An Ensemble of Transformer and LSTM Approach for Multivariate Time Series Data Classification.
IEEE BigData(2021)
摘要
Wafer manufacturing is a complex and time taking process. The multivariate time-series data collected from many soft sensors in the process are highly noisy and imbalanced. Thus, wafer classification is a challenging task. To overcome this challenge, we propose an effective ensemble approach with transformer and long short term memory (LSTM) based deep learning techniques for wafer classification. Though deep learning is a promising technique to analyze the data and make effective predictions, but not widely integrated in manufacture industries for soft sensing due to insufficient research. Also the research community has not been exposed to accessing the real and large scale wafer data that is highly noisy and imbalanced. Our proposed approach is an ensemble of four models, namely, multilayer LSTM, multilayer perceptron classifier, transformer, and feed forward neural network. We finally ensemble all of these models using ROC-AUC scores by adjusting the weights based on skewness of the models to obtain effective performance. We perform an exhaustive empirical analysis of the proposed approach and obtain a best ROC score of 0.748 that is significantly better compared to the baseline models.
更多查看译文
关键词
LSTM approach,multivariate time series data classification,wafer manufacturing,multivariate time-series data,soft sensors,wafer classification,long short term memory,deep learning techniques,manufacture industries,soft sensing,multilayer LSTM,multilayer perceptron classifier,transformer approach
AI 理解论文
溯源树
样例
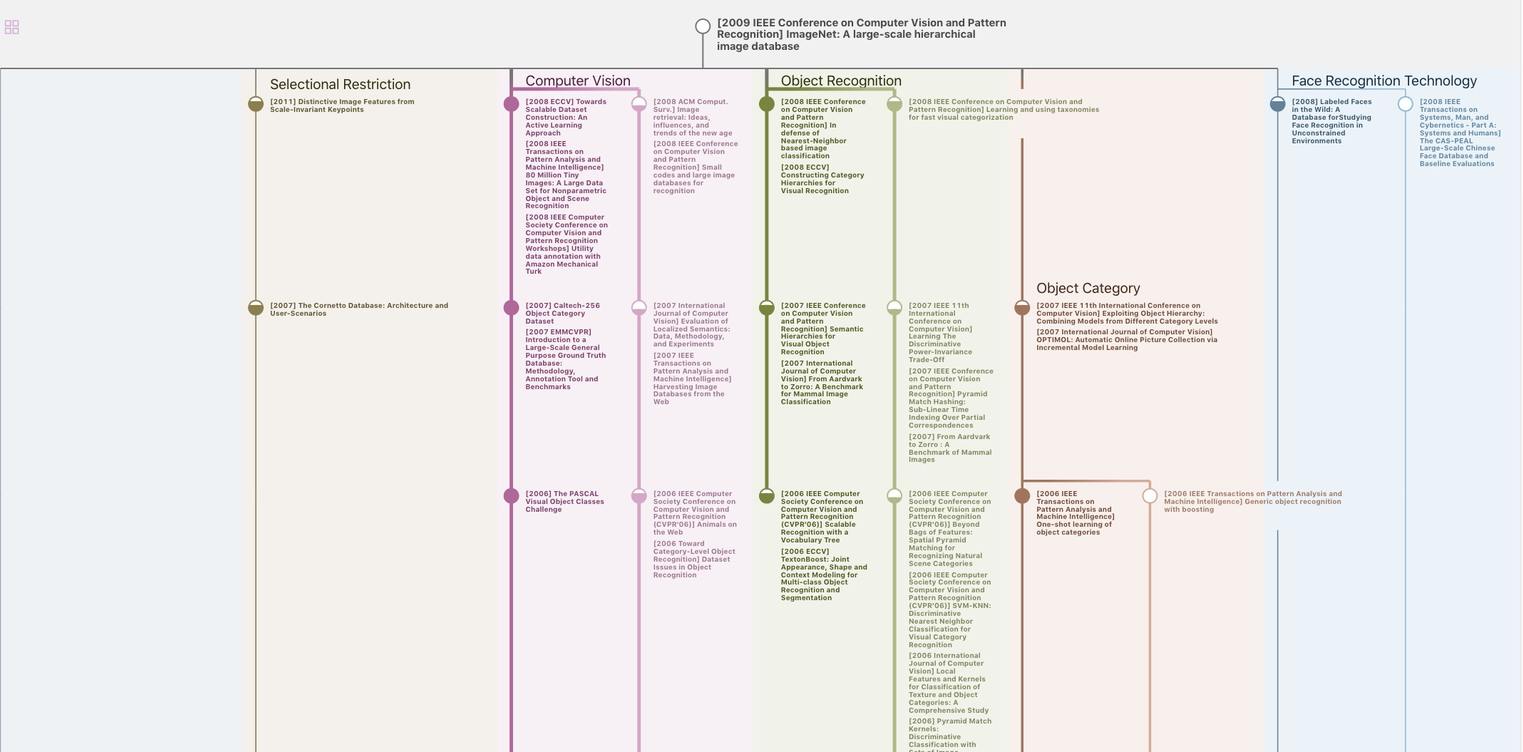
生成溯源树,研究论文发展脉络
Chat Paper
正在生成论文摘要