Probing Dynamic Environments with Informed Policy Regularization
semanticscholar(2020)
摘要
We study the problem of learning explorationexploitation strategies that effectively adapt to dynamic environments, where the task may change over time. While RNN-based policies could represent such strategies, in practice their training time is prohibitive and the learning process often converges to poor solutions. In this paper, we consider the case where the agent has access to a description of the task (e.g., a task id or task parameters) at training time. We propose a novel algorithm that regularizes the training of an RNN-based policy using informed policies trained to maximize the reward in each task, dramatically improving the sample efficiency of RNN-based policies, without losing their representational power. We evaluate our algorithm in a variety of environments where tasks may vary within each episode.
更多查看译文
AI 理解论文
溯源树
样例
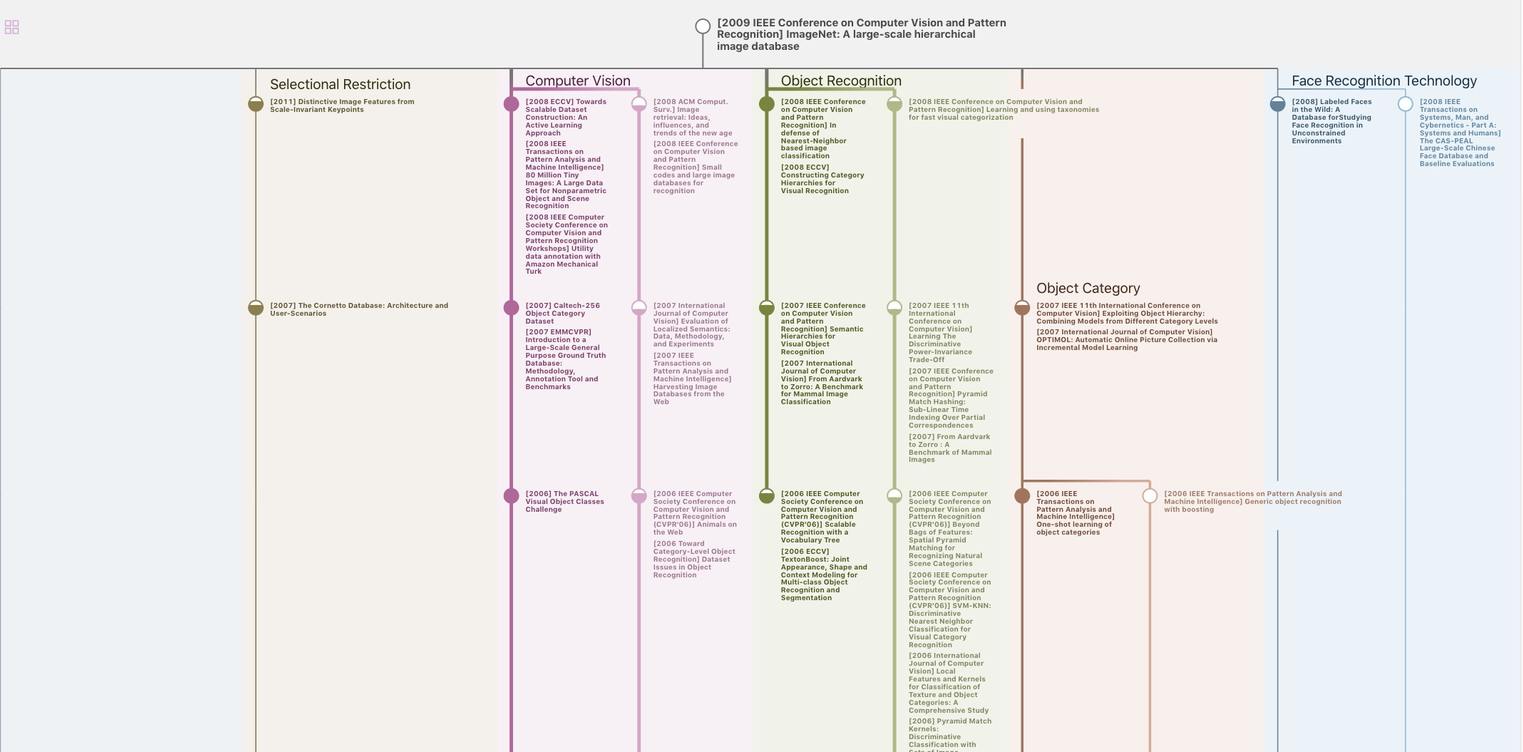
生成溯源树,研究论文发展脉络
Chat Paper
正在生成论文摘要