Color-based Superpixel Semantic Segmentation for Fire Data Annotation
IEEE International Conference on Fuzzy Systems(2021)
摘要
Image-based fire detection is a safety-critical task, which requires high-quality datasets to ensure performance guarantees in real scenarios. Automatic fire detection systems are in ever-increasing demand, but the limited number and size of open datasets, and lack of annotations, hinder model development. Solving this issue requires that experts dedicate a significant time to classify and segment fire events in image datasets. Towards building large-scale curated datasets, this paper presents a data annotation method that leverages semantic segmentation based on superpixel aggregation and color features. The approach introduces interpretable linguistic models that generate pixel-wise fire segmentation and annotations, which are explainable through simple fine-tunable rules that can support subsequent annotation validation by fire domain experts. The performance of the proposed algorithm is evaluated for relevant scenarios using a publicly available dataset, namely through the assessment of the segmentation quality and the labeling of fire color categories. The outcomes of this approach pave the way for creating large-scale datasets that can empower future deployments of learning-based architectures in fire detection systems.
更多查看译文
关键词
large-scale datasets,learning-based architectures,color-based superpixel semantic segmentation,fire data annotation,safety-critical task,high-quality datasets,automatic fire detection systems,open datasets,model development,segment fire events,image datasets,large-scale curated datasets,data annotation method,superpixel aggregation,color features,interpretable linguistic models,pixel-wise fire segmentation,simple fine-tunable rules,subsequent annotation validation,fire domain experts,relevant scenarios,publicly available dataset,segmentation quality,fire color categories
AI 理解论文
溯源树
样例
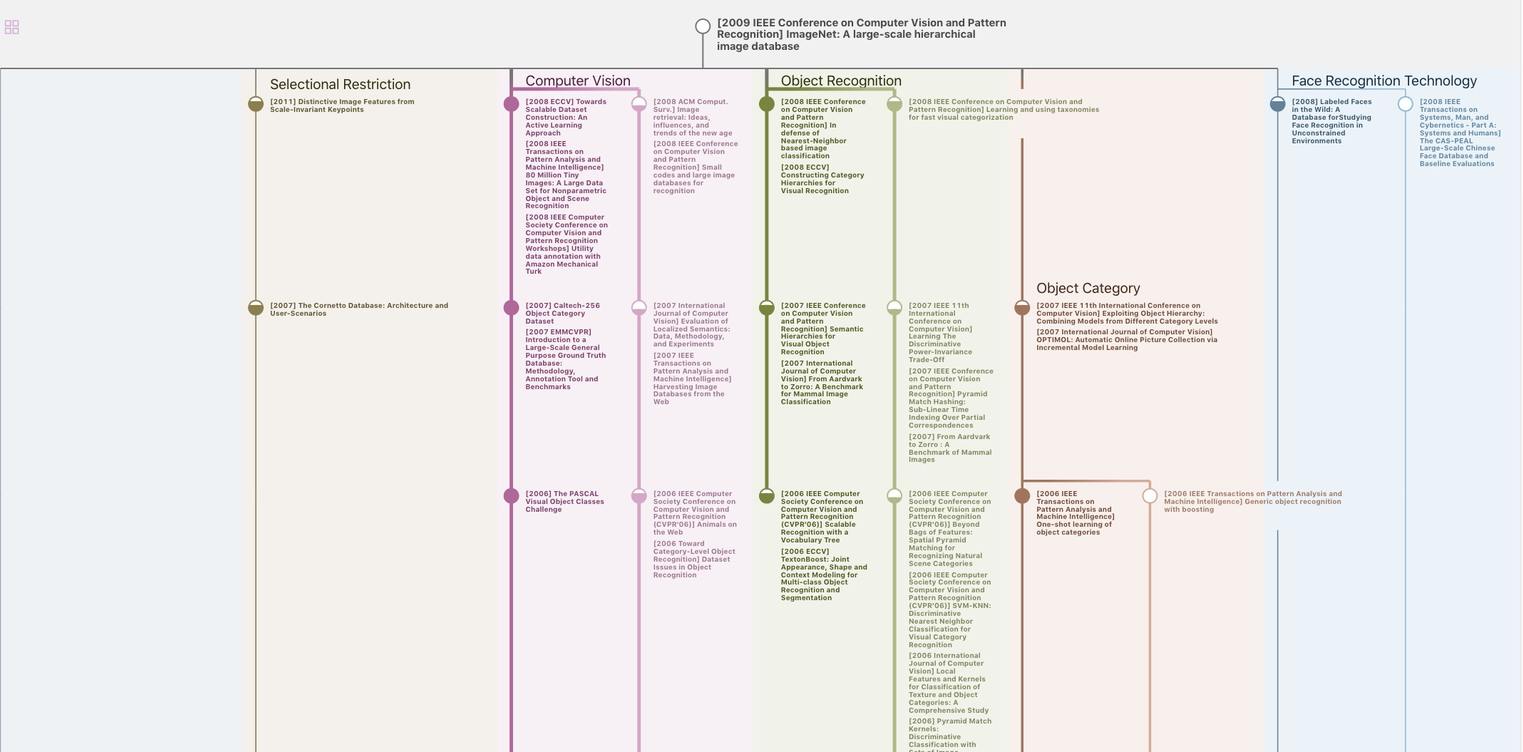
生成溯源树,研究论文发展脉络
Chat Paper
正在生成论文摘要