An Adaptive Boosting Technique to Mitigate Popularity Bias in Recommender System
CoRR(2021)
摘要
The observed ratings in most recommender systems are subjected to popularity bias and are thus not randomly missing. Due to this, only a few popular items are recommended, and a vast number of non-popular items are hardly recommended. Not suggesting the non-popular items lead to fewer products dominating the market and thus offering fewer opportunities for creativity and innovation. In the literature, several fair algorithms have been proposed which mainly focused on improving the accuracy of the recommendation system. However, a typical accuracy measure is biased towards popular items, i.e., it promotes better accuracy for popular items compared to non-popular items. This paper considers a metric that measures the popularity bias as the difference in error on popular items and non-popular items. Motivated by the fair boosting algorithm on classification, we propose an algorithm that reduces the popularity bias present in the data while maintaining accuracy within acceptable limits. The main idea of our algorithm is that it lifts the weights of the non-popular items, which are generally underrepresented in the data. With the help of comprehensive experiments on real-world datasets, we show that our proposed algorithm outperforms the existing algorithms on the proposed popularity bias metric.
更多查看译文
关键词
mitigate popularity bias,recommender system,adaptive boosting technique
AI 理解论文
溯源树
样例
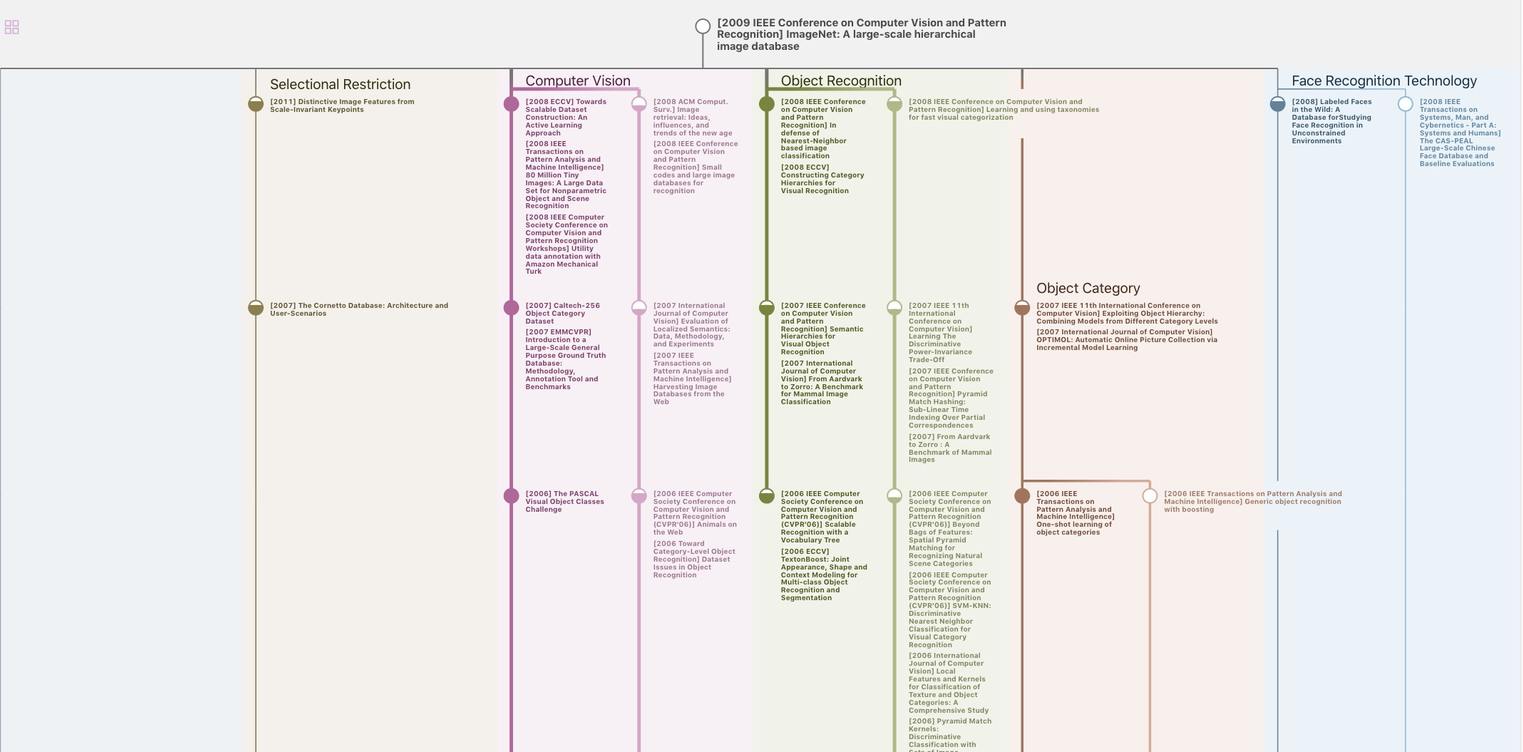
生成溯源树,研究论文发展脉络
Chat Paper
正在生成论文摘要