Machine learning algorithm to predict delirium from emergency department data
medRxiv(2021)
Abstract
Abstract Introduction: Delirium is a cerebral dysfunction seen commonly in the acute care setting. Delirium is associated with increased mortality and morbidity and is frequently missed in the emergency department (ED) by clinical gestalt alone. Identifying those at risk of delirium may help prioritize screening and interventions. Objective: Our objective was to identify clinically valuable predictive models for prevalent delirium within the first 24 hours of hospitalization based on the available data by assessing the performance of logistic regression and a variety of machine learning models. Methods: This was a retrospective cohort study to develop and validate a predictive risk model to detect delirium using patient data obtained around an ED encounter. Data from electronic health records for patients hospitalized from the ED between January 1, 2014, and December 31, 2019, were extracted. Eligible patients were aged 65 or older, admitted to an inpatient unit from the emergency department, and had at least one DOSS assessment or CAM-ICU recorded while hospitalized. The outcome measure of this study was delirium within one day of hospitalization determined by a positive DOSS or CAM assessment. We developed the model with and without the Barthel index for activity of daily living, since this was measured after hospital admission. Results: The area under the ROC curves for delirium ranged from .69 to .77 without the Barthel index. Random forest and gradient-boosted machine showed the highest AUC of .77. At the 90% sensitivity threshold, gradient-boosted machine, random forest, and logistic regression achieved a specificity of 35%. After the Barthel index was included, random forest, gradient-boosted machine, and logistic regression models demonstrated the best predictive ability with respective AUCs of .85 to .86. Conclusion: This study demonstrated the use of machine learning algorithms to identify the combination of variables that are predictive of delirium within 24 hours of hospitalization from the ED.
MoreTranslated text
AI Read Science
Must-Reading Tree
Example
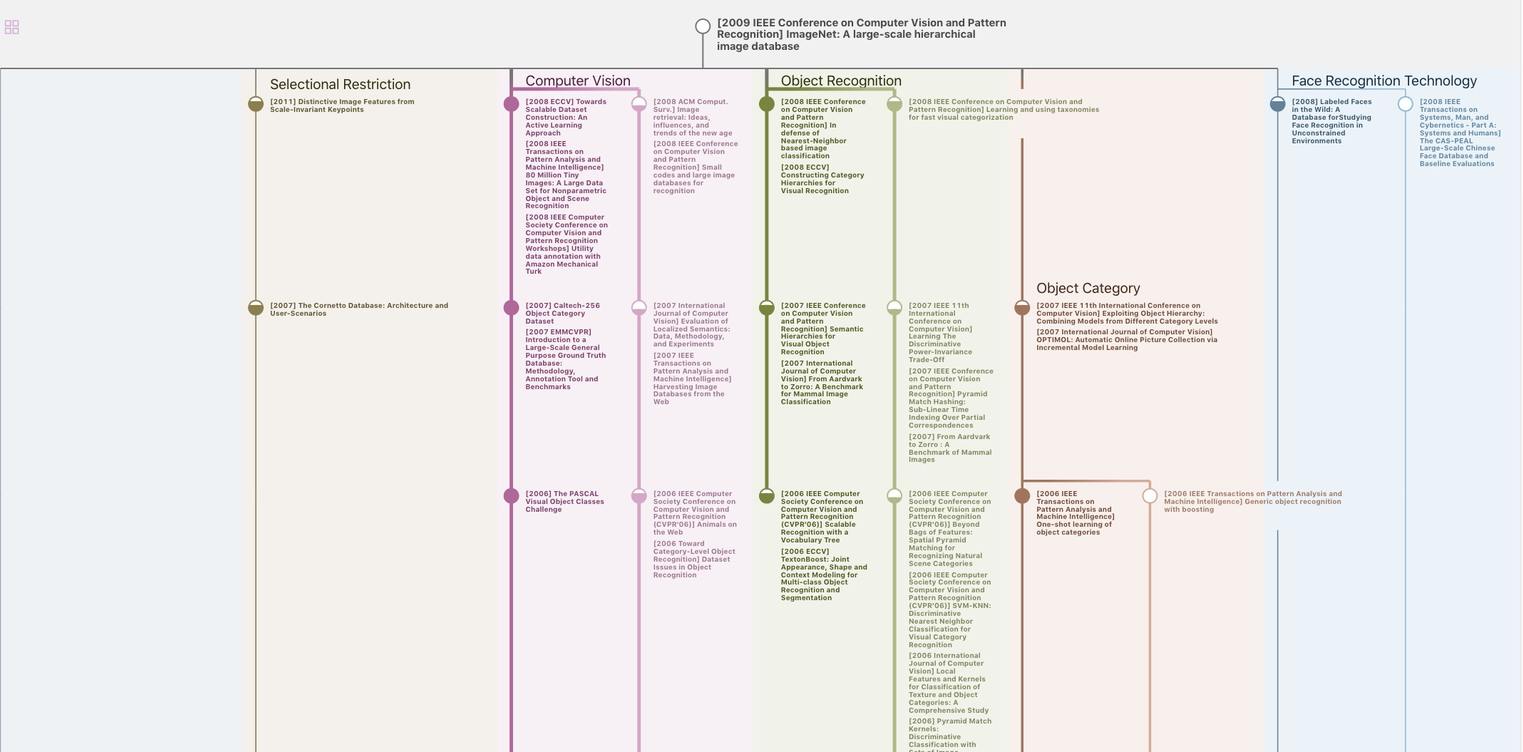
Generate MRT to find the research sequence of this paper
Chat Paper
Summary is being generated by the instructions you defined