Motivating Bilevel Approaches To Filter Learning - A Case Study.
ICIP(2021)
摘要
The recent trend in regularization methods for inverse problems is to replace handcrafted sparsifying operators with data-driven approaches. Although using such machine learning techniques often improves image reconstruction methods, the results can depend significantly on the learning methodology. This paper compares two supervised learning methods. First, the paper considers a transform learning approach and, to learn the transform, introduces a variant on the Procrustes method for wide matrices with orthogonal rows. Second, we consider a bilevel convolutional filter learning approach. Numerical experiments show the learned transform performs worse for denoising than both the handcrafted finite difference transform and the learned filters, which perform similarly. Our results motivate the use of bilevel learning.
更多查看译文
关键词
bilevel,co-sparse,denoising
AI 理解论文
溯源树
样例
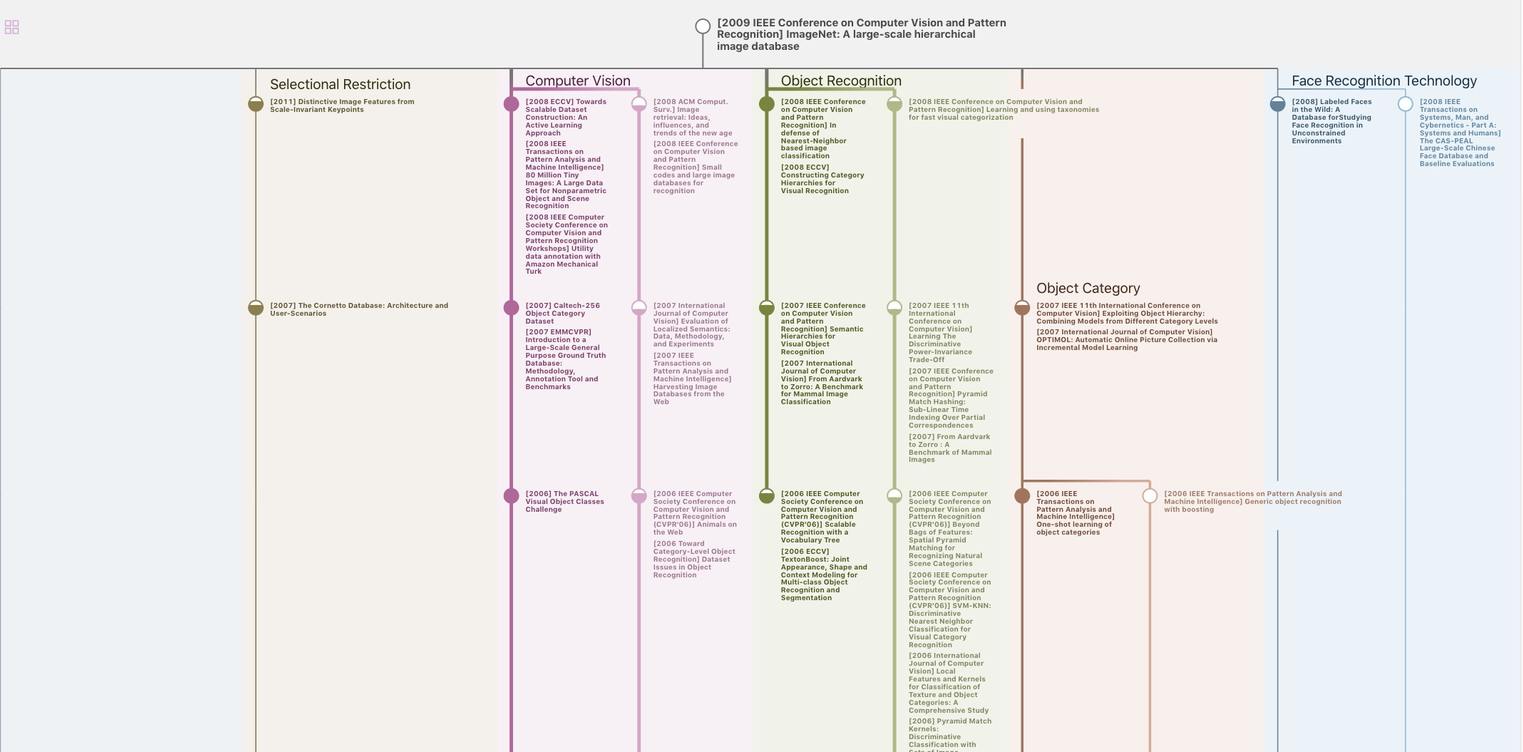
生成溯源树,研究论文发展脉络
Chat Paper
正在生成论文摘要