Camel: A Weakly Supervised Learning Framework For Histopathology Image Segmentation
2019 IEEE/CVF INTERNATIONAL CONFERENCE ON COMPUTER VISION (ICCV 2019)(2019)
摘要
Histopathology image analysis plays a critical role in cancer diagnosis and treatment. To automatically segment the cancerous regions, fully supervised segmentation algorithms require labor-intensive and time-consuming labeling at the pixel level. In this research, we propose CAMEL, a weakly supervised learning framework for histopathology image segmentation using only image-level labels. Using multiple instance learning (MIL)-based label enrichment, CAMEL splits the image into latticed instances and automatically generates instance-level labels. After label enrichment, the instance-level labels are further assigned to the corresponding pixels, producing the approximate pixel-level labels and making fully supervised training of segmentation models possible. CAMEL achieves comparable performance with the fully supervised approaches in both instance-level classification and pixel-level segmentation on CAMELYON16 and a colorectal adenoma dataset. Moreover, the generality of the automatic labeling methodology may benefit future weakly supervised learning studies for histopathology image analysis.
更多查看译文
关键词
time-consuming labeling,weakly supervised learning framework,histopathology image segmentation,image-level labels,multiple instance learning-based label enrichment,latticed instances,instance-level labels,approximate pixel-level labels,segmentation models,instance-level classification,pixel-level segmentation,automatic labeling methodology,histopathology image analysis,cancer diagnosis,cancerous regions,supervised segmentation algorithms,CAMEL framework,CAMELYON16 dataset,colorectal adenoma dataset
AI 理解论文
溯源树
样例
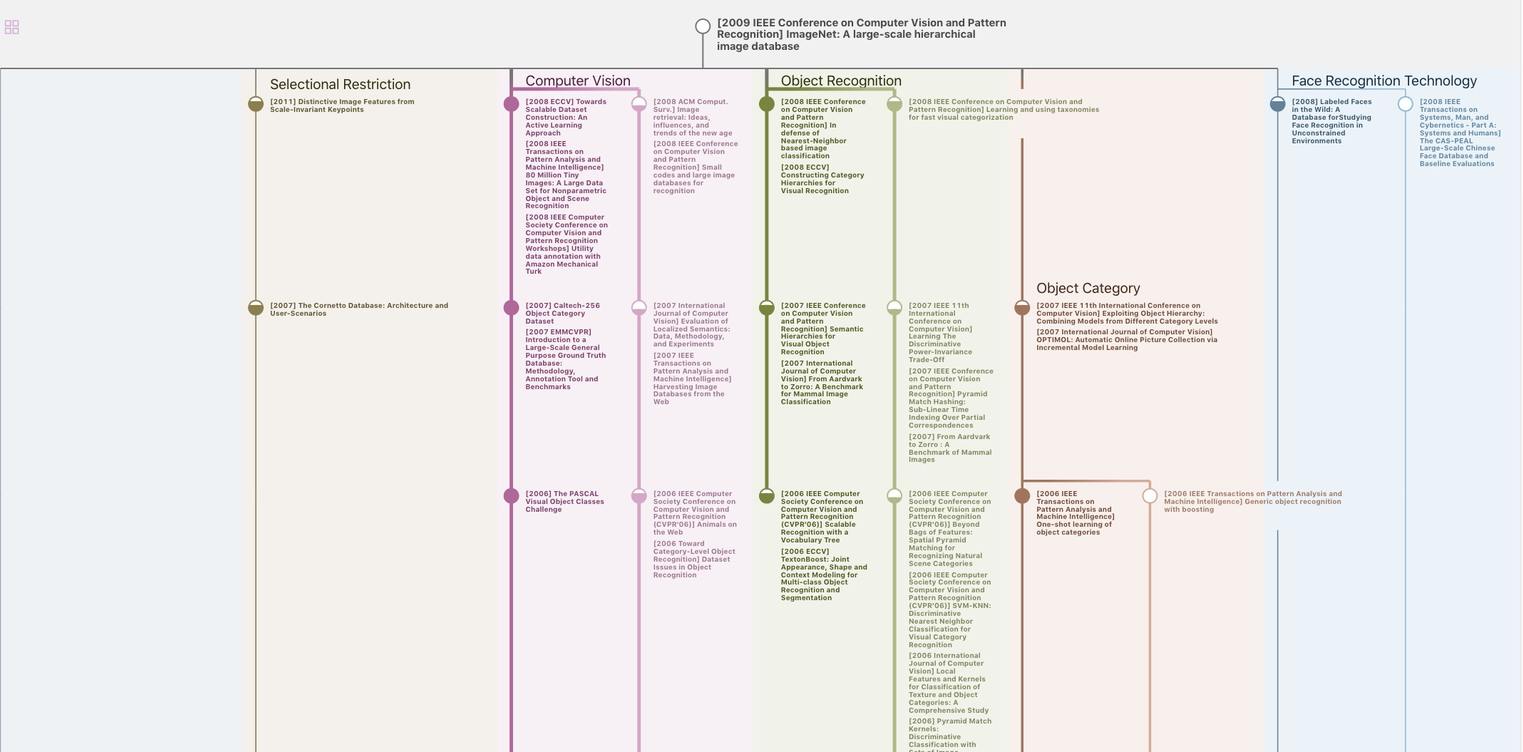
生成溯源树,研究论文发展脉络
Chat Paper
正在生成论文摘要