Dynamic Routing Programming for Power Communication Networks by Recurrent Neural Networks based Reliability Prediction and Particle Swarm Optimization
2019 Scientific Conference on Network, Power Systems and Computing(2019)
Abstract
The coupling Power Communication Network (PCN) has been proven as an effective supplement to modern power grid for carrying various auxiliary services. Conventional fault processing methods for PCN implement short term recovery of services from interruption caused by external risks. However, such methods normally optimize topological structure of routing according to direct communication states. In this paper, we propose a machine learning based method to predict the reliability of nodes as well as links on PCN and dynamically program routing strategy alongside with the variation of external environment. The method comprehensively takes various factors into account as input to a Recurrent Neural Network to predict the reliability of hardware in incoming days. Based on the predicted reliability, a Particle Swarm Optimization method is exploited to optimize the routing to prompt the robustness of services by avoiding path with potential risk. Our experimental analysis on both simulation cases as well as realistic records demonstrates that the proposed dynamic routing programming method can be applied as a medium and long term supplement to existing fault processing methods.
MoreTranslated text
Key words
particle swarm optimization,smart grid,neural network,programming
AI Read Science
Must-Reading Tree
Example
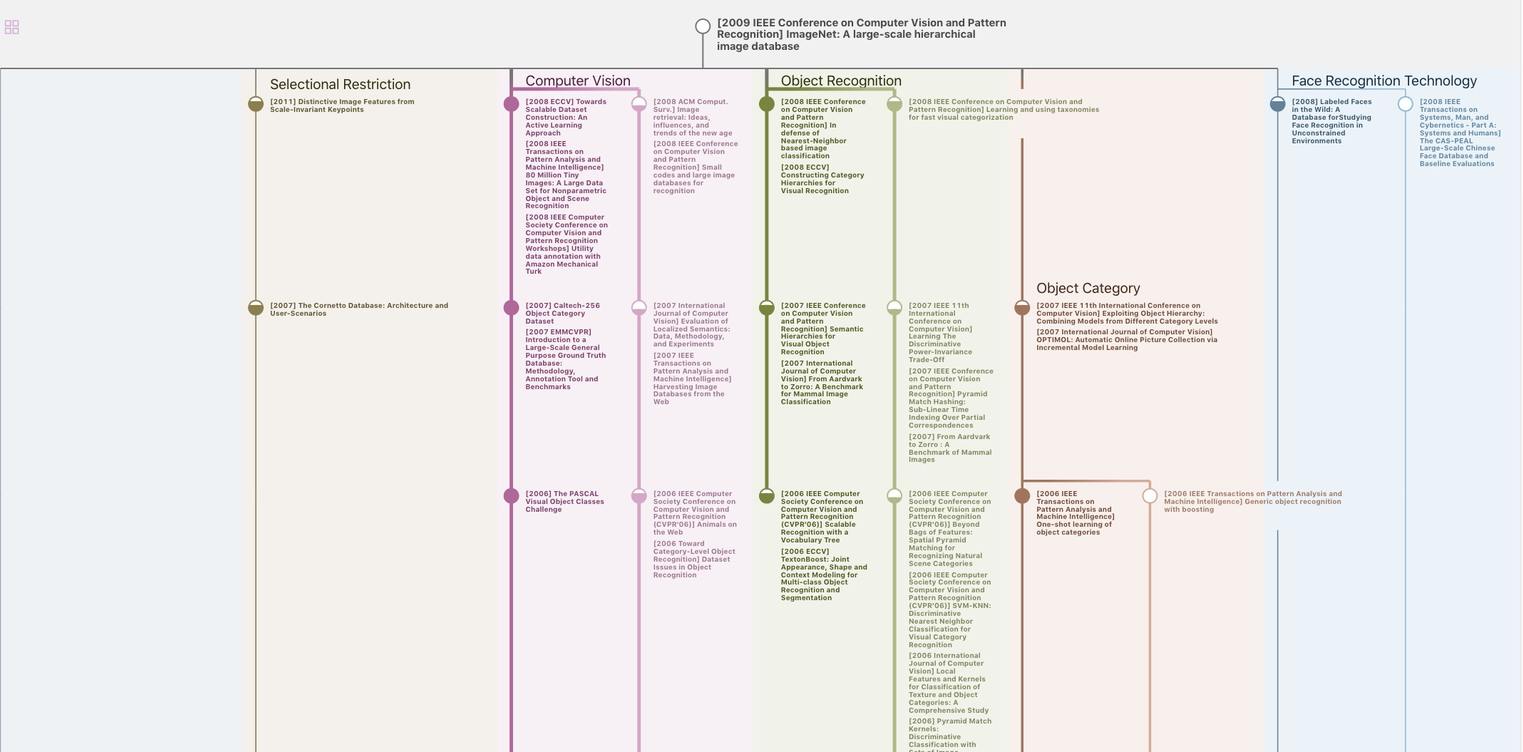
Generate MRT to find the research sequence of this paper
Chat Paper
Summary is being generated by the instructions you defined