An Approach to Improving Anomaly Detection Using Multiple Detectors
2022 16th International Conference on Ubiquitous Information Management and Communication (IMCOM)(2022)
摘要
Anomaly detection performs well in situations where signature (and other rule-based) methods fail; there is no need to identify every threat as long as it is different from the norm. The tradeoff is that anomaly detection often results in a large number of false positives. While previous work has capitalized on the data imbalance problem to train models with only one set of data (one-class classification), few have utilized the limiting set for anything other than testing purposes. This paper seeks to utilize two anomaly detectors: one that is trained on the positive set and one that is trained on the negative set. By utilizing multiple detectors, we can encode more information about each class and ensure that a data point is not only different from one class, but also similar to the other. We present a new approach to anomaly detection and show its effectiveness at reducing false positives with limited effect on detection rates.
更多查看译文
关键词
anomaly detection,class imbalance,one-class classification
AI 理解论文
溯源树
样例
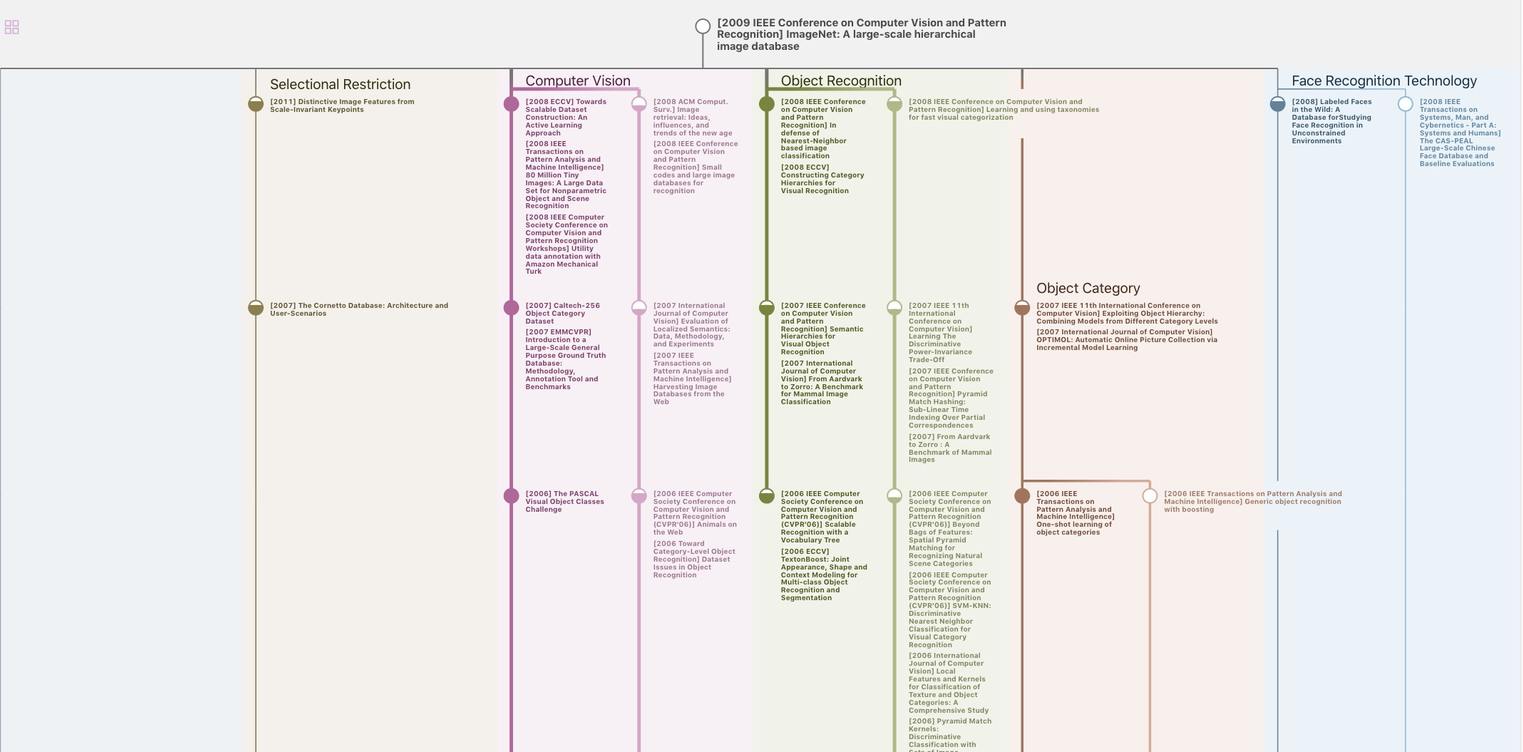
生成溯源树,研究论文发展脉络
Chat Paper
正在生成论文摘要