Learning Neural Networks Without Lazy Weights.
International Conference on Big Data and Smart Computing(2022)
摘要
Various approaches have been suggested for the regularization of neural networks, including the well-known Dropout and Dropconnect, which are simple and efficient to implement and therefore have been widely used. However, there is a risk of loss of well-trained weights when dropping nodes or weights randomly. In this paper, we propose a regularization method that preserves well-trained weights and removes poorly trained weights. This was motivated by the observation that the trained weights become further trained. We define these as eager weights whereas the opposite as lazy weights. On every weight update, the distribution of the changes in weight values is examined, and the lazy weights are removed layer-wise. The results demonstrate that the proposed method has a faster convergence rate, avoids overfitting, and outperforms competing methods on the classification of benchmark datasets.
更多查看译文
关键词
Neural networks,Regularization,Overfitting,Deep learning
AI 理解论文
溯源树
样例
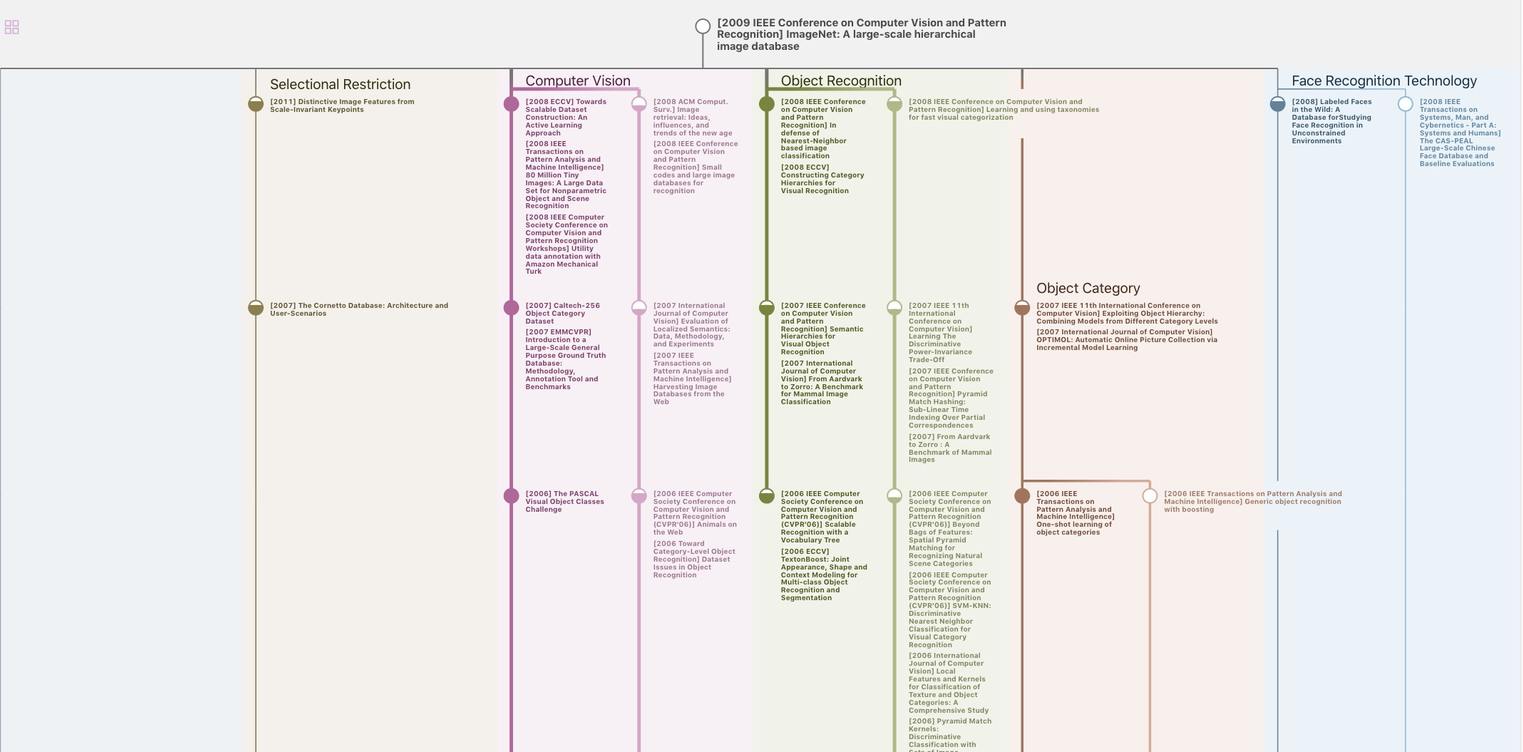
生成溯源树,研究论文发展脉络
Chat Paper
正在生成论文摘要