Improved Training of Generative Adversarial Networks Using Decision Forests.
IEEE Workshop/Winter Conference on Applications of Computer Vision(2021)
摘要
Whilst Generative Adversarial Networks (GANs) have gained a reputation as powerful generative models, they are notoriously difficult to train and suffer from instability in optimisation. Recent methods for tackling this drawback have typically approached it by inducing better behaviour on the discriminator component of the GAN; these include loss function modification, gradient regularisation and weight normalisation to create a discriminator that is well-behaved from a Lipschitz perspective. In this paper, we propose a novel and orthogonal contribution which modifies the architecture of a GAN. Our method embeds the powerful discriminating capabilities inherent in decision forests within the discriminator of a GAN. Empirically, we test the effectiveness of our approach on the CIFAR-10, Oxford Flowers and CUB Birds datasets. We show that our technique is easy to incorporate into existing GAN baselines and offers improvements on Fréchet-Inception Distance (FID) scores by as high as 56.1% over several GAN baselines.
更多查看译文
关键词
discriminator component,loss function modification,gradient regularisation,weight normalisation,decision forests,generative adversarial network training,GAN architecture
AI 理解论文
溯源树
样例
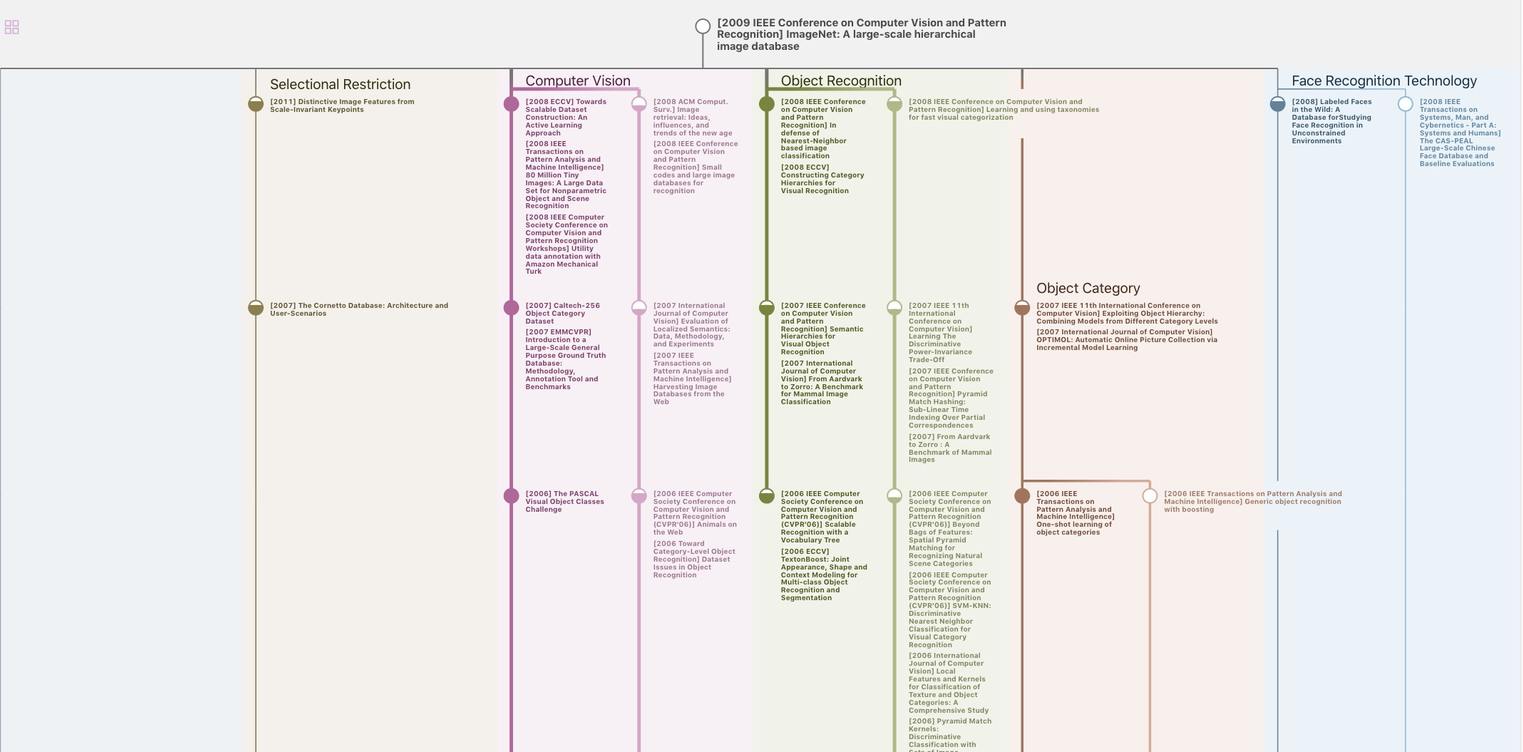
生成溯源树,研究论文发展脉络
Chat Paper
正在生成论文摘要