Revisiting Random Forests in a Comparative Evaluation of Graph Convolutional Neural Network Variants for Traffic Prediction.
ITSC(2021)
摘要
Traffic prediction is a spatiotemporal predictive task that plays an essential role in intelligent transportation systems. Today, graph convolutional neural networks (GCNNs) have become the prevailing models in the traffic prediction literature since they excel at extracting spatial correlations. In this work, we classify the components of successful GCNN prediction models and analyze the effects of matrix factorization, attention mechanism, and weight sharing on their performance. Furthermore, we compare these variations against random forests, a traditional regression method that predates GCNNs by over 15 years. We evaluated these methods using simulated data of two regions in Toronto as well as real-world sensor data from selected California highways. We found that incorporating matrix factorization, attention, and location-specific model weights either individually or collectively into GCNNs can result in a better overall performance. Moreover, although random forest regression is a less compact model, it matches or exceeds the performance of all variations of GCNNs in our experiments. This suggests that the current graph convolutional methods may not be the best approach to traffic prediction and there is still room for improvement. Finally, our findings also suggest that for future research on GCNN for traffic prediction to be credible, researchers must include performance comparison to random forests.
更多查看译文
关键词
location-specific model weights,GCNNs,random forest regression,current graph convolutional methods,random forests,graph convolutional neural network variants,spatiotemporal predictive task,graph convolutional neural networks,traffic prediction literature,successful GCNN prediction models,matrix factorization,intelligent transportation systems,time 15 year
AI 理解论文
溯源树
样例
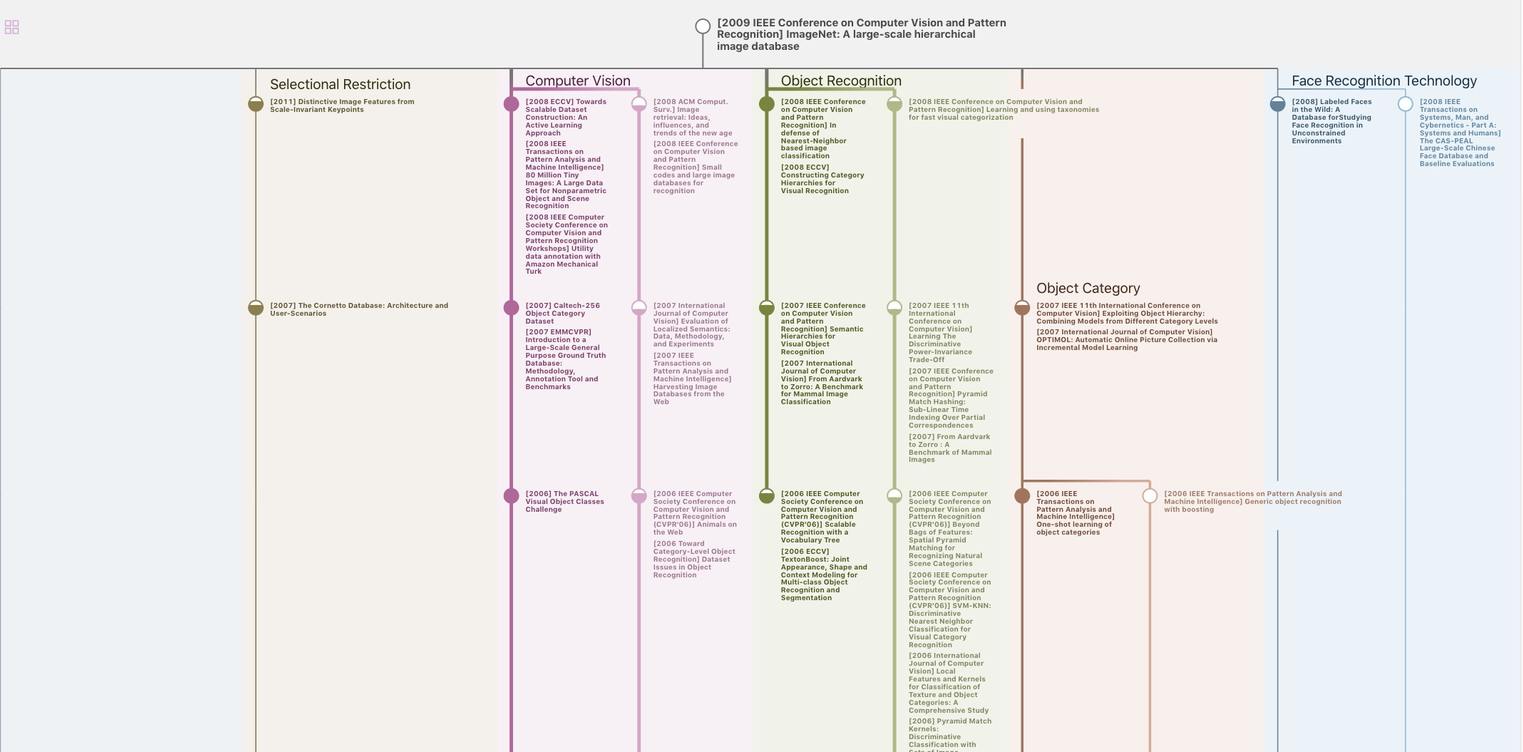
生成溯源树,研究论文发展脉络
Chat Paper
正在生成论文摘要