Missile Guidance with Assisted Deep Reinforcement Learning for Head-on Interception of Maneuvering Target
COMPLEX & INTELLIGENT SYSTEMS(2022)
摘要
In missile guidance, pursuit performance is seriously degraded due to the uncertainty and randomness in target maneuverability, detection delay, and environmental noise. In many methods, accurately estimating the acceleration of the target or the time-to-go is needed to intercept the maneuvering target, which is hard in an environment with uncertainty. In this paper, we propose an assisted deep reinforcement learning (ARL) algorithm to optimize the neural network-based missile guidance controller for head-on interception. Based on the relative velocity, distance, and angle, ARL can control the missile to intercept the maneuvering target and achieve large terminal intercept angle. To reduce the influence of environmental uncertainty, ARL predicts the target’s acceleration as an auxiliary supervised task. The supervised learning task improves the ability of the agent to extract information from observations. To exploit the agent’s good trajectories, ARL presents the Gaussian self-imitation learning to make the mean of action distribution approach the agent’s good actions. Compared with vanilla self-imitation learning, Gaussian self-imitation learning improves the exploration in continuous control. Simulation results validate that ARL outperforms traditional methods and proximal policy optimization algorithm with higher hit rate and larger terminal intercept angle in the simulation environment with noise, delay, and maneuverable target.
更多查看译文
关键词
Reinforcement learning,Missile guidance,Auxiliary learning,Self-imitation learning
AI 理解论文
溯源树
样例
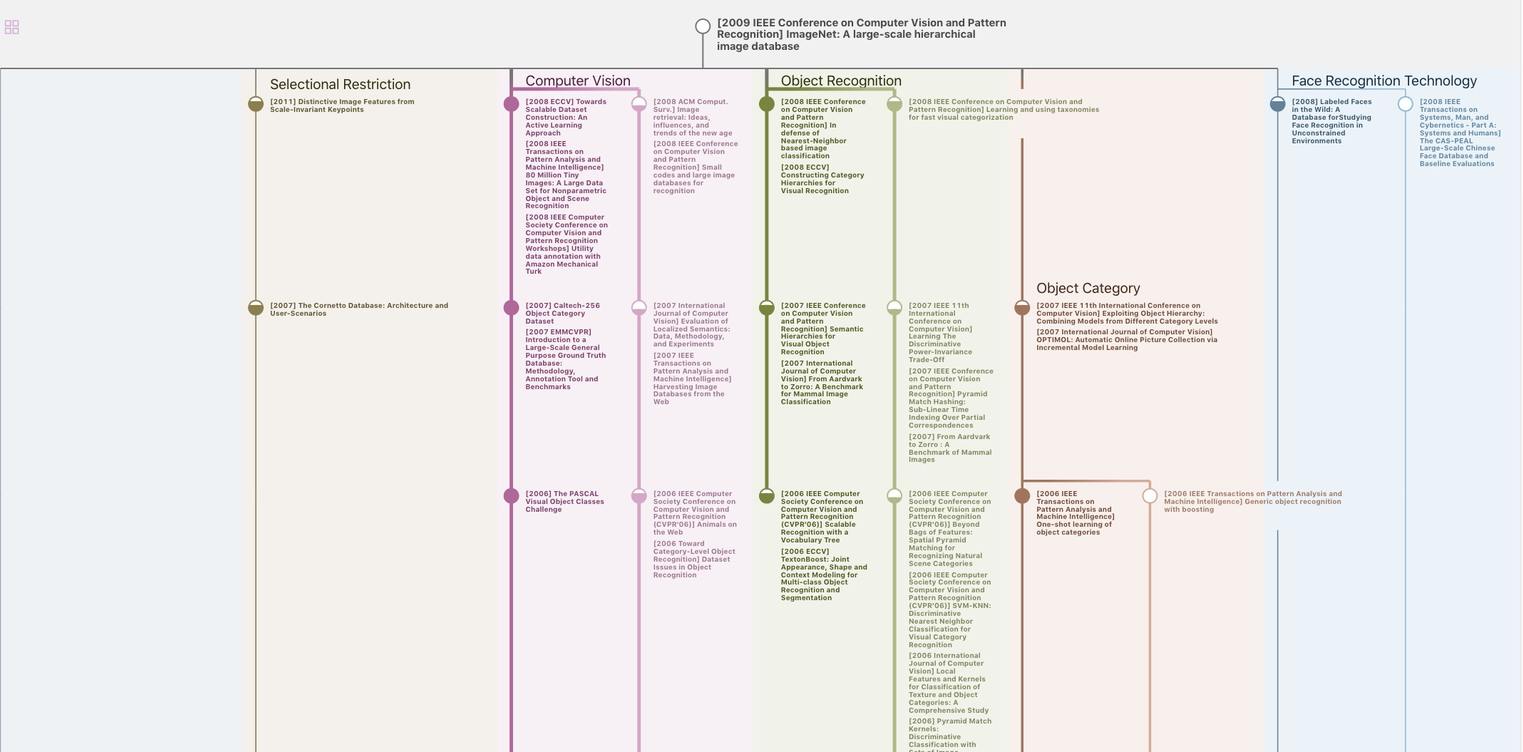
生成溯源树,研究论文发展脉络
Chat Paper
正在生成论文摘要