The Dark Energy Survey supernova program: cosmological biases from supernova photometric classification
arXiv (Cornell University)(2023)
摘要
Cosmological analyses of samples of photometrically identified type Ia supernovae (SNe Ia) depend on understanding the effects of 'contamination' from core-collapse and peculiar SN Ia events. We employ a rigorous analysis using the photometric classifier SuperNNova on state-of-the-art simulations of SN samples to determine cosmological biases due to such 'non-Ia' contamination in the Dark Energy Survey (DES) 5-yr SN sample. Depending on the non-Ia SN models used in the SuperNNova training and testing samples, contamination ranges from 0.8 to 3.5 per cent, with a classification efficiency of 97.7-99.5 percent. Using the Bayesian Estimation Applied to Multiple Species (BEAMS) framework and its extension BBC ('BEAMS with Bias Correction'), we produce a redshift-binned Hubble diagram marginalized over contamination and corrected for selection effects, and use it to constrain the dark energy equation-of-state, w. Assuming a flat universe with Gaussian Omega(M) prior of 0.311 +/- 0.010, we show that biases on w are <0.008 when using SuperNNova, with systematic uncertainties associated with contamination around 10 percent of the statistical uncertainty on w for the DES-SN sample. An alternative approach of discarding contaminants using outlier rejection techniques (e.g. Chauvenet's criterion) in place of SuperNNova leads to biases on w that are larger but still modest (0.015-0.03). Finally, we measure biases due to contamination on w(0) and w(a) (assuming a flat universe), and find these to be <0.009 in w(0) and <0.108 in w(a), 5 to 10 times smaller than the statistical uncertainties for the DES-SN sample.
更多查看译文
关键词
surveys,supernovae: general,cosmology: observations
AI 理解论文
溯源树
样例
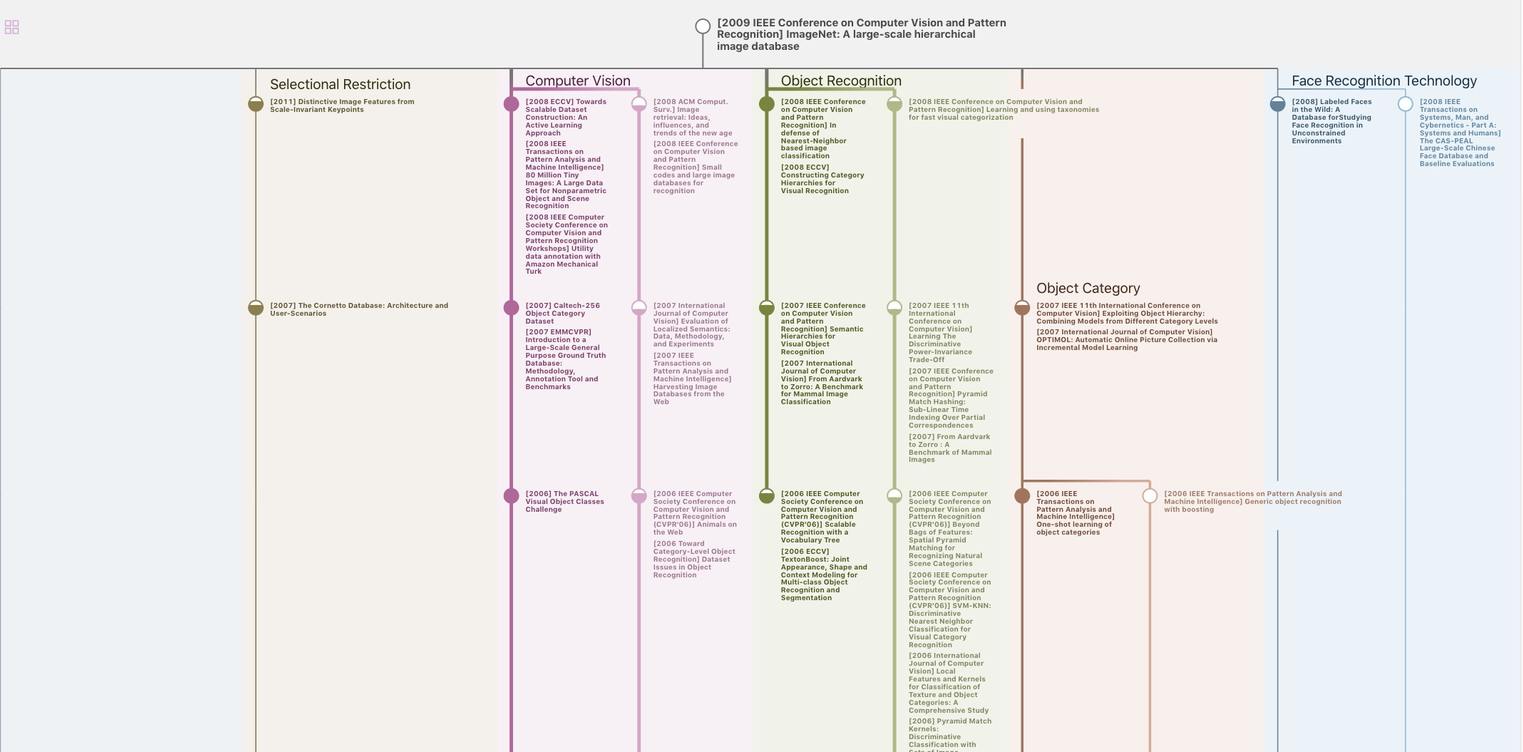
生成溯源树,研究论文发展脉络
Chat Paper
正在生成论文摘要