Sepsis Labels Defined by Claims-Based Methods Are Ill-Suited for Training Machine Learning Algorithms.
Clinical microbiology and infection the official publication of the European Society of Clinical Microbiology and Infectious Diseases(2022)
摘要
Accurate sepsis diagnosis in emergency department (ED) patients is of paramount importance for adequate treatment to improve clinical outcome. At the ED, no reference standard definition for sepsis is available. This impedes the development of machine learning (ML) models for automated sepsis diagnosis at the ED. Currently, ML algorithms are predominantly trained on data labeled by claims-based methods (e.g. International Classification of Diseases (ICD)-coding) [[1]Fleuren L.M. Klausch T.L.T. Zwager C.L. Schoonmade L.J. Guo T. Roggeveen L.F. et al.Machine learning for the prediction of sepsis: a systematic review and meta-analysis of diagnostic test accuracy.Intensive Care Med. 2020; 46: 383-400Crossref PubMed Scopus (146) Google Scholar]. These approaches have severe limitations [[2]Donnelly J.P. Dai Y. Colantonio L.D. Zhao H. Safford M.M. Baddley J.W. et al.Agreement of claims-based methods for identifying sepsis with clinical criteria in the Reasons for Geographic and Racial Differences in Stroke (REGARDS) cohort.BMC Med Res Methodol. 2020; 20: 54Crossref PubMed Scopus (1) Google Scholar]. For instance, labels based on claims-based methods are known to have high specificity, but low sensitivity [[2]Donnelly J.P. Dai Y. Colantonio L.D. Zhao H. Safford M.M. Baddley J.W. et al.Agreement of claims-based methods for identifying sepsis with clinical criteria in the Reasons for Geographic and Racial Differences in Stroke (REGARDS) cohort.BMC Med Res Methodol. 2020; 20: 54Crossref PubMed Scopus (1) Google Scholar]. In the context of automated sepsis diagnosis at the ED, sensitivity is crucial because underdiagnosing a patient with sepsis can have fatal consequences. As algorithms are oblivious to the label's validity, flawed labels can have major consequences on the model's accuracy, resulting in inaccurate diagnosis when used in clinical practice. By lack of a reference stfandard, an endpoint adjudication committee (EAC), consisting of clinical experts, is a proven method to gain consensus on a clinical diagnosis such as sepsis [[3]Rutjes A.W. Reitsma J.B. Coomarasamy A. Khan K.S. Bossuyt P.M. Evaluation of diagnostic tests when there is no gold standard. A review of methods.Heal Technol Assess. 2007; 11 (ix–51)PubMed Google Scholar]. We hypothesized that indeed claims-based methods lack diagnostic accuracy when compared to an EAC-based method. To test this hypothesis, we compared EAC sepsis labels with ICD-10 codes. The EAC consisted of 18 independent experts from a variety of specialisms, including ED specialists, internists, and ICU specialists. The EAC reviewed all ED visits of the University Medical Center Utrecht (UMC Utrecht), Utrecht, the Netherlands for the internal medicine department with suspicion of an infection (SPACE-database, approved by Medical Ethical Committee of the UMC Utrecht, 16/594) between January and April 2018 [[4]Uffen J.W. Oomen P. de Regt M. Oosterheert J.J. Kaasjager K. The prognostic value of red blood cell distribution width in patients with suspected infection in the emergency department.BMC Emerg Med. 2019; 19: 76Crossref PubMed Scopus (18) Google Scholar]. The EAC received all clinical information of the patients, including ED data and follow-up data (EAC group). Subsequently, we labeled the same patients based on the ICD-10 codes that were given to them in regular care; we positively labeled the ones that contained the term ‘sepsis’ in the title (ICD-10 group). In total, the EAC labeled 397 patients. In the EAC group 77 (19.4%) patients were identified as having sepsis, while this was only 12 (3.0%) for the ICD-10 group. To investigate underdiagnosing in the ICD-10 group, we compared the patients labeled negative in both groups. Patients in the ICD-10 group were more likely to be admitted to the hospital (161 (50.3%) vs. 226 (58.7%), p = 0.031) and had a higher quick Sequential Organ Failure Assessment (qSOFA) ≥2 count (7 (2.2%) vs. 20 (5.2%), p = 0.061, not significant) when compared to the EAC group (Table 1). Interestingly, only 7 of the 12 patients who were identified as having sepsis with the ICD-10 labels overlapped with the EAC labels. Characteristics of positive-labeled patients in both groups did not show any significant differences (Table S1).Table 1Characteristics of patients labeled negative for sepsis by the EAC or the ICD-10EAC (n = 320/397)ICD-10 (n = 385/397)SignificanceAge, years, mean (SD)57.4 (16.1)58.4 (15.8)0.418Sex, male ED visits (%)175 (54.7)210 (54.5)1.000CCI, mean (SD)4.4 (3.0)4.6 (3.0)0.444ED visits with qSOFA ≥2, count (%)7 (2.2)20 (5.2)0.061ED specialty, n (%)HaematologyInternal Medicine –Nephrology –Oncology -Other60 (18.8)99 (30.9)59 (18.4)56 (17.5)46 (14.4)70 (18.2)124 (32.2)66 (17.1)71 (18.4)54 (14.0)0.984Immunocompromised, n (%)120 (37.6)141 (36.7)0.867Death in 30 days after ED visit, n (%)7 (2.2)15 (3.9)0.279Admission, n (%)161 (50.3)226 (58.7)0.031ICU admissionaOnly patients that were applicable for ICU admission are shown., n (%)8 (2.6)13 (3.5)0.615Length of hospital stay, days (SD)6.4 (7.7)7.6 (9.8)0.182Significance level of p < 0.05 was deemed significant.CCI, Charlson Comorbidity Index; EAC, endpoint adjudication committee; ED, emergency department; ICD, International Classification of Diseases; ICU, intensive care unit; SD, standard deviation.a Only patients that were applicable for ICU admission are shown. Open table in a new tab Significance level of p < 0.05 was deemed significant. CCI, Charlson Comorbidity Index; EAC, endpoint adjudication committee; ED, emergency department; ICD, International Classification of Diseases; ICU, intensive care unit; SD, standard deviation. We found that the ED sepsis incidence differs significantly depending on the labeling method (EAC vs. ICD-10). More importantly, our data suggest that ICD-10 coding is prone to miss sepsis cases. As a consequence, ML models trained on data labeled by claims-based methods are therefore unintentionally trained to miss sepsis patients in clinical practice, thereby preventing adequate treatment for patients in need. These findings are strengthened by other studies that describe ICD-10 codes to lack sensitivity when compared with methods that use objective clinical data to define sepsis labels [[5]Rhee C. Jentzsch M.S. Kadri S.S. Seymour C.W. Angus D.C. Murphy D.J. et al.Variation in identifying sepsis and organ dysfunction using administrative versus electronic clinical data and impact on hospital outcome comparisons.Crit Care Med. 2019; 47: 493-500Crossref PubMed Scopus (30) Google Scholar]. For training sepsis models, timelines are of crucial importance. In this context, two matters should be kept apart, namely training the model on available data and implementation of the algorithm in clinical practice. ED algorithms should be trained with data that is available at the ED, otherwise an algorithm would be useless due to missing variables during the ED visit. However, labeling the outcome of sepsis patients to train the model on, is independent of this moment in time and should, above all, be done correctly, i.e. if in retrospect the diagnosis at the ED turned out to be sepsis after all and the first ICD-10 coding did not indicate this, the model should be able to ‘catch’ this patient when it is applied in clinical practice. Overall, providing additional retrospective data can thus improve the label's quality resulting in better identification of sepsis patients who visit the ED, including the ones that were ‘missed’ by ICD-10 coding. Most importantly, models trained on high quality outcome labels, based on the total clinical course, will still be able to identify sepsis patients based on only data available at the ED. For future studies that develop models for early sepsis diagnosis, we therefore encourage ML experts to use EAC-labels. Although we acknowledge that an EAC is labor-intensive and experts are influenced by their own concept of sepsis, an EAC combines clinical experience and nuance in unstructured clinical data with the most recent guidelines and is therefore more capable to better capture the clinical picture beyond registration coding. A model trained on EAC-labels will thus provide a better reflection of reality, thereby increasing the model's diagnostic accuracy and missing less sepsis cases that need our urgent care. MSAN is supported by a PhD fellowship from SkylineDx, Rotterdam; and SH is supported by a fellowship from Abbott Diagnostics. This research received no external funding. Conceptualization (TH, MN), methodology (TH, MN, SH), formal analysis (TH, MN), investigation (TH, SH), data curation (TH, MN), writing original draft (TH, MN), writing reviewing and editing (TH, MN, WS, JO, SH, KK), supervision (WS, JO, SH, KK). The following are the Supplementary data to this article: Download .docx (.11 MB) Help with docx files Multimedia component 1 Download .docx (.01 MB) Help with docx files Multimedia component 1
更多查看译文
AI 理解论文
溯源树
样例
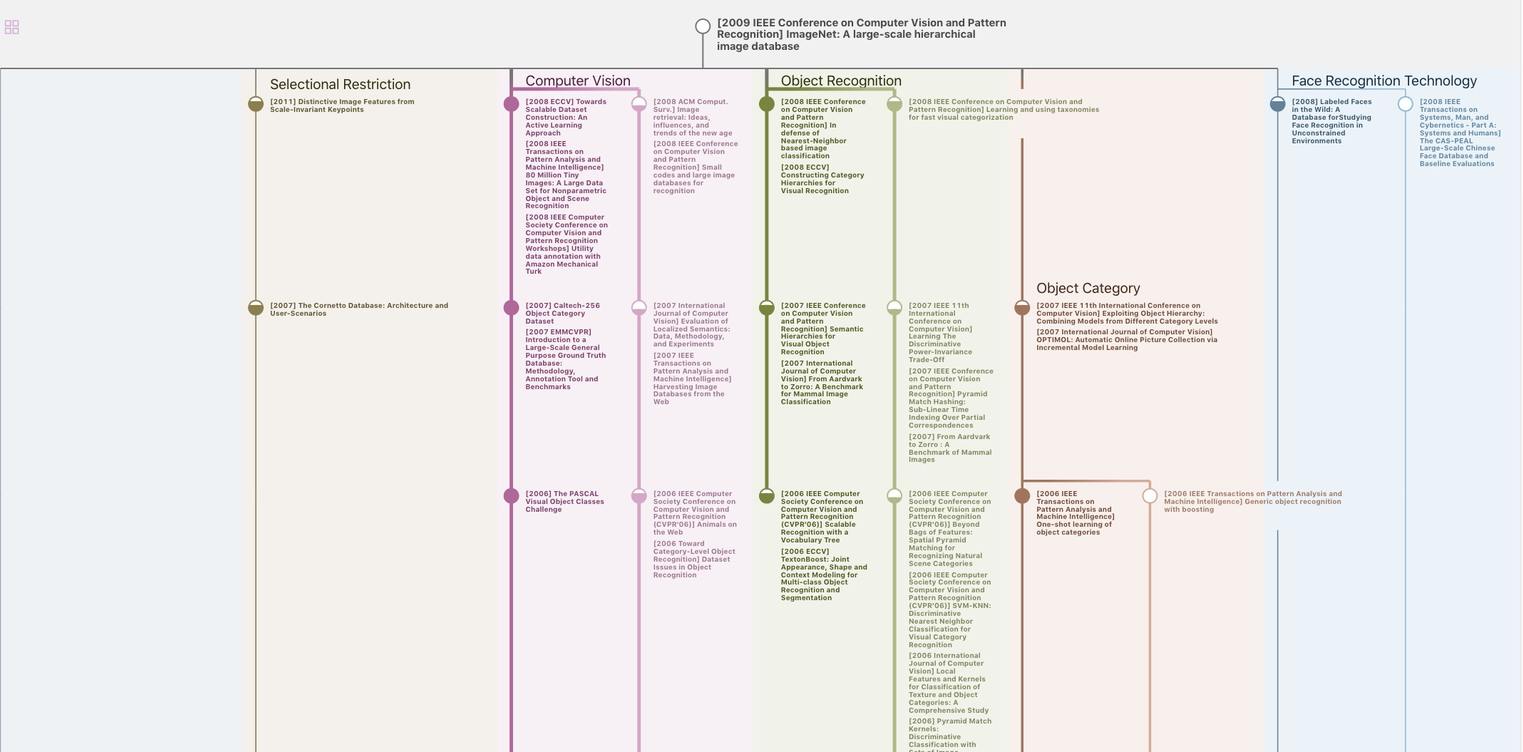
生成溯源树,研究论文发展脉络
Chat Paper
正在生成论文摘要