Deep Transfer Learning Based Multi-source Heterogeneous data Fusion with Application to Cross-scenario Tool Wear monitoring
2021 7TH INTERNATIONAL CONFERENCE ON MECHANICAL ENGINEERING AND AUTOMATION (ICMEAS 2021)(2021)
Abstract
Tool wear monitoring is the key part of intelligent maintenance and has been attracting considerable interest. However, traditional data-driven methods assume that the collected data following identical distribution and the training data is sufficient, which is impractical in practice. This paper proposed a novel framework to realize tool wear monitoring across scenarios based on deep transfer learning. The collected multi-source heterogeneous signal is fused based on the feature self-extraction and selection capabilities of deep learning. Particularly, the feature extraction capability of convolutional neural networks (CNN) for structured data is improved by integrating traditional feature extraction methods. Furthermore, the transfer learning technique is introduced to migrate the pre-trained model from the source domain to the target domain, and thus achieving the tool wear monitoring across scenarios. The proposed framework was applied to the continuous cutting process of tools and the excellent experimental results demonstrated the effectiveness of the deep transfer learning network for tool wear monitoring with a small number of labeled data, which demonstrates the practicality of the proposed framework.
MoreTranslated text
Key words
tool wear monitoring,deep transfer learning,multi-source heterogeneous,data fusion
AI Read Science
Must-Reading Tree
Example
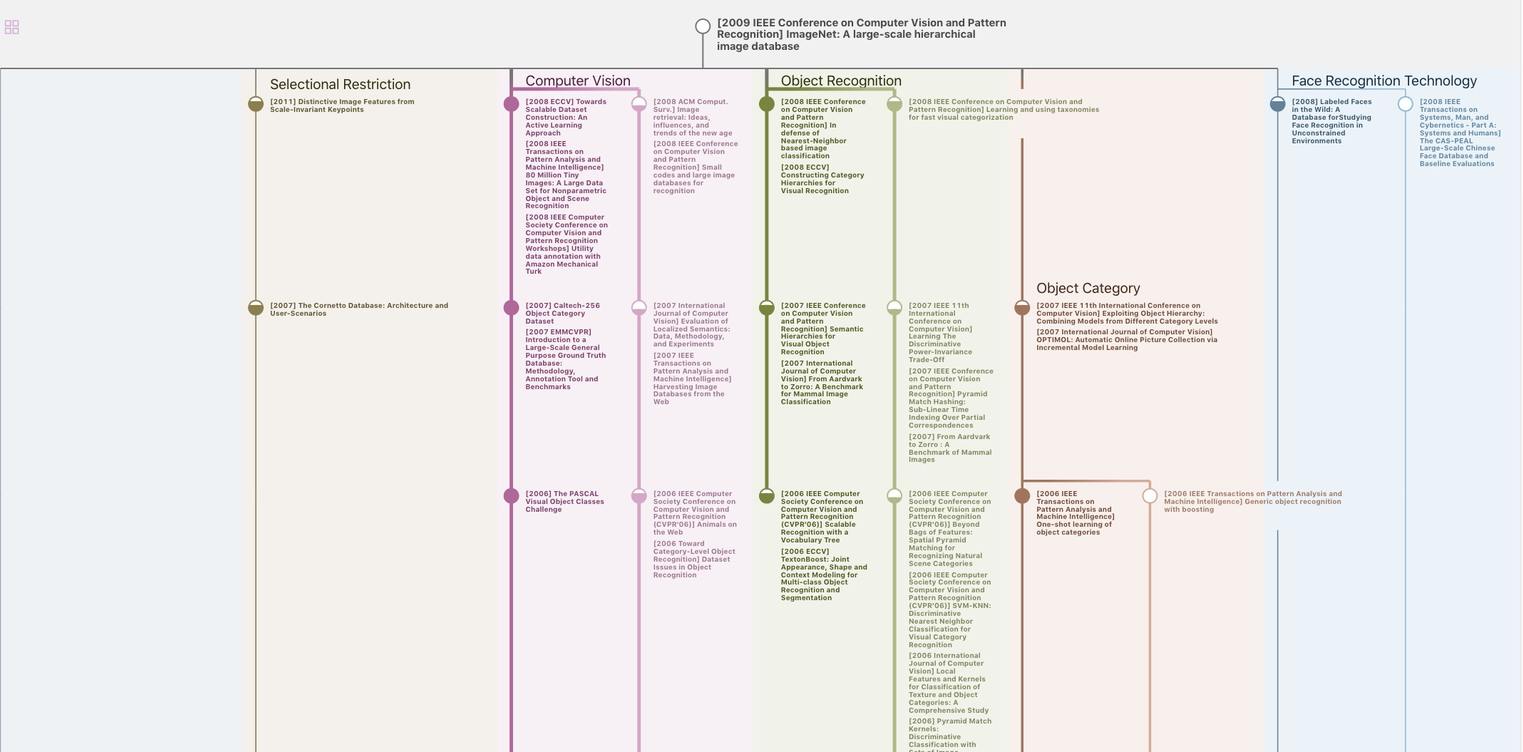
Generate MRT to find the research sequence of this paper
Chat Paper
Summary is being generated by the instructions you defined