Sparse Gated Mixture-of-Experts to Separate and Interpret Patient Heterogeneity in EHR data
2021 IEEE EMBS International Conference on Biomedical and Health Informatics (BHI)(2021)
摘要
A chalenge in developing machine learning models for patient risk prediction involves addressing patient heterogeneity and interpreting the model outcome in clinical settings. Patient heterogeneity manifests as clinical differences among homogeneous patient subtypes in observational datasets. The discovery of such subtypes is helpful in precision medicine, where different risk factors from different patient would contribute differently to disease development and thus personalized treatment. In this paper, we use a Mixture-of-Experts (MoE) model and specifically couple it with a sparse gating network to handle patient heterogeneity for prediction and to aid interpretation of patient subtype separation. In experiment we show that with this sparsity we can improve the risk prediction. We therefore conduct empirical study to understand why and how the model learn to subtype patients from sparse training.
更多查看译文
关键词
Deep learning,electronic health record (EHR),mixture-of-experts
AI 理解论文
溯源树
样例
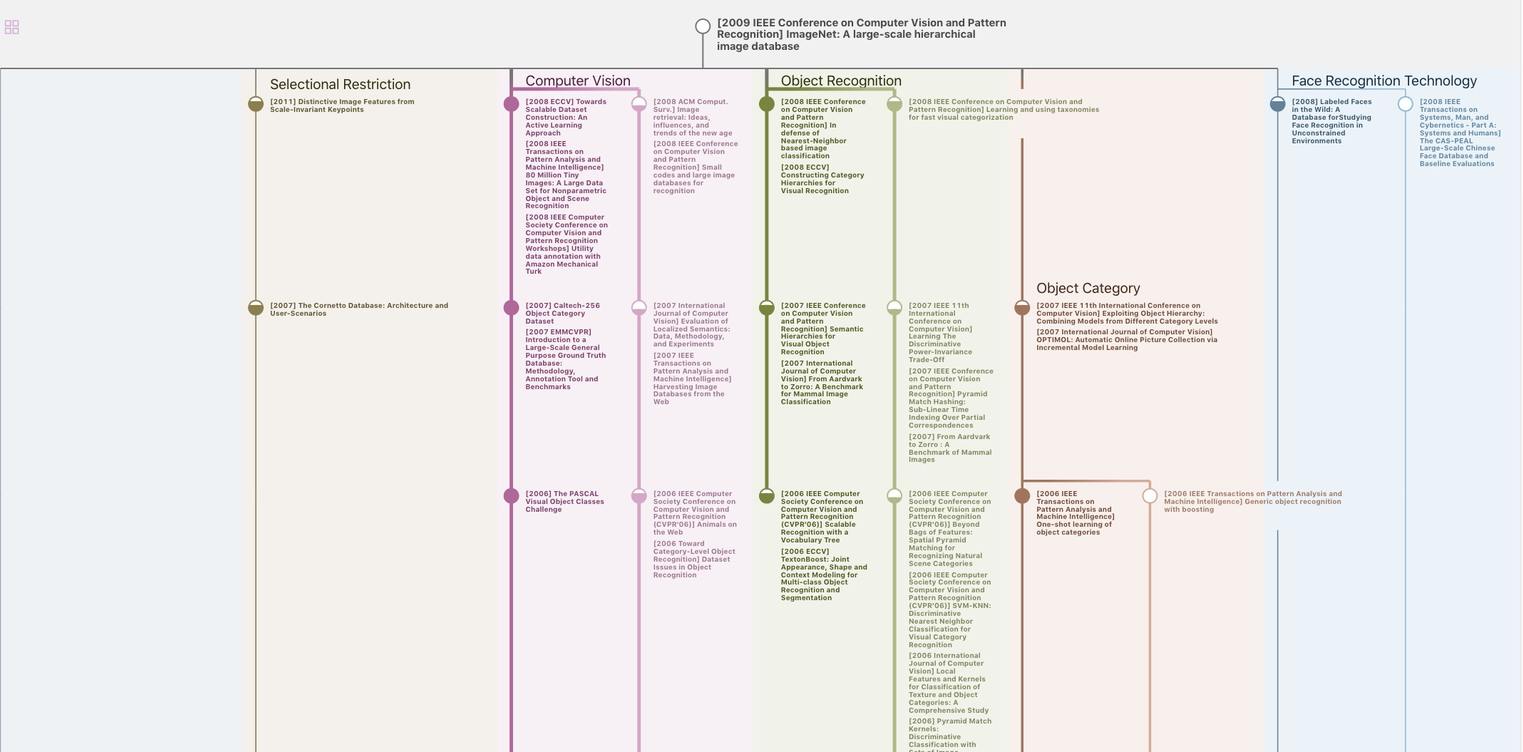
生成溯源树,研究论文发展脉络
Chat Paper
正在生成论文摘要