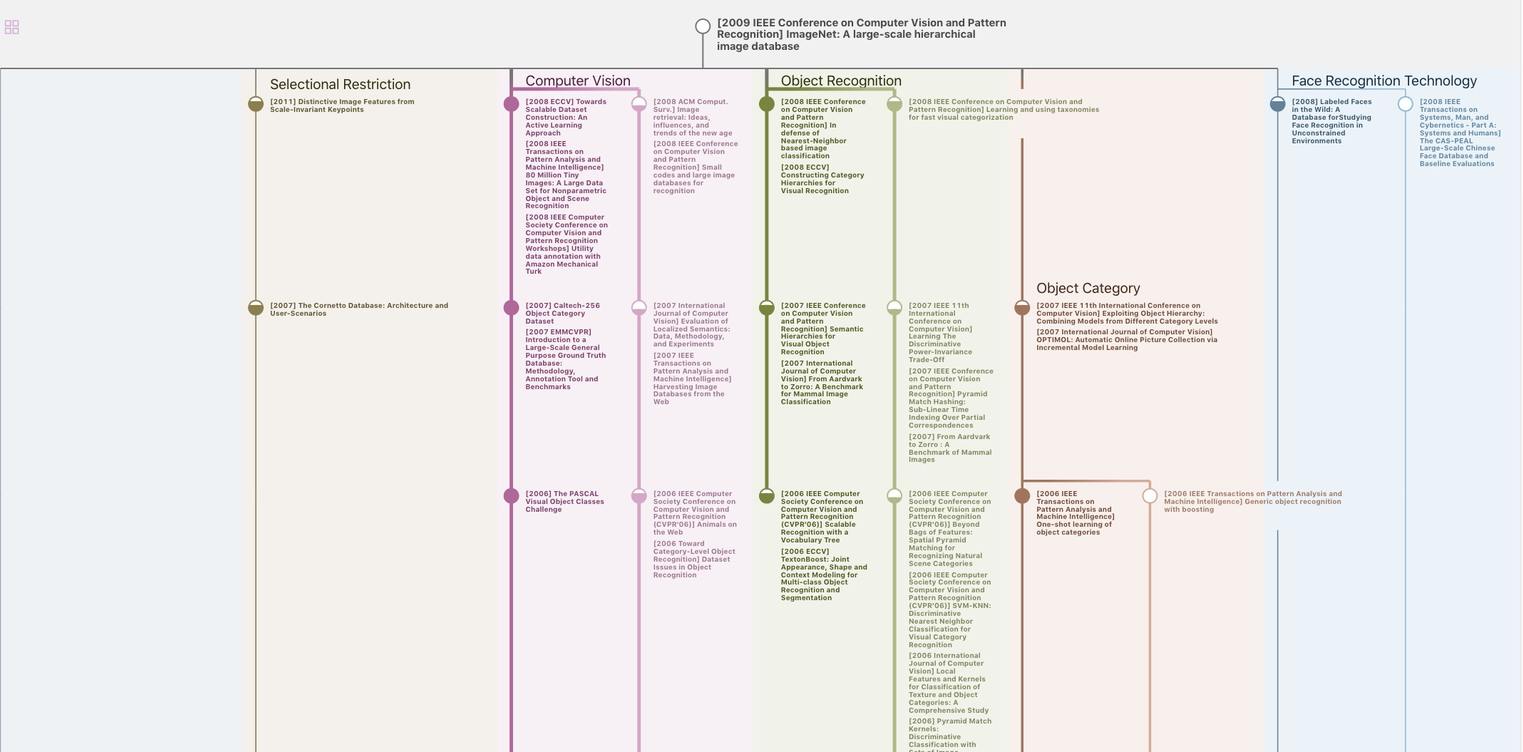
生成溯源树,研究论文发展脉络
Chat Paper
正在生成论文摘要