Doubly Robust Stein-Kernelized Monte Carlo Estimator: Simultaneous Bias-Variance Reduction and Supercanonical Convergence
JOURNAL OF MACHINE LEARNING RESEARCH(2023)
摘要
Standard Monte Carlo computation is widely known to exhibit a canonical square-root convergence speed in terms of sample size. Two recent techniques, one based on control variate and one on importance sampling, both derived from an integration of reproducing kernels and Stein's identity, have been proposed to reduce the error in Monte Carlo computation to supercanonical convergence. This paper presents a more general framework to encompass both techniques that is especially beneficial when the sample generator is biased and noise-corrupted. We show our general estimator, which we call the doubly robust Stein-kernelized estimator, outperforms both existing methods in terms of mean squared error rates across different scenarios. We also demonstrate the superior performance of our method via numerical examples.
更多查看译文
关键词
Monte Carlo methods,kernel ridge regression,Stein's identity,control functionals,importance sampling
AI 理解论文
溯源树
样例
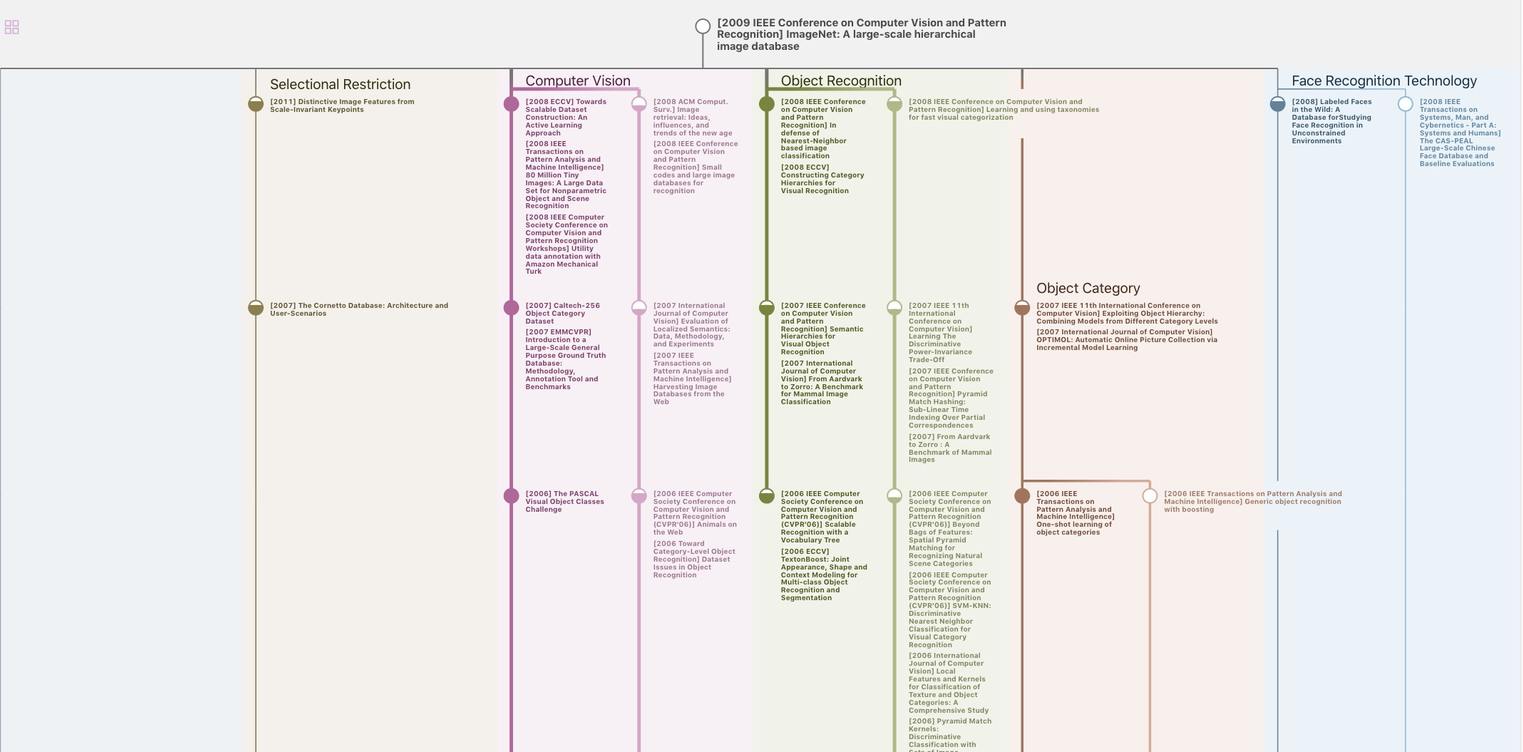
生成溯源树,研究论文发展脉络
Chat Paper
正在生成论文摘要