Enhancing and Dissecting Crowd Counting by Synthetic Data
IEEE International Conference on Acoustics, Speech, and Signal Processing (ICASSP)(2022)
Abstract
In this article, we propose a simulated crowd counting dataset CrowdX, which has a large scale, accurate labeling, parameterized realization, and high fidelity. The experimental results of using this dataset as data enhancement show that the performance of the proposed streamlined and efficient benchmark network ESA-Net can be improved by 8.4\%. The other two classic heterogeneous architectures MCNN and CSRNet pre-trained on CrowdX also show significant performance improvements. Considering many influencing factors determine performance, such as background, camera angle, human density, and resolution. Although these factors are important, there is still a lack of research on how they affect crowd counting. Thanks to the CrowdX dataset with rich annotation information, we conduct a large number of data-driven comparative experiments to analyze these factors. Our research provides a reference for a deeper understanding of the crowd counting problem and puts forward some useful suggestions in the actual deployment of the algorithm.
MoreTranslated text
Key words
Crowd Counting,Crowd Density Estimation,Synthetic Data,Domain Transfer
AI Read Science
Must-Reading Tree
Example
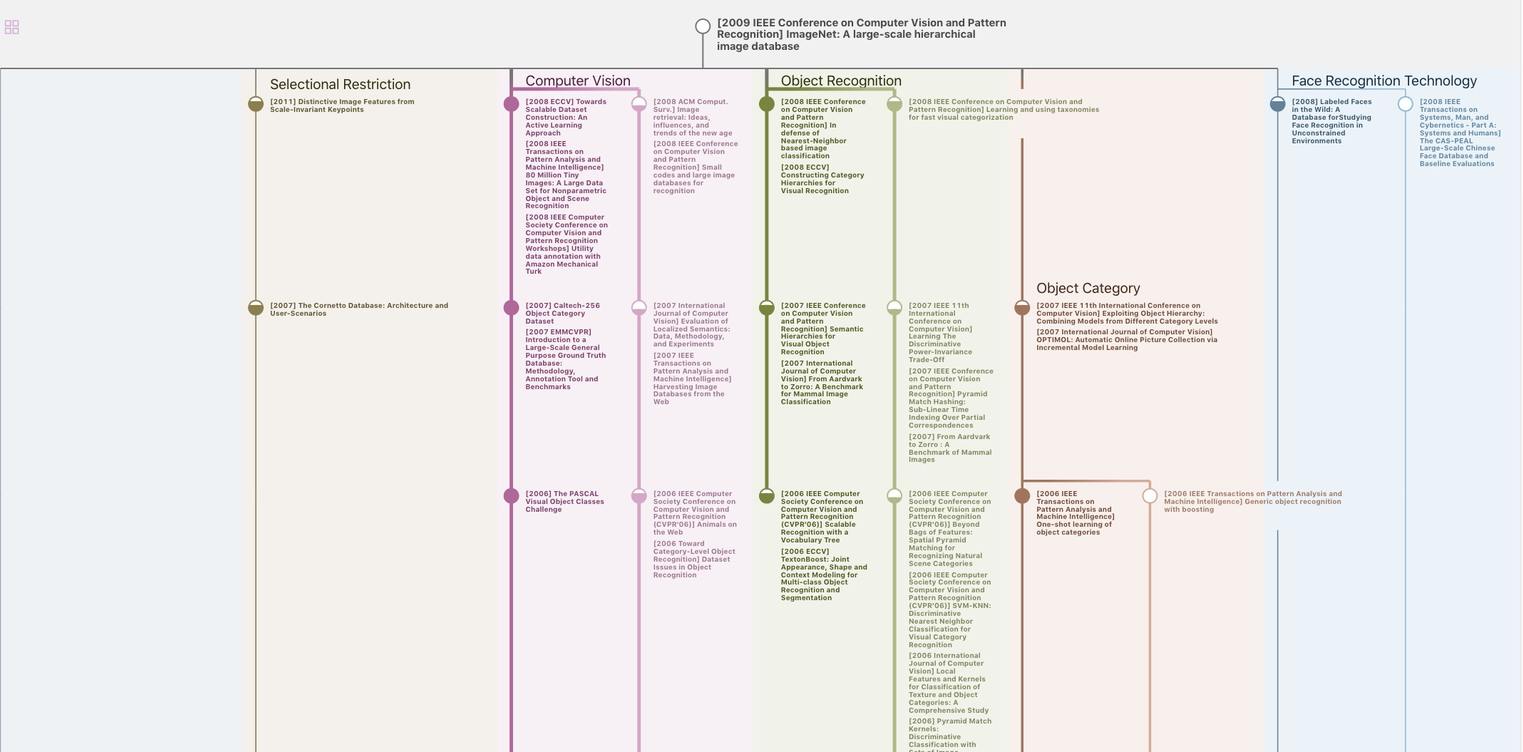
Generate MRT to find the research sequence of this paper
Chat Paper
Summary is being generated by the instructions you defined