Wrong-way driving crash injury analysis on arterial road networks using non-parametric data mining techniques
JOURNAL OF TRANSPORTATION SAFETY & SECURITY(2022)
摘要
Wrong-way driving (WWD) crashes result in more fatalities per crash, involve more vehicles, and cause extended road closures compared to other types of crashes. Previous studies have used descriptive and parametric statistical models to identify factors that affect WWD crash severity on limited access facilities. This study adopted a combination of non-parametric data mining techniques aiming to recognize the pattern of contributing factors that affect the WWD crash severity on non-limited access facilities. These non-parametric methods can handle heterogeneity in crash datasets well. In this study, hierarchical clustering was used to divide the crash dataset into homogeneous clusters. A random forests analysis was used to select important variables, and decision trees and decision rules were generated to show the underlying pattern and interactions between different factors that affect WWD crash severity. The analysis was based on 1,475 WWD crashes that occurred on arterial streets from 2012-2016 in Florida. Results show that head-on collisions, weekend days, high-speed facilities, crashes involving vehicles entering from a driveway, dark-not lighted roadways, older drivers, and driver impairment are important factors that play a crucial role in WWD crash severity on non-limited access facilities.
更多查看译文
关键词
classification and regression trees (CART),clustering,data mining,decision rules,decision tree,machine learning,random forests,wrong-way driving
AI 理解论文
溯源树
样例
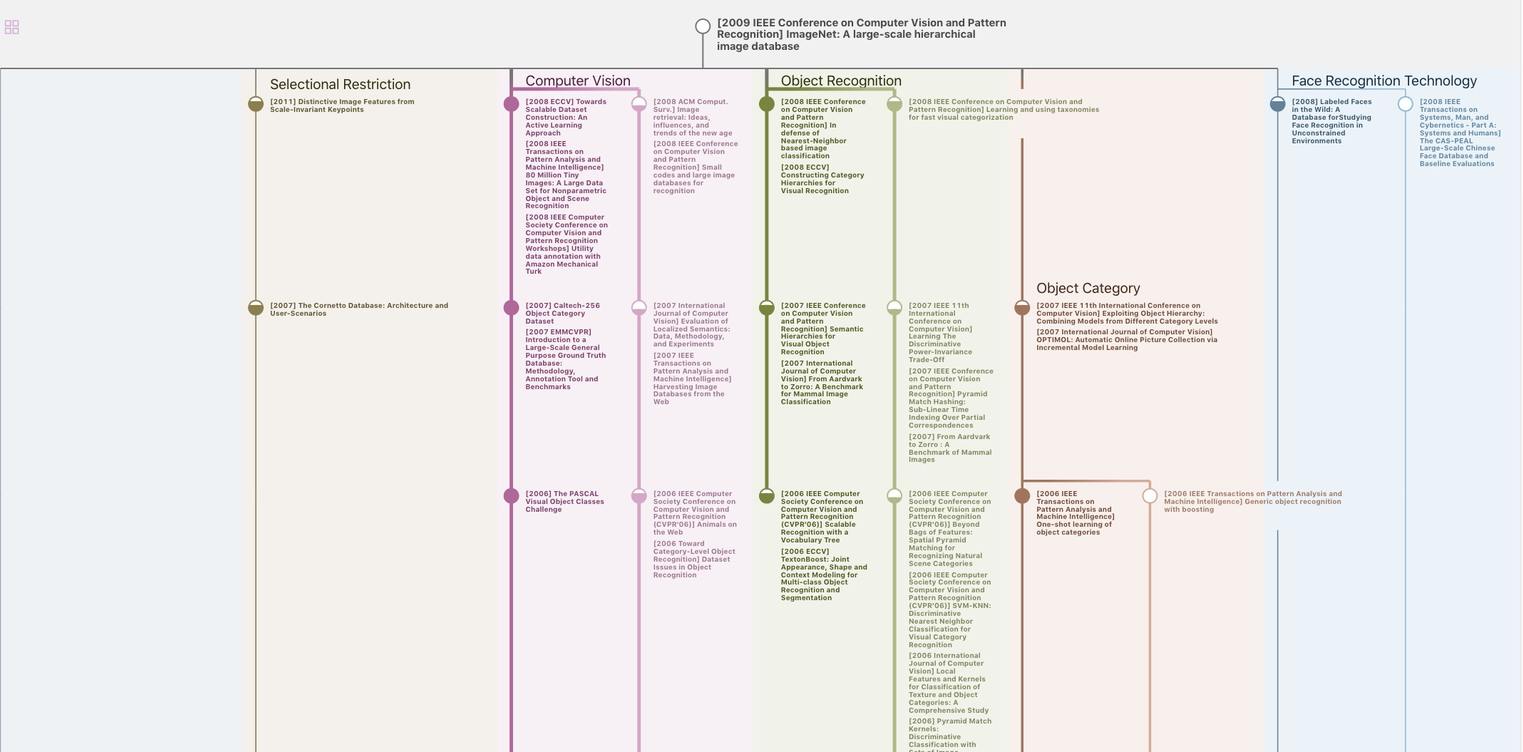
生成溯源树,研究论文发展脉络
Chat Paper
正在生成论文摘要