Hawkes-Based Models For High Frequency Financial Data
JOURNAL OF THE OPERATIONAL RESEARCH SOCIETY(2022)
摘要
Compared with low frequency data, high frequency data exhibit distinct empirical properties, including, for instance, essentially discontinuous evolution paths, time-varying intensities, and self-exciting features. All these make it more challenging to model appropriately the dynamics associated with high frequency data such as order arrival and price formation. To capture more accurately the microscopic structures and properties pertaining to the limit order books, this paper focuses on modeling high frequency data using Hawkes processes. Two models, one with exponential kernels and the other with power-law kernels, are introduced systematically, algorithmized precisely, and compared with each other extensively from various perspectives, including the goodness of fit to the original data and the computational time in searching for the maximum likelihood estimator, with search algorithm being taken into consideration as well. To measure the goodness of fit, a number of quantities are proposed. Studies based on both multiple-trading-day data of one stock and multiple-stock data on one trading day indicate that Hawkes processes with slowly-decaying kernels are able to reproduce the intensity of jumps in the price processes more accurately. The results suggest that Hawkes processes with power-law kernels and their implied long memory nature of self-excitation phenomena could, on the level of microstructure, serve as a realistic model for high frequency data.
更多查看译文
关键词
Hawkes processes, high frequency financial data, intensity kernel, maximum likelihood estimation, sample-path simulation
AI 理解论文
溯源树
样例
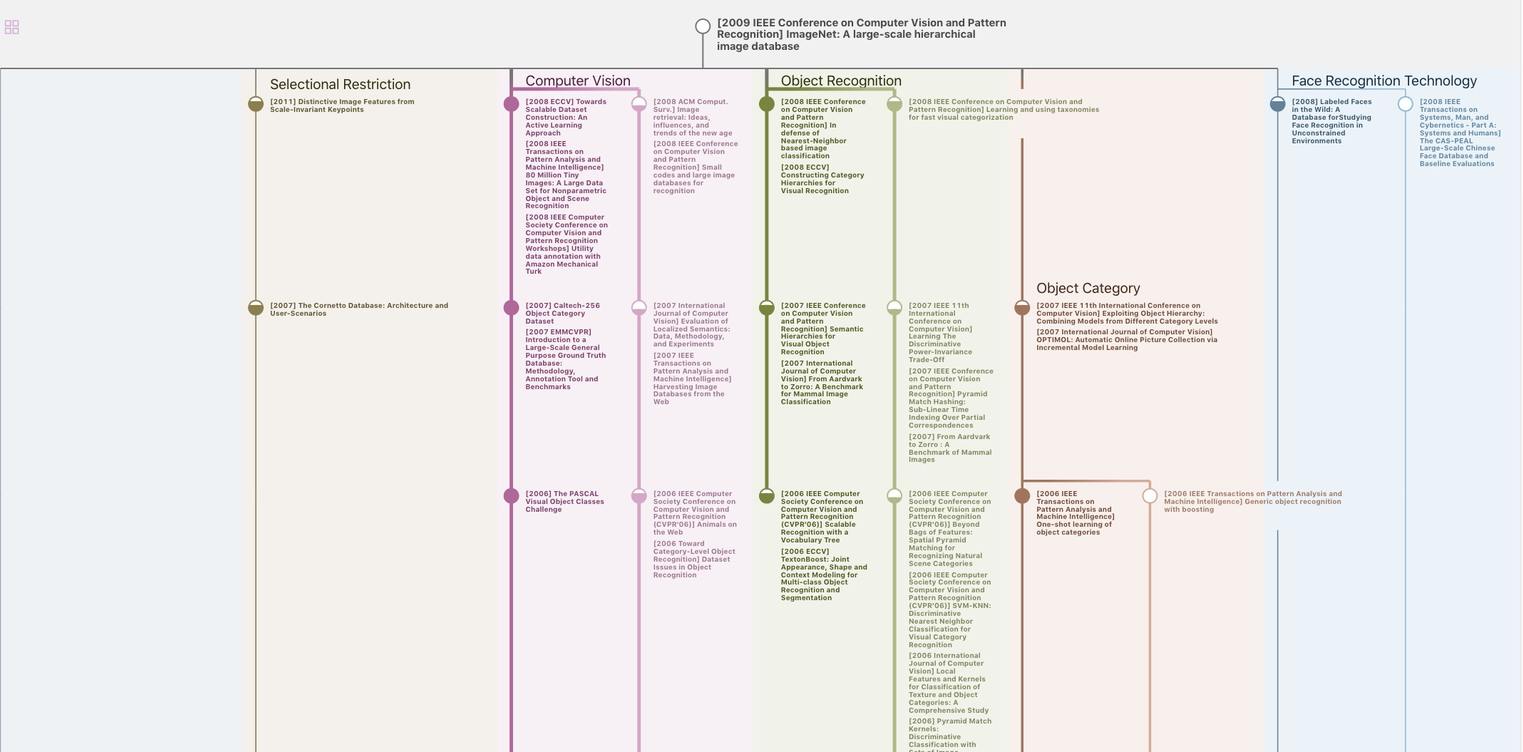
生成溯源树,研究论文发展脉络
Chat Paper
正在生成论文摘要