Under-bagging Nearest Neighbors for Imbalanced Classification
JOURNAL OF MACHINE LEARNING RESEARCH(2022)
摘要
In this paper, we propose an ensemble learning algorithm called under-bagging k-nearest neighbors (under-bagging k-NN ) for imbalanced classification problems. On the theoretical side, by developing a new learning theory analysis, we show that with properly chosen parameters, i.e., the number of nearest neighbors k, the expected sub-sample size s, and the bagging rounds B, optimal convergence rates for under-bagging k-NN can be achieved under mild assumptions w.r.t. the arithmetic mean (AM) of recalls. Moreover, we show that with a relatively small B, the expected sub-sample size s can be much smaller than the number of training data n at each bagging round, and the number of nearest neighbors k can be reduced simultaneously, especially when the data are highly imbalanced, which leads to substantially lower time complexity and roughly the same space complexity. On the practical side, we conduct numerical experiments to verify the theoretical results on the benefits of the under-bagging technique by the promising AM performance and efficiency of our proposed algorithm.
更多查看译文
关键词
Imbalanced classification,under-sampling,bagging,k-nearest neighbors,ensemble learning,arithmetic mean measure,optimal convergence rates,learning theory
AI 理解论文
溯源树
样例
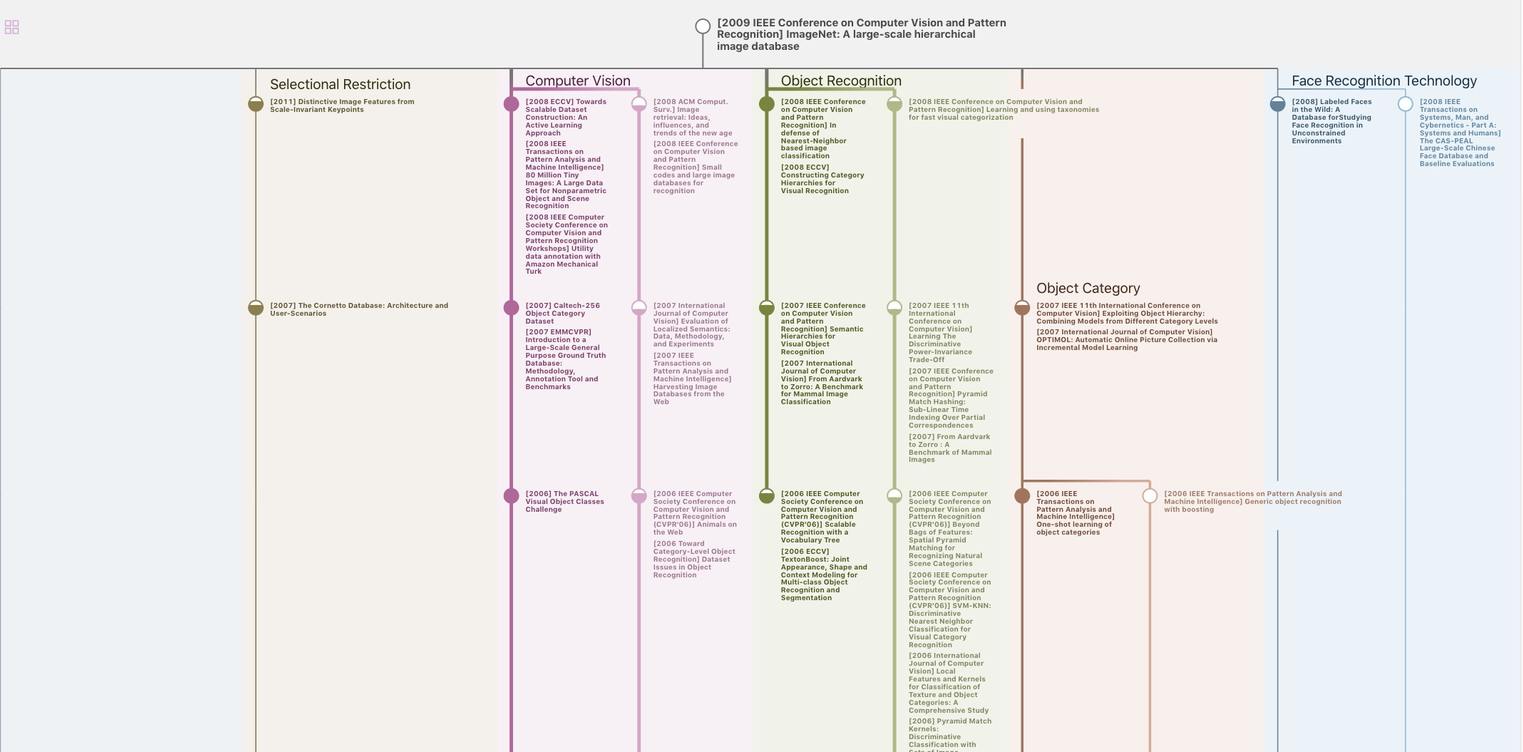
生成溯源树,研究论文发展脉络
Chat Paper
正在生成论文摘要