Multi-Space Point Geometry Compression with Progressive Relation-Aware Transformer
IEEE Transactions on Multimedia(2024)
摘要
Deep network-based point cloud geometry compression is becoming more crucial and attractive due to constantly expanding 3D applications. The current strategy employing holistic point clouds as input imposes limitations on the compressed point cloud size and results in the loss of local geometry information, simplifying the repetition of local point cloud patterns. Nevertheless, employing point cloud patches as input results in a fresh set of erroneous points within the spliced point cloud. This occurrence can be attributed to the loss of global information and the relationship among the point cloud patches. Hence, this paper introduces a novel framework for progressive downsampling point cloud compression that follows the principles of two distinct methodologies. Our strategy still uses the autoencoder structure. Specifically, the encoder reduces the point cloud size and learns features incorporating local patch structure information and global semantic information. At the same time, the decoder upsamples the quantized and entropy-encoded features to reconstruct the original point cloud. More specifically, the geometrical details inside the patch and the relationship between patches are encoded to obtain the point cloud's global semantic and local geometry information. Concurrently, the computational burden is reduced by partitioning the complete point cloud input into segments and conducting continuous downsampling. Furthermore, we introduced an attention-based point cloud deconvolution module to address the localized repeating concentrations in reconstructed point clouds resulting from linear interpolation. This module samples the parent node and its neighboring relationships in the multi-space domain to improve the characteristics of the parent nodes to be sampled. It then uses the deconvolution to create sub-node characteristics that are more varied than the linear interpolation. Empirical evidence demonstrates that the proposed methodology achieves a effective equilibrium between the compression quality and ratio.
更多查看译文
关键词
transformer,self-attention,cross-attention,point cloud geometry compression
AI 理解论文
溯源树
样例
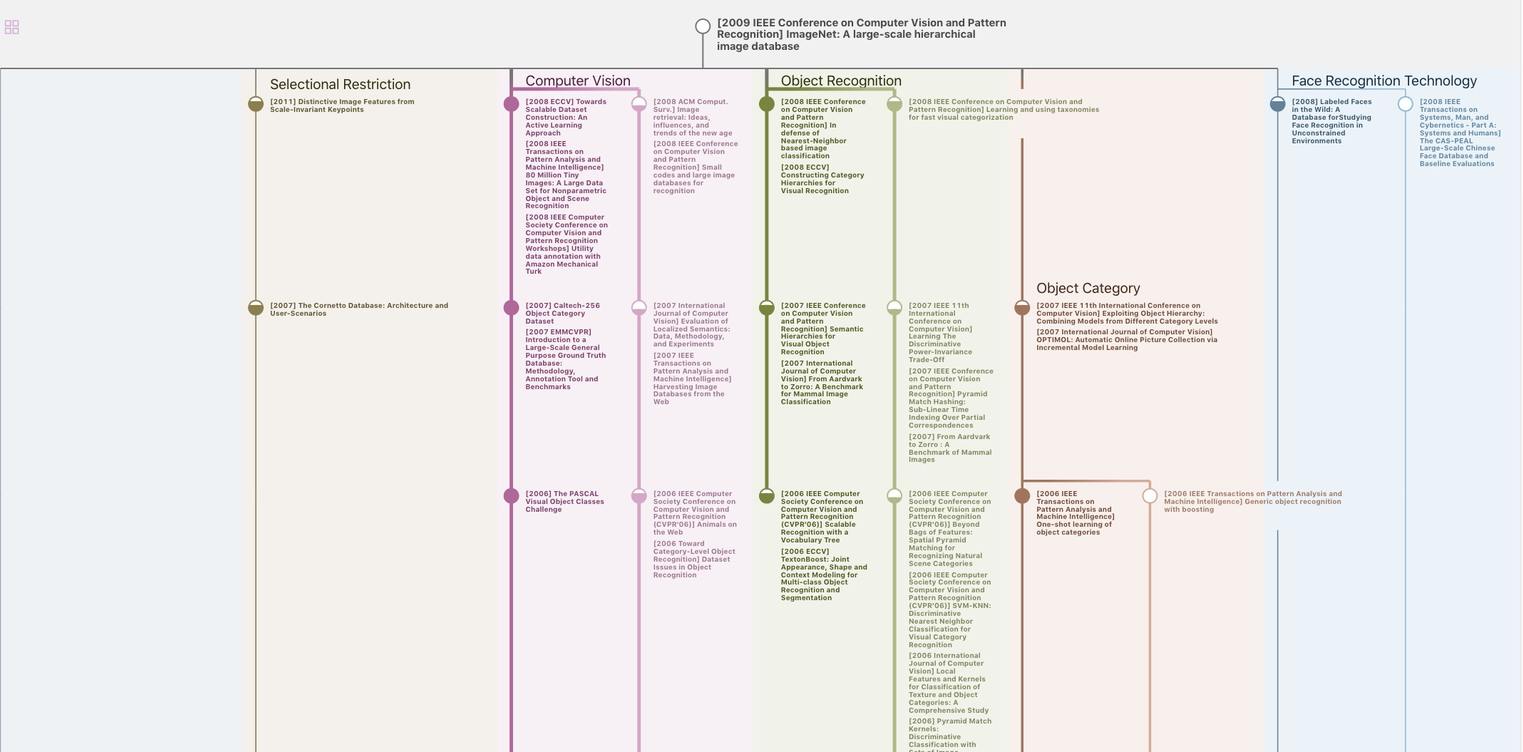
生成溯源树,研究论文发展脉络
Chat Paper
正在生成论文摘要