Evaluation of computational models of infant language development against robust empirical data from meta-analyses: what, why, and how?
semanticscholar(2021)
摘要
Computational models of child language development can help us understand the cognitive underpinnings of the language learning process. One advantage of computational modeling is that is has the potential to address multiple aspects of language learning within a single learning architecture. If successful, such integrated models would help to pave the way for a more comprehensive and mechanistic understanding of language development. However, in order to develop more accurate, holistic, and hence impactful models of infant language learning, the research on models also requires model evaluation practices that allow comparison of model behavior to empirical data from infants across a range of language capabilities. Moreover, there is a need for practices that can compare developmental trajectories of infants to those of models as a function of language experience. The present study aims to take the first steps to address these needs. More specifically, we will introduce the concept of comparing models with large-scale cumulative empirical data from infants, as quantified by meta-analyses conducted across a large number of individual behavioral studies. We start by formalizing the connection between measurable model and human behavior, and then present a basic conceptual framework for meta-analytic evaluation of computational models together with basic guidelines intended as a starting point for later work in this direction. We exemplify the meta-analytic model evaluation approach with two modeling experiments on infant-directed speech preference and native/non-native vowel discrimination. We also discuss the advantages, challenges, and potential future directions of meta-analytic evaluation practices.
更多查看译文
AI 理解论文
溯源树
样例
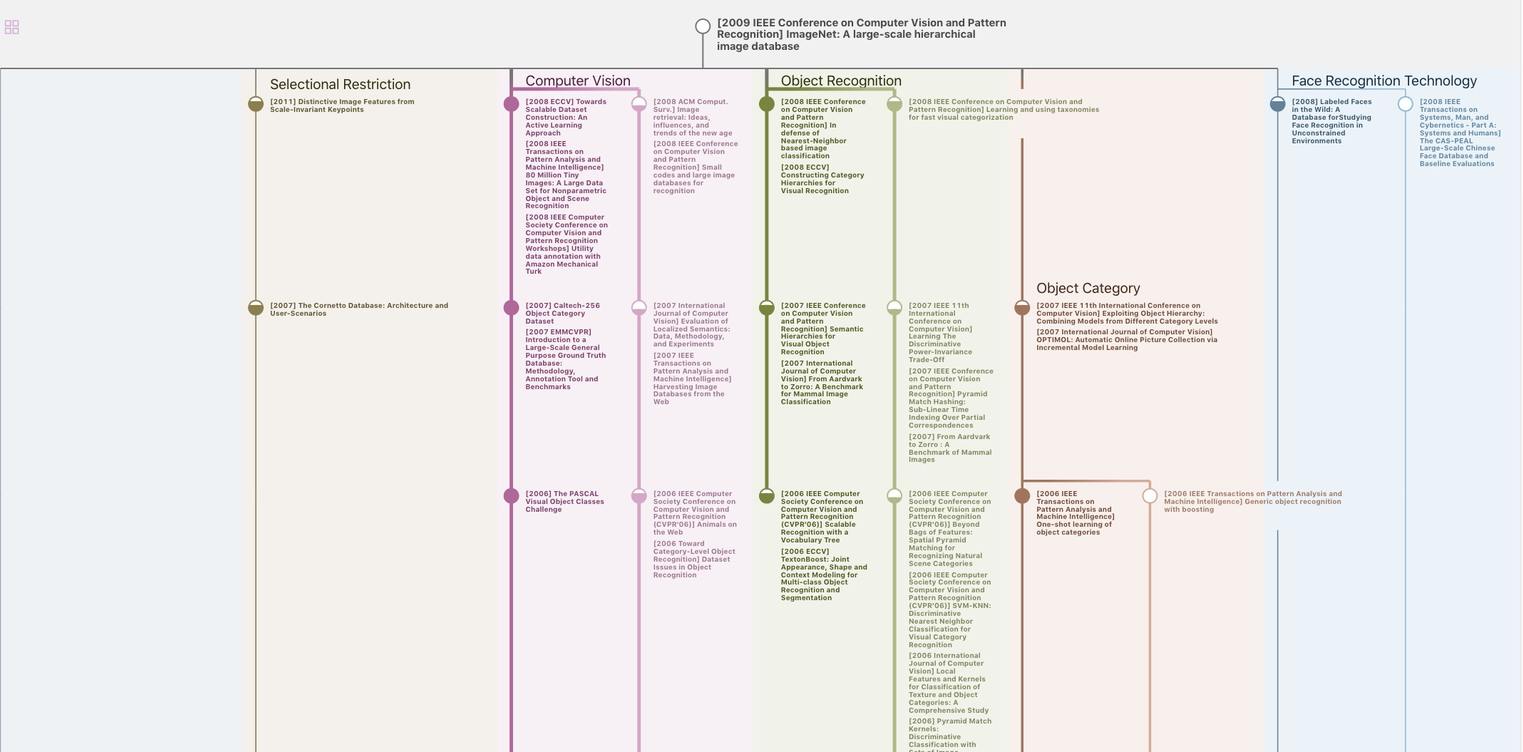
生成溯源树,研究论文发展脉络
Chat Paper
正在生成论文摘要