A hybrid binary grey wolf optimizer for selection and reduction of reference points with extreme learning machine approach on local GNSS/leveling geoid determination
APPLIED SOFT COMPUTING(2021)
摘要
Modeling and optimization from natural phenomena and observations of the physical earth is an extremely important issue. In the light of the developments in computer and artificial intelligence technologies, the applications of learning-based modeling and optimization techniques in all kinds of study fields are increasing. In this research, the applicability of four different state-of-the-art metaheuristic algorithms which are Particle swarm optimization (PSO), Tree-Seed Algorithm (TSA), Artificial Bee Colony (ABC) algorithm, and Grey Wolf Optimizer (GWO), in local GNSS/leveling geoid studies have been examined. The most suitable geoid model has been tried to be obtained by using different reference points via the well-known machine learning algorithms, Artificial Neural Network (ANN) and Extreme Learning Machine (ELM), at the existing GNSS/leveling points in Burdur city of Turkey. In this study, eight different hybrid approaches are proposed by using each metaheuristic algorithm together with machine learning methods. By using these hybrid approaches, the model closest to the minimum number of reference points has been tried to be obtained. Furthermore, the performance of the hybrid approaches has been compared. According to the comparisons, the hybrid approach performed with GWO and ELM has achieved better results than other proposed hybrid approaches. As a result of the research, it has been seen that the most suitable local GNSS/Leveling geoid can be determined with a lower number of reference points in an appropriate distribution. (C) 2021 Elsevier B.V. All rights reserved.
更多查看译文
关键词
GNSS/leveling geoid,Modeling,Optimization,Grey wolf optimizer,Extreme learning machine
AI 理解论文
溯源树
样例
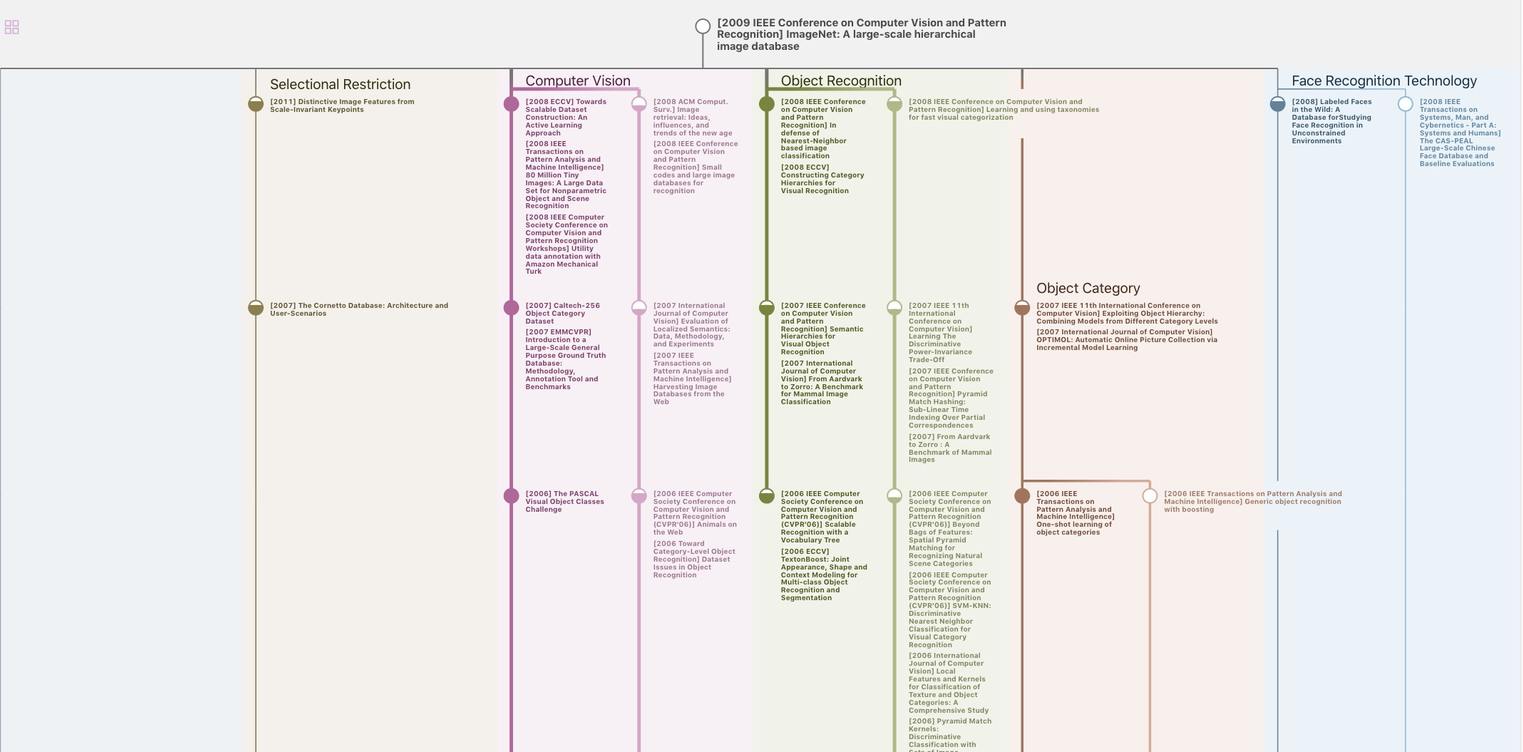
生成溯源树,研究论文发展脉络
Chat Paper
正在生成论文摘要