Multiclass histogram-based thresholding using kernel density estimation and scale-space representations
arXiv (Cornell University)(2022)
摘要
We present a new method for multiclass thresholding of a histogram which is based on the nonparametric Kernel Density (KD) estimation, where the unknown parameters of the KD estimate are defined using the Expectation-Maximization (EM) iterations. The method compares the number of extracted minima of the KD estimate with the number of the requested clusters minus one. If these numbers match, the algorithm returns positions of the minima as the threshold values, otherwise, the method gradually decreases/increases the kernel bandwidth until the numbers match. We verify the method using synthetic histograms with known threshold values and using the histogram of real X-ray computed tomography images. After thresholding of the real histogram, we estimated the porosity of the sample and compare it with the direct experimental measurements. The comparison shows the meaningfulness of the thresholding.
更多查看译文
关键词
thresholding,kernel density estimation
AI 理解论文
溯源树
样例
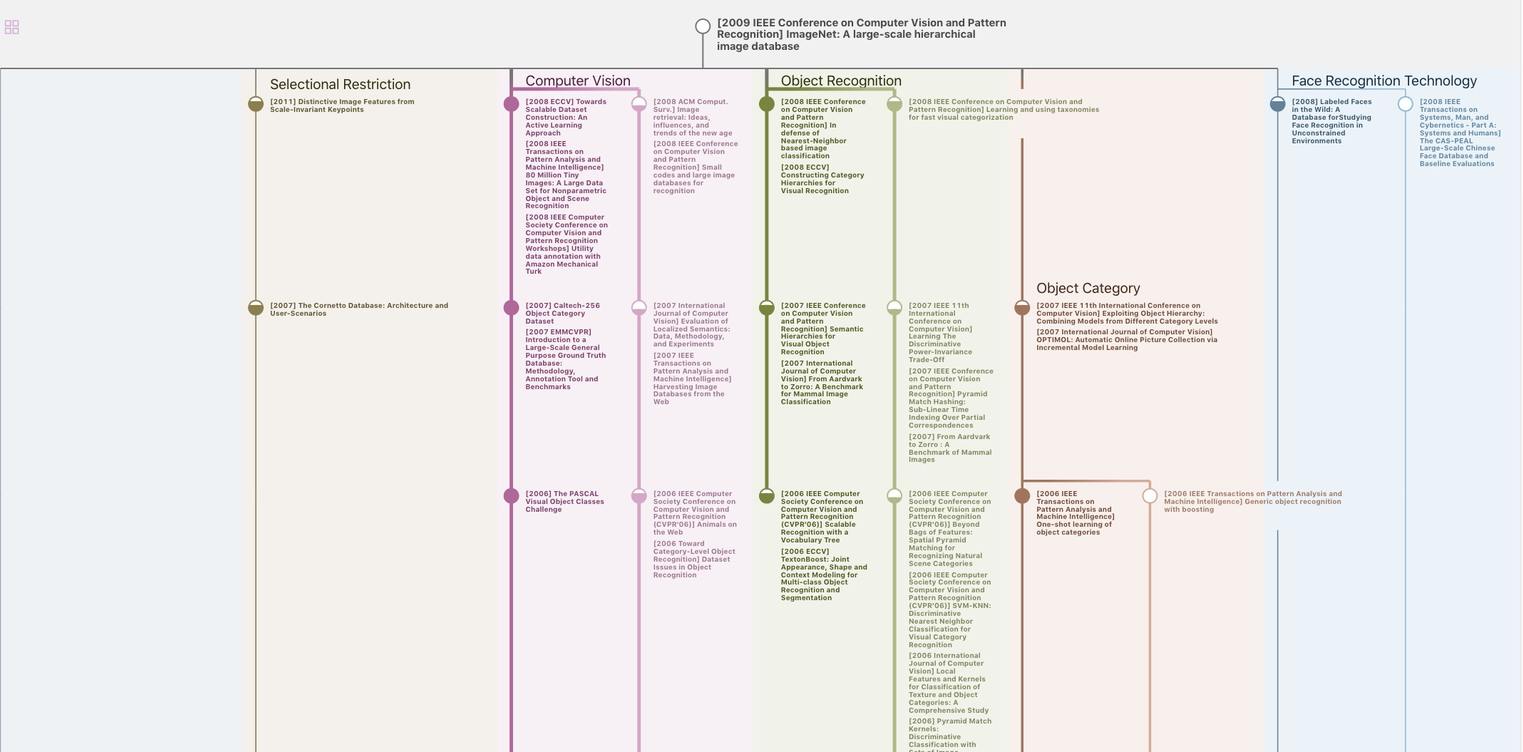
生成溯源树,研究论文发展脉络
Chat Paper
正在生成论文摘要