Flexible Networks for Learning Physical Dynamics of Deformable Objects
arXiv.org(2022)
摘要
Learning the physical dynamics of deformable objects with particle-based representation has been the objective of many computational models in machine learning. While several state-of-the-art models have achieved this objective in simulated environments, most existing models impose a precondition, such that the input is a sequence of ordered point sets. That is, the order of the points in each point set must be the same across the entire input sequence. This precondition restrains the model from generalizing to real-world data, which is considered to be a sequence of unordered point sets. In this paper, we propose a model named time-wise PointNet (TP-Net) that solves this problem by directly consuming a sequence of unordered point sets to infer the future state of a deformable object with particle-based representation. Our model consists of a shared feature extractor that extracts global features from each input point set in parallel and a prediction network that aggregates and reasons on these features for future prediction. The key concept of our approach is that we use global features rather than local features to achieve invariance to input permutations and ensure the stability and scalability of our model. Experiments demonstrate that our model achieves state-of-the-art performance with real-time prediction speed in both synthetic dataset and real-world dataset. In addition, we provide quantitative and qualitative analysis on why our approach is more effective and efficient than existing approaches.
更多查看译文
AI 理解论文
溯源树
样例
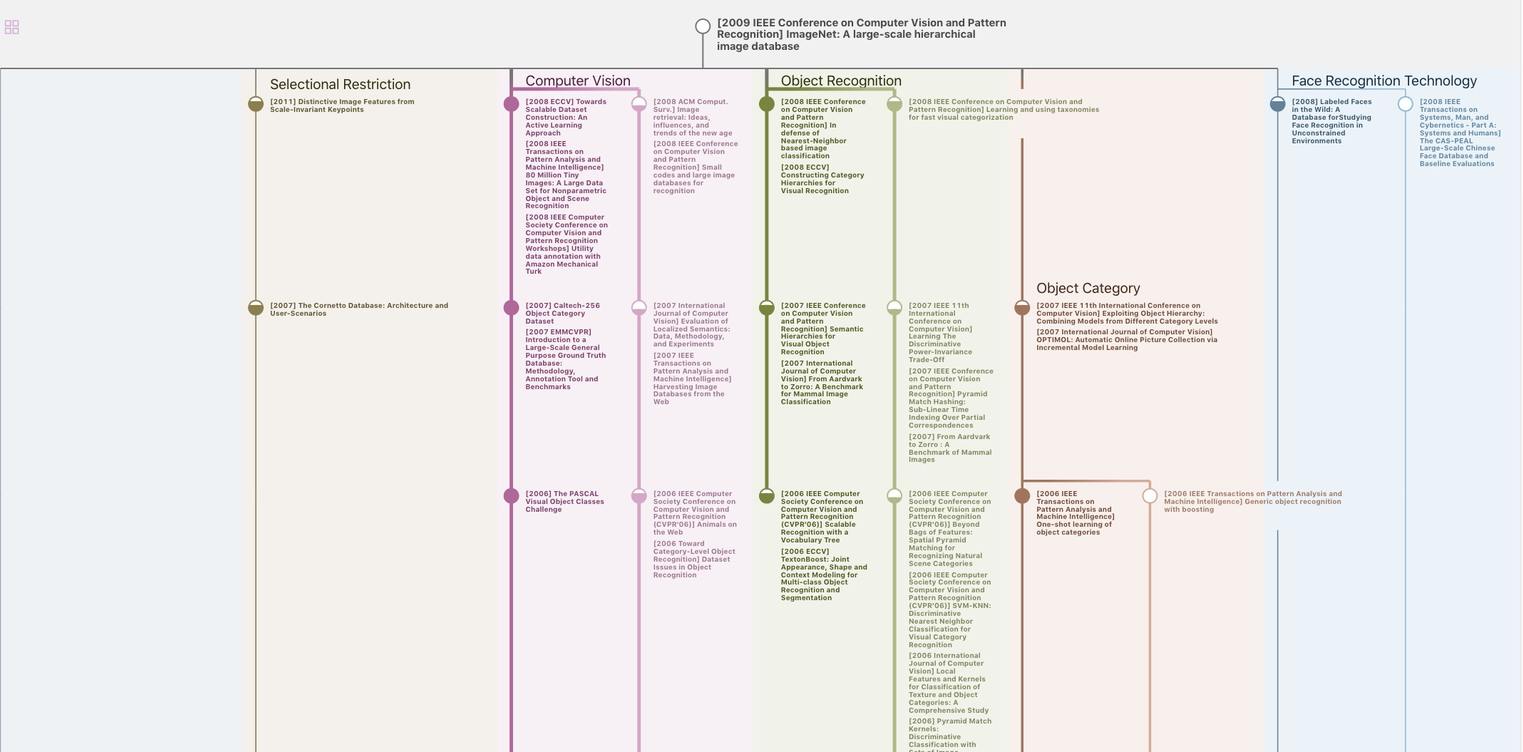
生成溯源树,研究论文发展脉络
Chat Paper
正在生成论文摘要