An On-Demand Charging for Connected Target Coverage in WRSNs Using Fuzzy Logic and Q-Learning
SENSORS(2021)
摘要
In wireless rechargeable sensor networks (WRSNs), a mobile charger (MC) moves around to compensate for sensor nodes' energy via a wireless medium. In such a context, designing a charging strategy that optimally prolongs the network lifetime is challenging. This work aims to solve the challenges by introducing a novel, on-demand charging algorithm for MC that attempts to maximize the network lifetime, where the term "network lifetime" is defined by the interval from when the network starts till the first target is not monitored by any sensor. The algorithm, named Fuzzy Q-charging, optimizes both the time and location in which the MC performs its charging tasks. Fuzzy Q-charging uses Fuzzy logic to determine the optimal charging-energy amounts for sensors. From that, we propose a method to find the optimal charging time at each charging location. Fuzzy Q-charging leverages Q-learning to determine the next charging location for maximizing the network lifetime. To this end, Q-charging prioritizes the sensor nodes following their roles and selects a suitable charging location where MC provides sufficient power for the prioritized sensors. We have extensively evaluated the effectiveness of Fuzzy Q-charging in comparison to the related works. The evaluation results show that Fuzzy Q-charging outperforms the others. First, Fuzzy Q-charging can guarantee an infinite lifetime in the WSRNs, which have a sufficient large sensor number or a commensurate target number. Second, in other cases, Fuzzy Q-charging can extend the time until the first target is not monitored by 6.8 times on average and 33.9 times in the best case, compared to existing algorithms.
更多查看译文
关键词
WRSN,Q-learning,on-demand charging algorithm,target coverage,connectivity
AI 理解论文
溯源树
样例
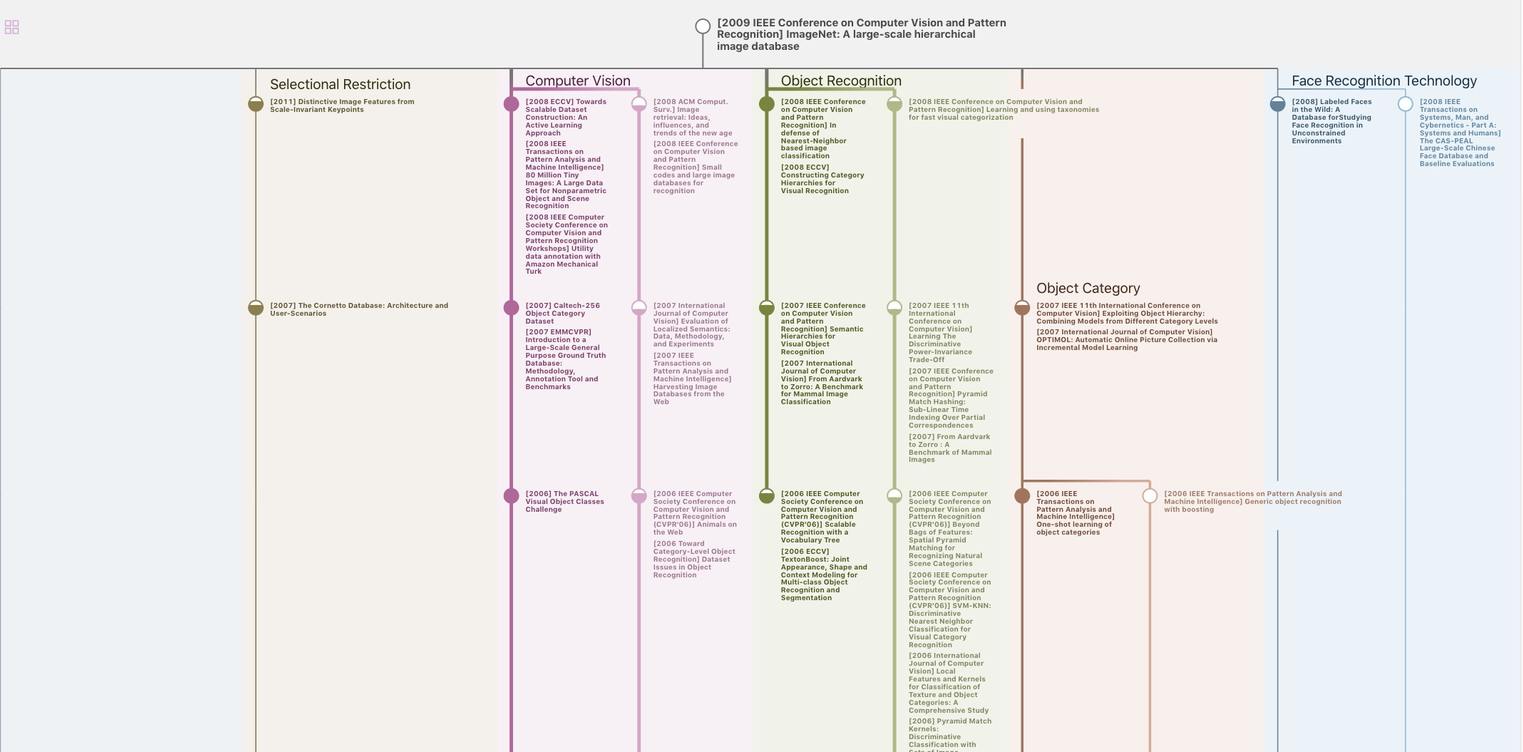
生成溯源树,研究论文发展脉络
Chat Paper
正在生成论文摘要