Multi-Armed Bandits Learning for Task Offloading in Maritime Edge Intelligence Networks
IEEE transactions on vehicular technology(2022)
摘要
In the context of complex and dynamic marine environment, the offloading of computing tasks for ships of Internet of Things (IoT) users is a very challenging problem considering the different quality of service (QoS) requirements of maritime applications. Mobile edge computing driven by powerful computing capability and edge intelligence is taken as a promising solution, especially for the resource-constrained and delay-sensitive maritime IoT users. In this paper, we study the optimal edge server selection problem for ship IoT users to jointly minimize the latency and energy consumption for task offloading. Specifically, we first propose a novel space-air-ground-edge (SAGE) integrated maritime network architecture to offload computation-intensive IoT services at sea. Then, the latency and energy consumption of data transmission and processing during offloading are modelled. Based on the models, the edge server selection problem is formulated into a Multi-Armed Bandits learning problem, with considering the task latency requirement and energy budget. To achieve the optimal solution, a novel algorithm, referred to as UCB1-ESSS, is developed, which links the latency, energy consumption, and network constraints by introducing both reward and cost. The simulation results show that the proposed algorithm can achieve considerably lower offloading latency and weighted latency-energy cost compared with the traditional algorithms under different QoS requirements, which proves the efficacy of theproposed algorithm.
更多查看译文
关键词
Servers,Internet of Things,Marine vehicles,Task analysis,Energy consumption,Delays,Computational modeling,Computing offloading,edge intelligence,maritime wireless networks,multi-armed bandits,marine ship IoT users
AI 理解论文
溯源树
样例
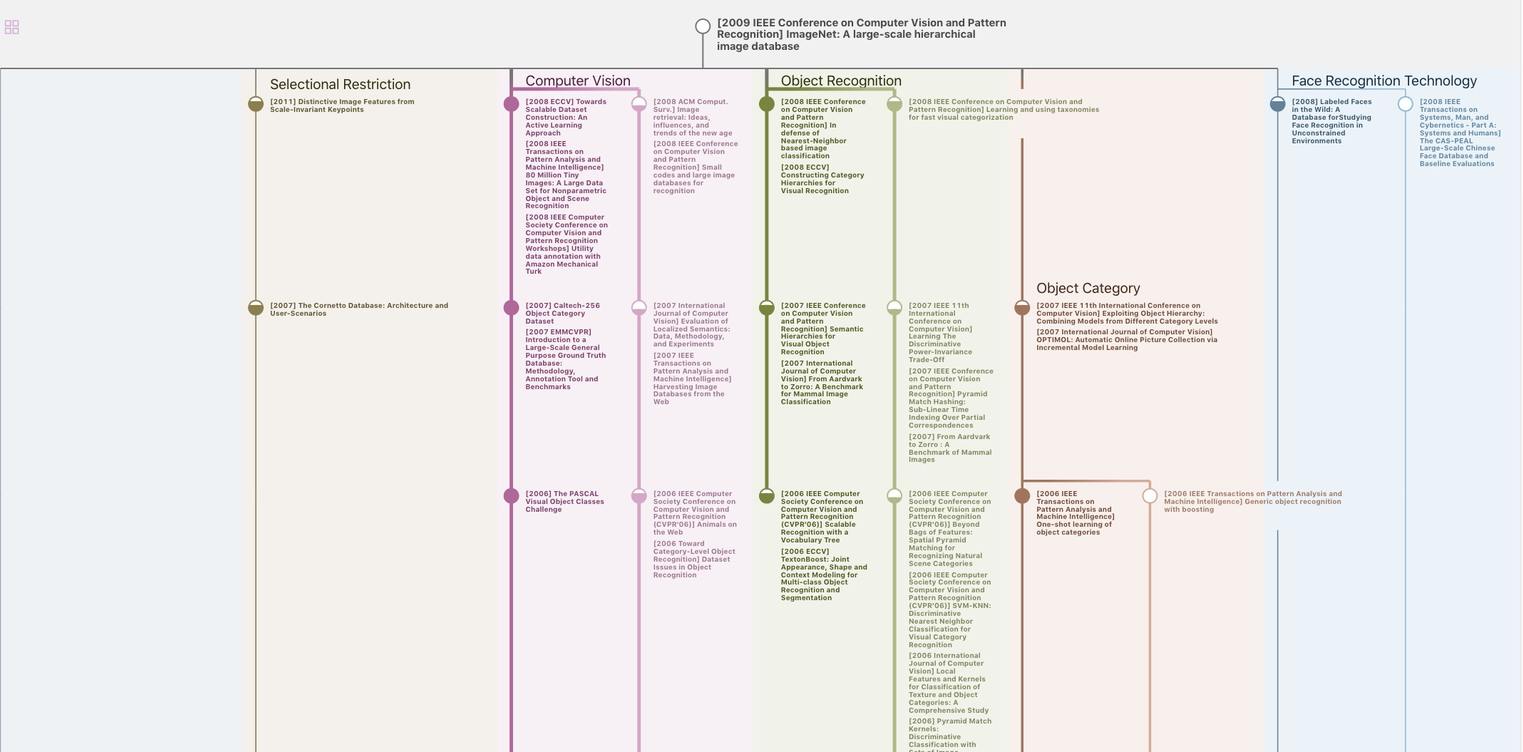
生成溯源树,研究论文发展脉络
Chat Paper
正在生成论文摘要