Approaches To Address The Data Skew Problem In Federated Learning
ARTIFICIAL INTELLIGENCE AND MACHINE LEARNING FOR MULTI-DOMAIN OPERATIONS APPLICATIONS(2019)
摘要
A Federated Learning approach consists of creating an AI model from multiple data sources, without moving large amounts of data across to a central environment. Federated learning can be very useful in a tactical coalition environment, where data can be collected individually by each of the coalition partners, but network connectivity is inadequate to move the data to a central environment. However, such data collected is often dirty and imperfect. The data can be imbalanced, and in some cases, some classes can be completely missing from some coalition partners. Under these conditions, traditional approaches for federated learning can result in models that are highly inaccurate. In this paper, we propose approaches that can result in good machine learning models even in the environments where the data may be highly skewed, and study their performance under different environments.
更多查看译文
关键词
Federated Learning,AI,Data Skew,Coalition Operations,Future Battlespace
AI 理解论文
溯源树
样例
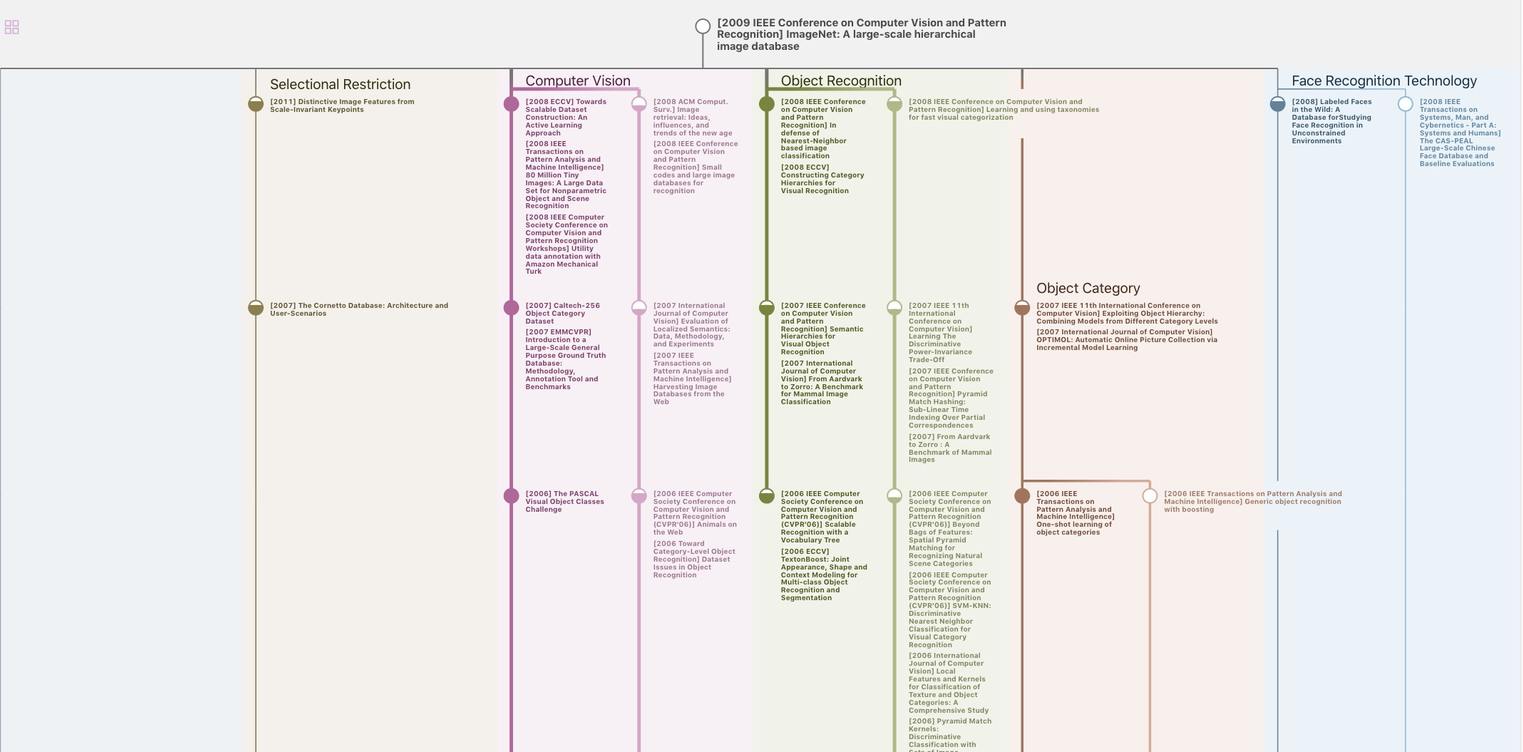
生成溯源树,研究论文发展脉络
Chat Paper
正在生成论文摘要