CheckSim: A Reference-Based Identity Document Verification by Image Similarity Measure
DOCUMENT ANALYSIS AND RECOGNITION, ICDAR 2021 WORKSHOPS, PT I(2021)
摘要
This paper presents a generic deep-learning based framework for identity document verification, which we call CheckSim. We tackle the document verification problem as a similarity measure between two aligned images: a query document image and a predefined reference representing the document model. To this end, we explore the use of two particular architectures of Convolutional Neural Network (CNN) models that are well adapted to find similarity or relationship between two comparable inputs, namely siamese and triplet CNN. The models are trained using specific loss function, then used for extracting high-dimensional features from the query and the reference images. The distance between these two feature vectors is used to decide if the two images are similar or different based on a threshold optimized by minimizing a custom cost function. An experimental comparison of these two models as well as traditional approaches based on handcrafted features is conducted on a real-world data set of patches extracted from identity documents. The obtained results show that our approach achieves good results and outperforms handcrafted features based methods.
更多查看译文
关键词
Identity document verification,Siamese/Triplet Network,Similarity measure,Forgery detection
AI 理解论文
溯源树
样例
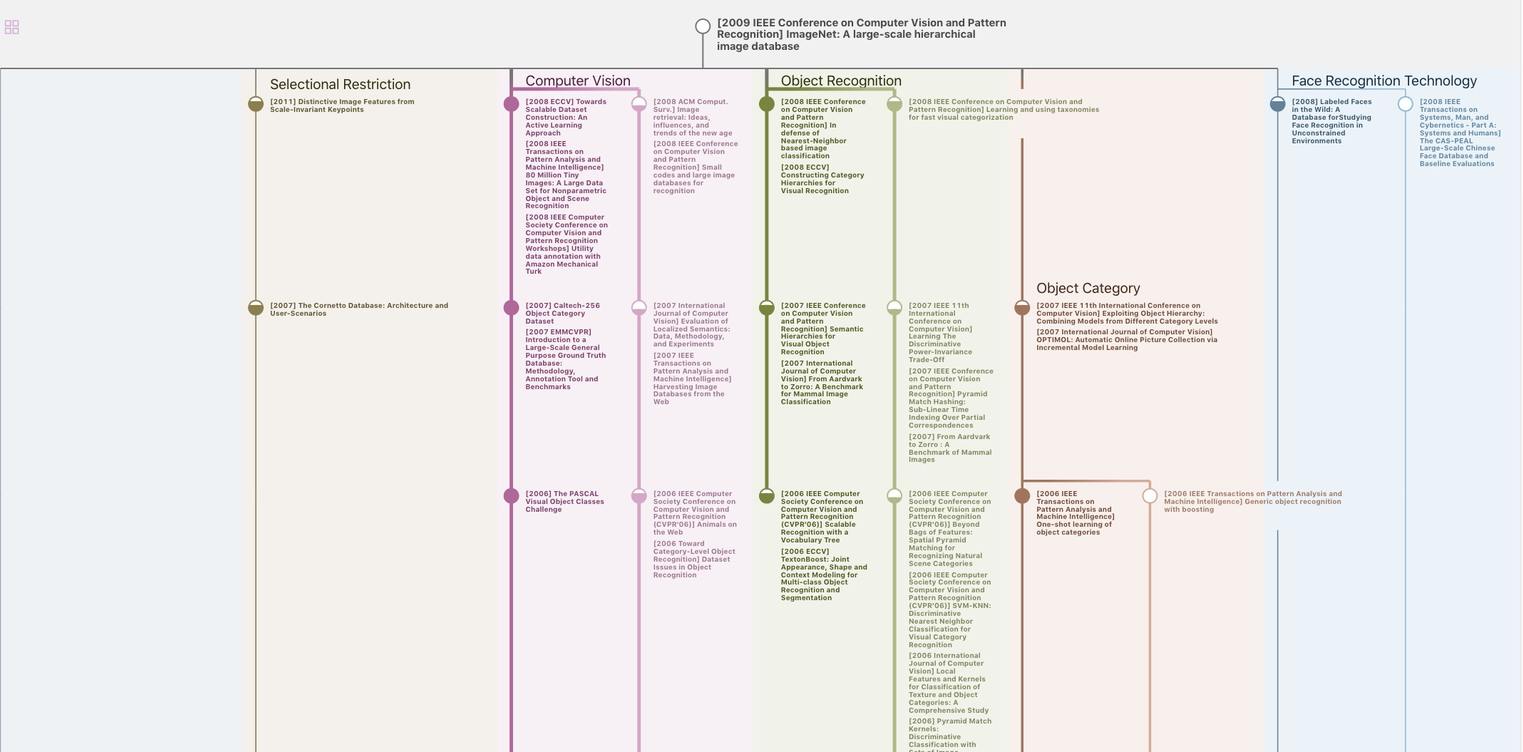
生成溯源树,研究论文发展脉络
Chat Paper
正在生成论文摘要