Sparse and Robust RRAM-based Efficient In-memory Computing for DNN Inference.
2022 IEEE International Reliability Physics Symposium (IRPS)(2022)
摘要
Resistive random-access memory (RRAM)-based in-memory computing (IMC) recently became a promising paradigm for efficient deep neural network acceleration. The multi-bit RRAM arrays provide dense storage and high throughput, whereas the physical non-ideality of the RRAM devices impairs the retention characteristics of the resistive cells, leading to accuracy degradation. On the algorithm side, various hardware-aware compression algorithms have been proposed to accelerate the computation of deep neural networks (DNNs) computation. However, most recent works individually consider the "model compression" and "hardware robustness". The impact of the RRAM non-ideality for the sparse model is still underexplored. In this work, we present a novel temperature-resilient RRAM-based IMC scheme for reliable DNN inference hardware. Based on the measurement from a 90nm RRAM prototype chip, we first explore the robustness of the sparse model under the different operating temperatures (25 degrees C to 85 degrees C). On top of that, we propose a novel robustness-aware pruning algorithm, then further enhance the model robustness with a novel sparsity-aware noise-injected fine-tuning. The proposed scheme achieves >92% CIFAR-10 inference accuracy after one-day operation, which is >37% higher than the state-of-art method.
更多查看译文
关键词
Convolutional neural network,in-memory computing,multilevel RRAM,data retention,structured pruning
AI 理解论文
溯源树
样例
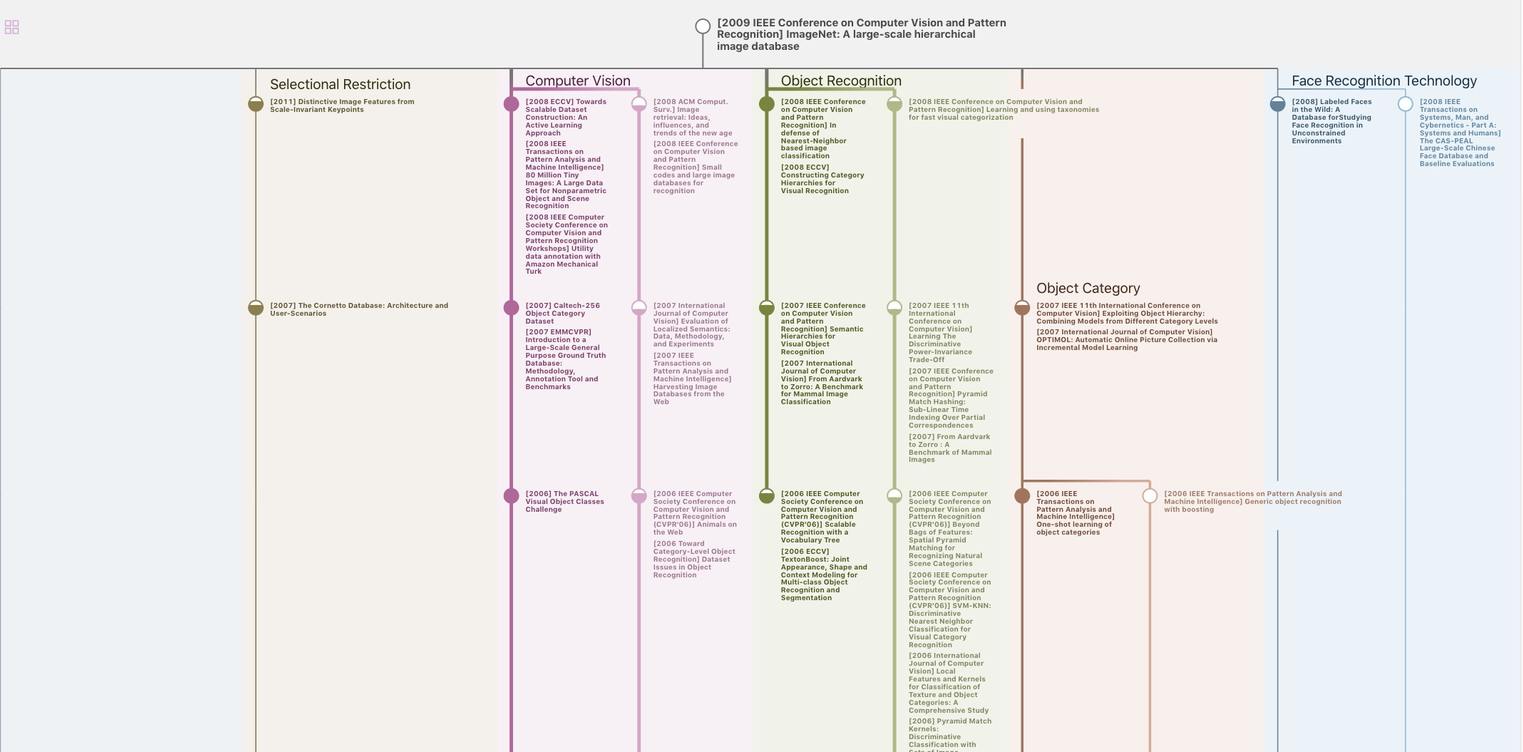
生成溯源树,研究论文发展脉络
Chat Paper
正在生成论文摘要