CAD: Co-Adapting Discriminative Features for Improved Few-Shot Classification
IEEE Conference on Computer Vision and Pattern Recognition(2022)
Abstract
Few-shot classification is a challenging problem that aims to learn a model that can adapt to unseen classes given a few labeled samples. Recent approaches pre-train a feature extractor, and then fine-tune for episodic metalearning. Other methods leverage spatial features to learn pixel-level correspondence while jointly training a classifier. However, results using such approaches show marginal improvements. In this paper, inspired by the transformer style self-attention mechanism, we propose a strategy to cross-attend and re-weight discriminative features for fewshot classification. Given a base representation of support and query images after global pooling, we introduce a single shared module that projects features and cross-attends in two aspects: (i) query to support, and (ii) support to query. The module computes attention scores between features to produce an attention pooled representation of features in the same class that is later added to the original representation followed by a projection head. This effectively re-weights features in both aspects (i & ii) to produce features that better facilitate improved metric-based metalearning. Extensive experiments on public benchmarks show our approach outperforms state-of-the-art methods by 3%~5%.
MoreTranslated text
Key words
Self-& semi-& meta- Recognition: detection,categorization,retrieval, Representation learning
AI Read Science
Must-Reading Tree
Example
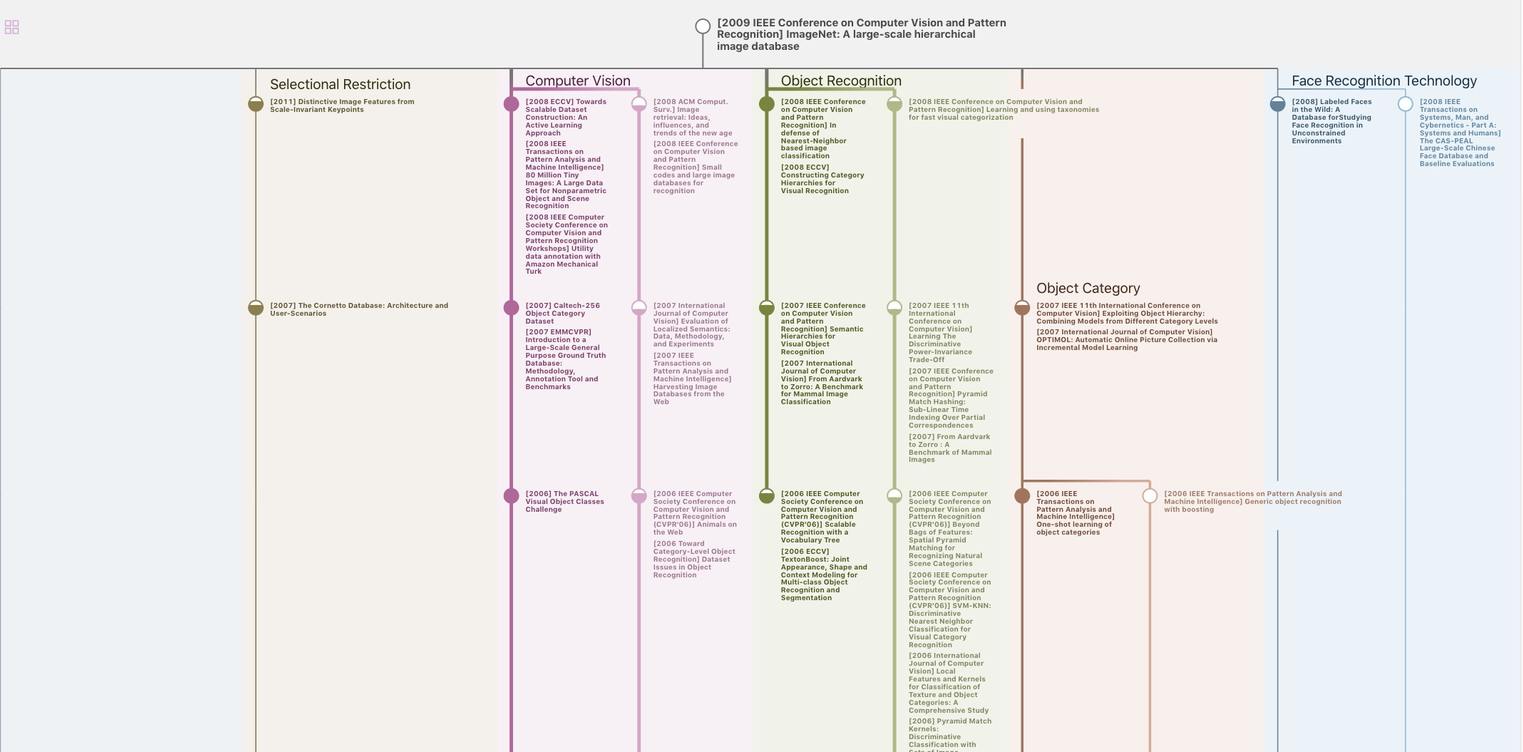
Generate MRT to find the research sequence of this paper
Chat Paper
Summary is being generated by the instructions you defined