Multi-Source and Temporal Attention Network for Probabilistic Wind Power Prediction
IEEE Transactions on Sustainable Energy(2021)
摘要
The temporal dependencies of wind power are significant to be involved in the modeling of short-term wind power forecasts. However, different time series inputs will contribute differently to the forecasting performance and bring in challenges to the selection of the relevant driving information. In this paper, a Multi-Source and Temporal Attention Network (MSTAN) is proposed for short-term wind power probabilistic prediction. The MSTAN model introduces multi-source NWP and makes three specific designs to improve prediction performance. Firstly, a novel multi-source variable attention module is proposed to select the driving variables of NWP. Secondly, a temporal attention module is used to capture the implicit temporal dependency hidden in the historical measurements and multi-source NWP sequence. Thirdly, the residual module is wrapped in MSTAN to skip some unnecessary nonlinear transformations and provide adaptive complexity to the entire model. After training, multi-horizon density forecasts for the next 48 hours are yielded by MSTAN. The MSTAN is compared with state-of-the-art machine learning schemes in the wind power forecasting system using the operation data from 3 wind farms. We demonstrate that MSTAN outperforms other counterparts on both deterministic and probabilistic prediction. The structure design scheme of MSTAN has been proven effective.
更多查看译文
关键词
Predictive models,Wind farms,Wind power generation,Wind forecasting,Wind speed,Wind power probabilistic prediction,multi-step prediction,multi-source NWP,variable attention,attention mechanism,residual connection,mixture density
AI 理解论文
溯源树
样例
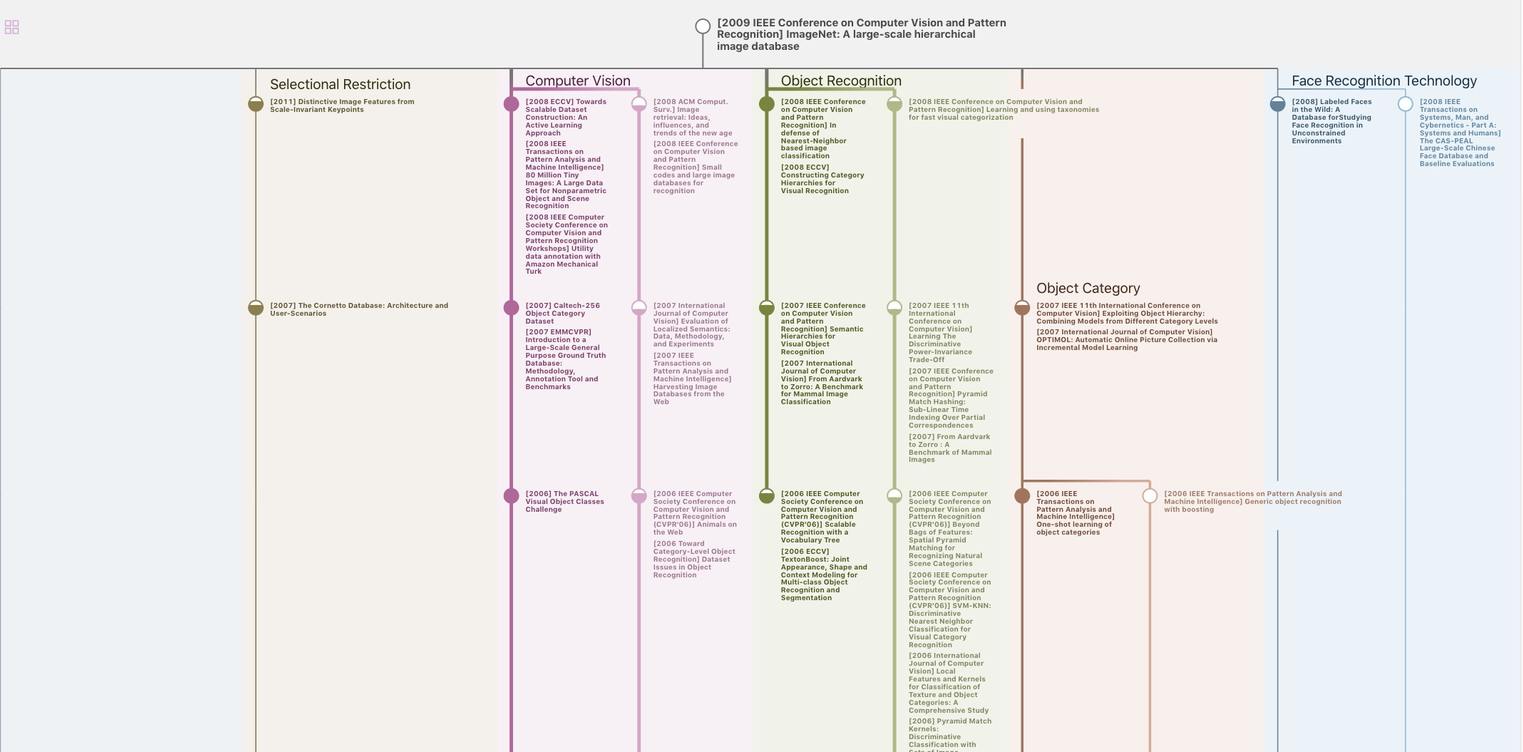
生成溯源树,研究论文发展脉络
Chat Paper
正在生成论文摘要