Data assimilation of synthetic data as a novel strategy for predicting disease progression in alopecia areata
Mathematical medicine and biology(2021)
摘要
The goal of patient-specific treatment of diseases requires a connection between clinical observations with models that are able to accurately predict the disease progression. Even when realistic models are available, it is very difficult to parameterize them and often parameter estimates that are made using early time course data prove to be highly inaccurate. Inaccuracies can cause different predictions, especially when the progression depends sensitively on the parameters. In this study, we apply a Bayesian data assimilation method, where the data are incorporated sequentially, to a model of the autoimmune disease alopecia areata that is characterized by distinct spatial patterns of hair loss. Using synthetic data as simulated clinical observations, we show that our method is relatively robust with respect to variations in parameter estimates. Moreover, we compare convergence rates for parameters with different sensitivities, varying observational times and varying levels of noise. We find that this method works better for sparse observations, sensitive parameters and noisy observations. Taken together, we find that our data assimilation, in conjunction with our biologically inspired model, provides directions for individualized diagnosis and treatments.
更多查看译文
关键词
personalized medicine,data assimilation,autoimmunity,alopecia areata
AI 理解论文
溯源树
样例
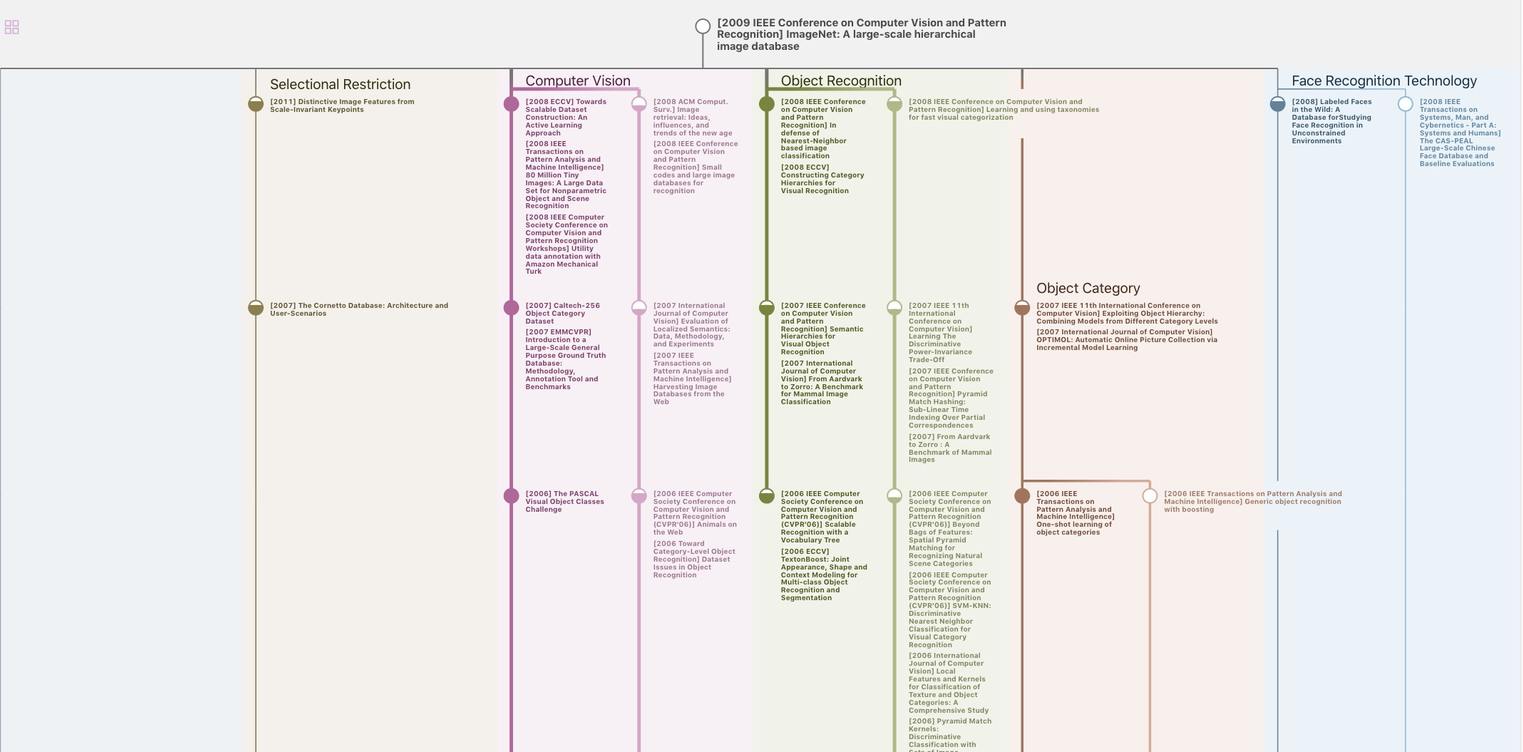
生成溯源树,研究论文发展脉络
Chat Paper
正在生成论文摘要