Personalized and Dynamic top-k Recommendation System using Context Aware Deep Reinforcement Learning
2021 IEEE 45th Annual Computers, Software, and Applications Conference (COMPSAC)(2021)
摘要
With the ever-increasing number of choices in just about every domain, ranging from e-commerce platforms to music and video streaming apps, it requires a highly personalized and best-in-class experience to make the users stay. Relevant, dynamic and smart item recommendations play a significant role in providing quality user experience. The majority of existing work either relies on explicit user feedback or gives static recommendations that poorly adapt to the changing user preferences. This paper proposes novel model architectures, namely, Sequential Feature Bandits(SFB) and Cross Feature Bandits(CFB) for providing online top-k context-aware recommendations. It leverages the item features, and uses implicit user feedback with a reinforcement learning backed dynamic algorithm. We use deep-q learning to maximize the cumulative estimated reward(CMR) and click-through rate(CTR) along with an experience replay strategy to prevent the proposed models from getting destabilized. Detailed experiments were conducted on a commercially available news article data set with implicit user-feedback and user-item context features. A thorough experimentation shows an average increase of 23.4% in CTR values and proves the effectiveness of the proposed SFB and CFB architectures for real-time recommendation.
更多查看译文
关键词
Context Aware Recommender Systems,Ubiquitous Computing,Reinforcement Learning,Intelligent and self-organizing computing,Deep Learning
AI 理解论文
溯源树
样例
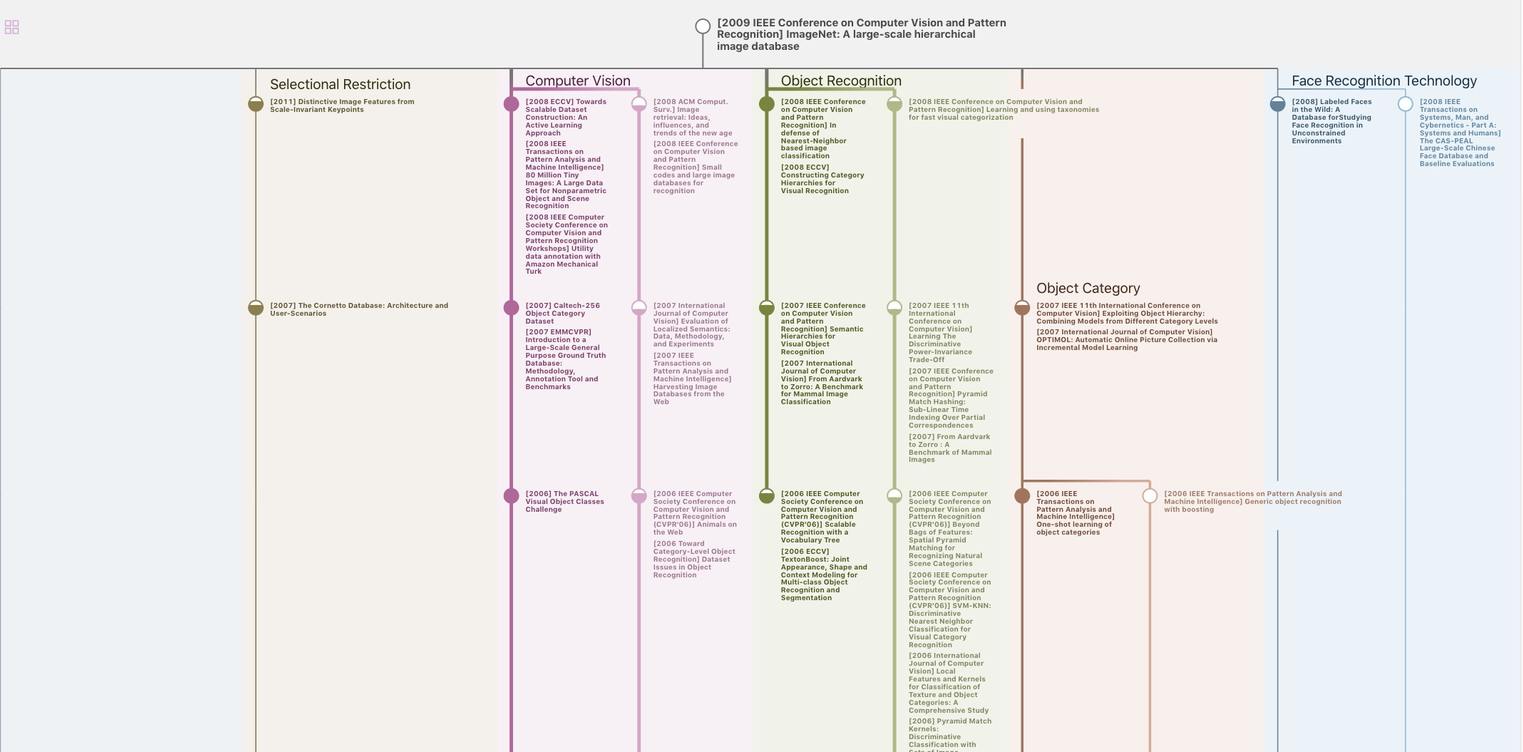
生成溯源树,研究论文发展脉络
Chat Paper
正在生成论文摘要