Establishment of prediction models for lung cancer NOG/PDX models: A guideline for machine learning in small biomedical datasets
Research Square (Research Square)(2020)
Abstract
Abstract Background: Targeted therapy and immune checkpoint inhibitors are the most promising treatments for lung cancers but still facing multiple challenges, including resistance and individual difference. Therefore, patient-derived tumor xenografts (PDX) models are developed for drug discovery and screening. NOG mice is under the destruction of the interleukin-2 (IL-2) receptor common gamma chain, which is appropriate for building PDX models to test immunotherapies. However, current studies have little understanding of the causes of genotype mismatches in PDX or NOG/PDX models, which leads to a massive economic and time loss.Methods: Lung cancer tissues from 53 patients were obtained and engrafted into NOG mice. All of the patients' tumors and NOG/PDX models were detected for common gene mutations. Seventeen clinicopathological features were organized and input to stepwise logistic regression based on the lowest Akaike information criterion (AIC), least absolute shrinkage and selection operator (LASSO)-logistic regression, support vector machine recursive feature elimination (SVM-RFE), eXtreme Gradient Boosting (XGBoost), Gradient Boosting & Categorical Features (CatBoost), and synthetic minority over-sampling technique (SMOTE). Finally, the performance of all models was evaluated by the accuracy, area under the receiver operating characteristic curve (AUC), and F1 score in 100 testing groups.Results: Fifty-three lung cancer NOG/PDX models were successfully established, with a genotype matching rate of 79.2% (42/53). Two multivariable logistic regressions revealed that age, the number of driver mutations, epidermal growth factor receptor (EGFR) gene mutations, the type of prior chemotherapy, prior tyrosine kinase inhibitors (TKIs) therapy, and the source were potent predictors. Moreover, CatBoost (mean accuracy=0.960; mean AUC=0.939; mean F1 score=0.908) and 8-feature SVM (mean accuracy=0.950; mean AUC=0.934; mean F1 score=0.903) showed the best performance compared with the other algorithms. Moreover, the combination of SMOTE with SVM significantly improved the predictive capability (mean accuracy: 0.961 vs. 0.958, P=0.025; mean AUC: 0.940 vs. 0.935, P=0.045; mean F1 score: 0.909 vs. 0.903, P=0.047).Conclusions: We established an optimal predictive model to screen lung cancer patients for NOG/PDX models, and also offered a general approach for building prediction models in small unbalanced biomedical samples.
MoreTranslated text
Key words
lung cancer nog/pdx,prediction models,machine learning,nog/pdx models
AI Read Science
Must-Reading Tree
Example
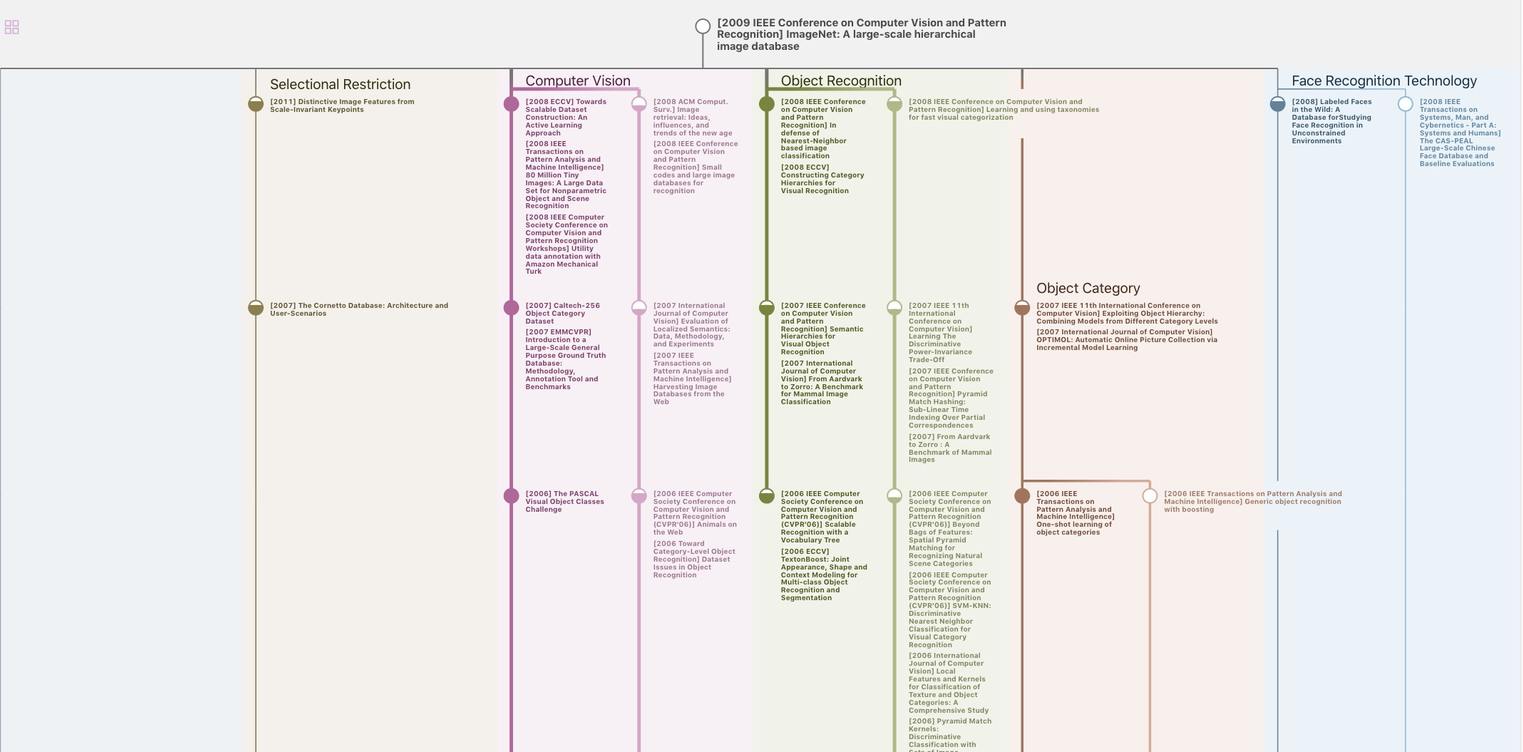
Generate MRT to find the research sequence of this paper
Chat Paper
Summary is being generated by the instructions you defined