A Multi-Constraint Similarity Learning with Adaptive Weighting for Visible-Thermal Person Re-Identification.
IJCAI(2021)
摘要
The challenges of visible-thermal person re-identification (VT-ReID) lies in the inter-modality discrepancy and the intra-modality variations. An appropriate metric learning plays a crucial role in optimizing the feature similarity between the two modalities. However, most existing metric learning-based methods mainly constrain the similarity between individual instances or class centers, which are inadequate to explore the rich data relationships in the cross-modality data. Besides, most of these methods fail to consider the importance of different pairs, incurring an inefficiency and ineffectiveness of optimization. To address these issues, we propose a Multi-Constraint (MC) similarity learning method that jointly considers the cross-modality relationships from three different aspects, i.e., Instance-to-Instance (I2I), Center-to-Instance (C2I), and Center-to-Center (C2C). Moreover, we devise an Adaptive Weighting Loss (AWL) function to implement the MC efficiently. In the AWL, we first use an adaptive margin pair mining to select informative pairs and then adaptively adjust weights of mined pairs based on their similarity. Finally, the mined and weighted pairs are used for the metric learning. Extensive experiments on two benchmark datasets demonstrate the superior performance of the proposed over the state-of-the-art methods.
更多查看译文
AI 理解论文
溯源树
样例
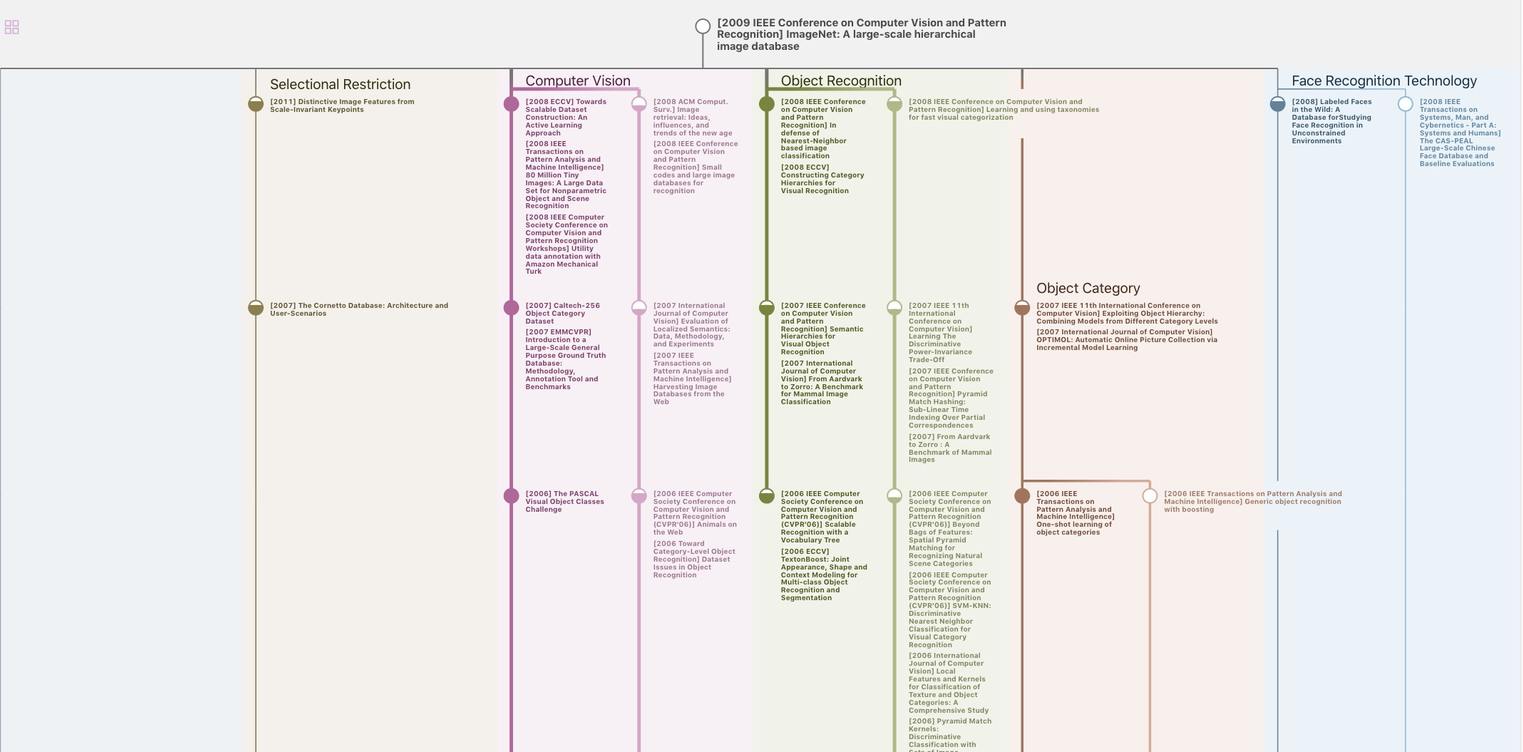
生成溯源树,研究论文发展脉络
Chat Paper
正在生成论文摘要