Knowledge Improvement and Diversity under Interaction-Driven Adaptation of Learned Ontologies.
AAMAS(2021)
摘要
When agents independently learn knowledge, such as ontologies, about their environment, it may be diverse, incorrect or incomplete. This knowledge heterogeneity could lead agents to disagree, thus hindering their cooperation. Existing approaches usually deal with this interaction problem by relating ontologies, without modifying them, or, on the contrary, by focusing on building common knowledge. Here, we consider agents adapting ontologies learned from the environment in order to agree with each other when cooperating. In this scenario, fundamental questions arise: Do they achieve successful interaction? Can this process improve knowledge correctness? Do all agents end up with the same ontology? To answer these questions, we design a two-stage experiment. First, agents learn to take decisions about the environment by classifying objects and the learned classifiers are turned into ontologies. In the second stage, agents interact with each other to agree on the decisions to take and modify their ontologies accordingly. We show that agents indeed reduce interaction failure, most of the time they improve the accuracy of their knowledge about the environment, and they do not necessarily opt for the same ontology.
更多查看译文
关键词
ontologies,knowledge,adaptation,diversity,interaction-driven
AI 理解论文
溯源树
样例
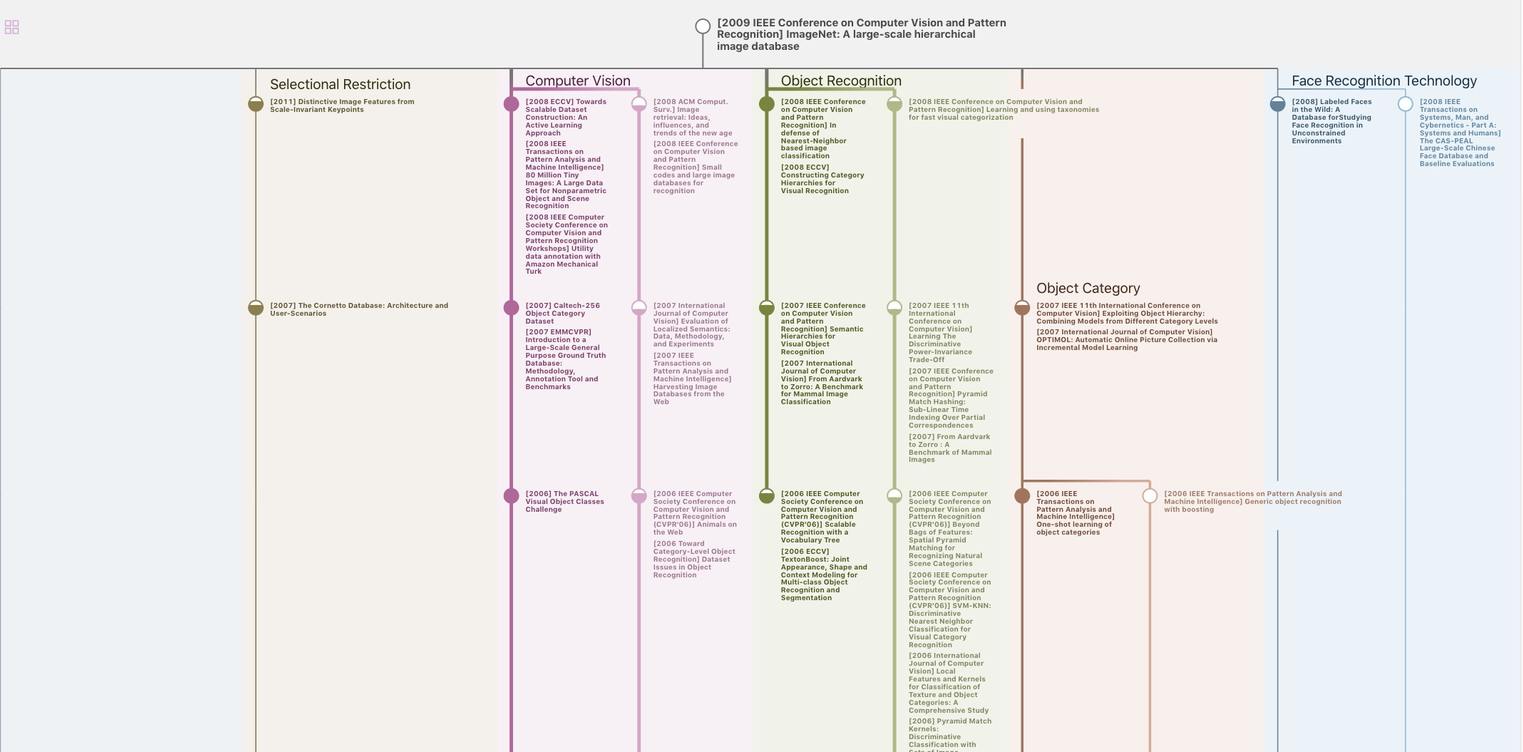
生成溯源树,研究论文发展脉络
Chat Paper
正在生成论文摘要