Statistica Sinica Preprint No: SS-2020-0340
semanticscholar(2021)
摘要
Frequentist Model Averaging for the Nonparametric Model Abstract: This paper develops an optimal frequentist model averaging approach for estimating the unknown conditional mean function in the nonparametric additive model when the covariates and the degree of smoothing are subject to uncertainty. Our weight choice criterion selects model weights by minimising a plug-in estimator of the risk of the model average estimator under a squared error loss function. We derive the convergence rate of the model weights obtained from our proposed method to the infeasible optimal weights, and prove that the resultant model average estimators are asymptotically optimal. An extension to the additive autoregressive model for time series data is also considered. Our simulation analysis shows that the proposed model average estimators can signif-icantly outperform several commonly used model selection estimators and their model averaging counterparts in terms of mean squared error in a large part of the parameter space. We further illustrate our methods in two real data studies.
更多查看译文
AI 理解论文
溯源树
样例
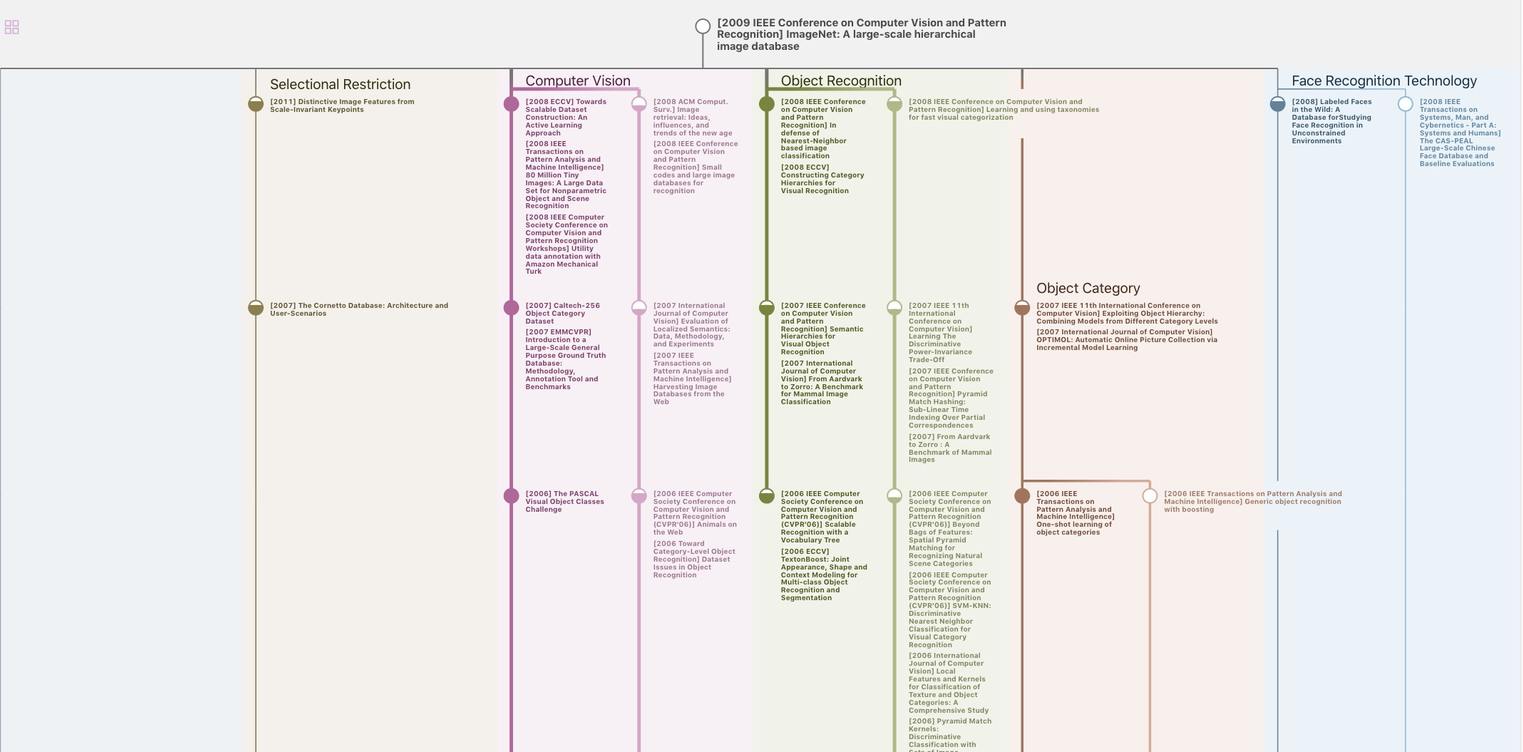
生成溯源树,研究论文发展脉络
Chat Paper
正在生成论文摘要