Distributed Algorithms and Inverse Graph Filtering
Sampling Theory, Signal Processing, and Data Analysis(2020)
摘要
Graph signal processing provides an innovative framework to handle data residing on distributed networks, smart grids, neural networks, social networks and many other irregular domains. By leveraging applied harmonic analysis and graph spectral theory, graph signal processing has been extensively exploited, and many important concepts in classical signal processing have been extended to the graph setting such as graph Fourier transform, graph wavelets and graph filter banks. Similarly, many optimization problems in machine learning, sensor networks, power systems, control theory and signal processing can be modeled using underlying network structure. In modern applications, the size of a network is large, and amount of data needed to store and analyze is massive. Due to privacy and security concern, storage limitations and communication cost, a traditional centralized optimization methods are not suitable to solve these optimization problems, and distributed optimization methods are desirable. Graph filters and their inverses have been widely used in denoising, smoothing, sampling, interpolating and learning. Implementation of an inverse filtering procedure on spatially distributed networks (SDNs) is a remarkable challenge, as each agent on an SDN is equipped with a data processing subsystem with limited capacity and a communication subsystem with confined range due to engineering limitations. In this dissertation, we implement the filtering procedure associated with a polynomial graph filter of multiple shifts at the vertex level in a distributed network, where each vertex is equipped with a data processing subsystem for limited computation power and data storage, and a communication subsystem for direct data exchange to its adjacent vertices. We also consider the implementation of inverse filtering procedure associated with a polynomial graph filter of multiple shifts, and we propose two iterative approximation algorithms applicable in a distributed network and in a central
更多查看译文
AI 理解论文
溯源树
样例
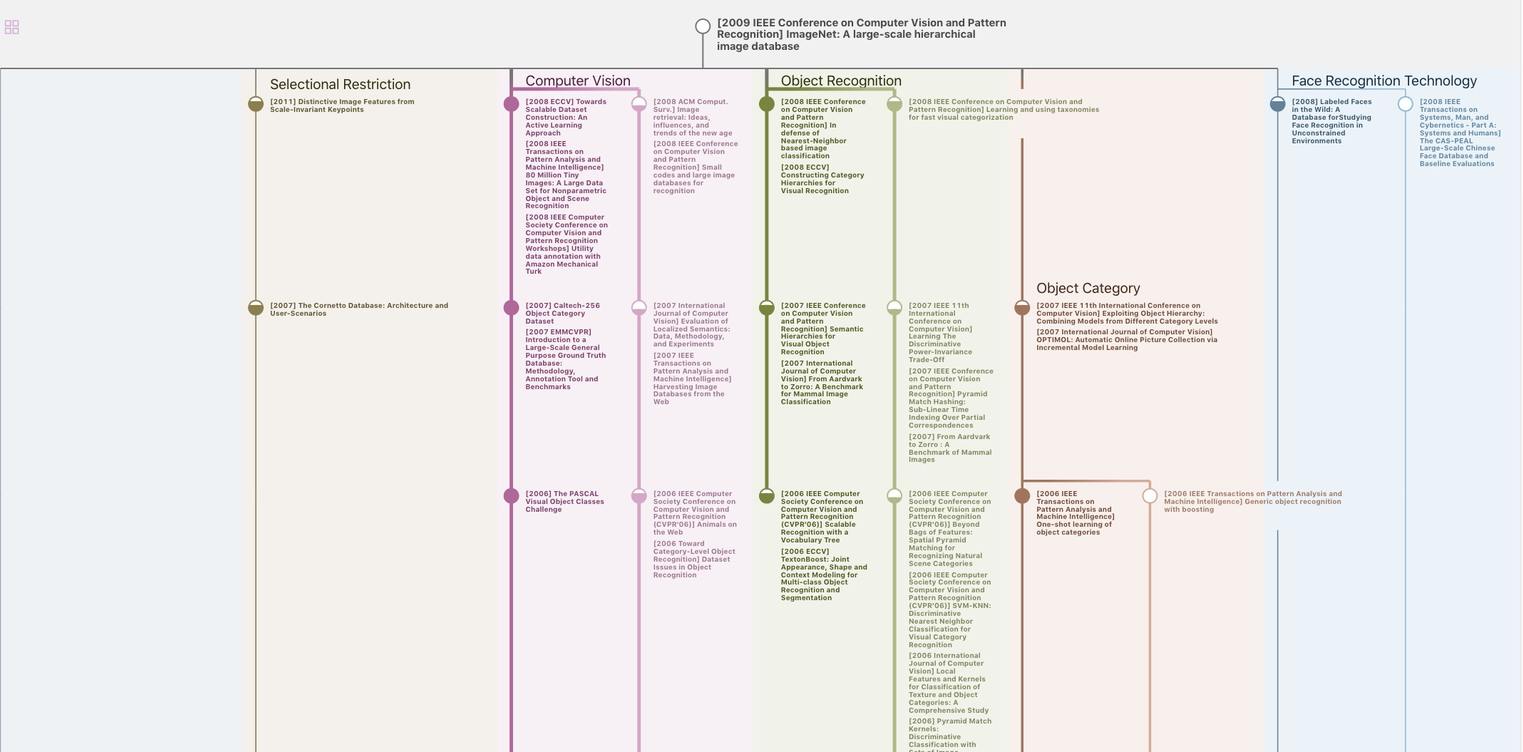
生成溯源树,研究论文发展脉络
Chat Paper
正在生成论文摘要