Multi-Parametric Mri Based Radiomics With Tumor Subregion Partitioning For Differentiating Benign And Malignant Soft-Tissue Tumors
BIOMEDICAL SIGNAL PROCESSING AND CONTROL(2021)
摘要
Objective: This study aims to explore MRI-based tumoral and intratumoral radiomics approaches on distinguishing malignant from benign soft-tissue tumors using handcrafted and deep learning-based features. Methods: A set of 82 patients underwent contrast-enhanced (CE) T1 and T1-weighted imaging (T1WI) MRI scans were enrolled between Jan. 2017 and Sep. 2019. The whole tumor regions were segmented by an unsupervised kmeans algorithm. Radiomics handcrafted and deep learning-based features were extracted and selected from the whole tumor regions and intratumor subregions, and used to develop radiomics models based on a k-nearest neighbors (KNN) classifier. A radiomics nomogram was developed for potential clinical uses. The receiver operating characteristic (ROC) analysis was used to evaluate the discriminative performance of each model. Calibration and decision curve analysis were applied to evaluate the nomogram. Results: Our findings revealed that the active subregion in the CE-T1 and the whole tumor region in the T1WI MRI are the most discriminative regions. A fusion radiomics nomogram was established and achieved the best diagnostic performance with the area under the ROC curve (AUC) of 0.941 (SEN = 0.789, SPE = 1.000) in the training cohort and 0.922 (SEN = 0.667, SPE = 0.921) in the validation cohort. Conclusions: The proposed tumoral and intratumoral radiomics were potentially clinical valuable and could improve the application of computer-aided diagnosis (CAD) in soft-tissue tumor diagnosis.
更多查看译文
关键词
Soft-Tissue tumor, MRI, Radiomics, Deep learning
AI 理解论文
溯源树
样例
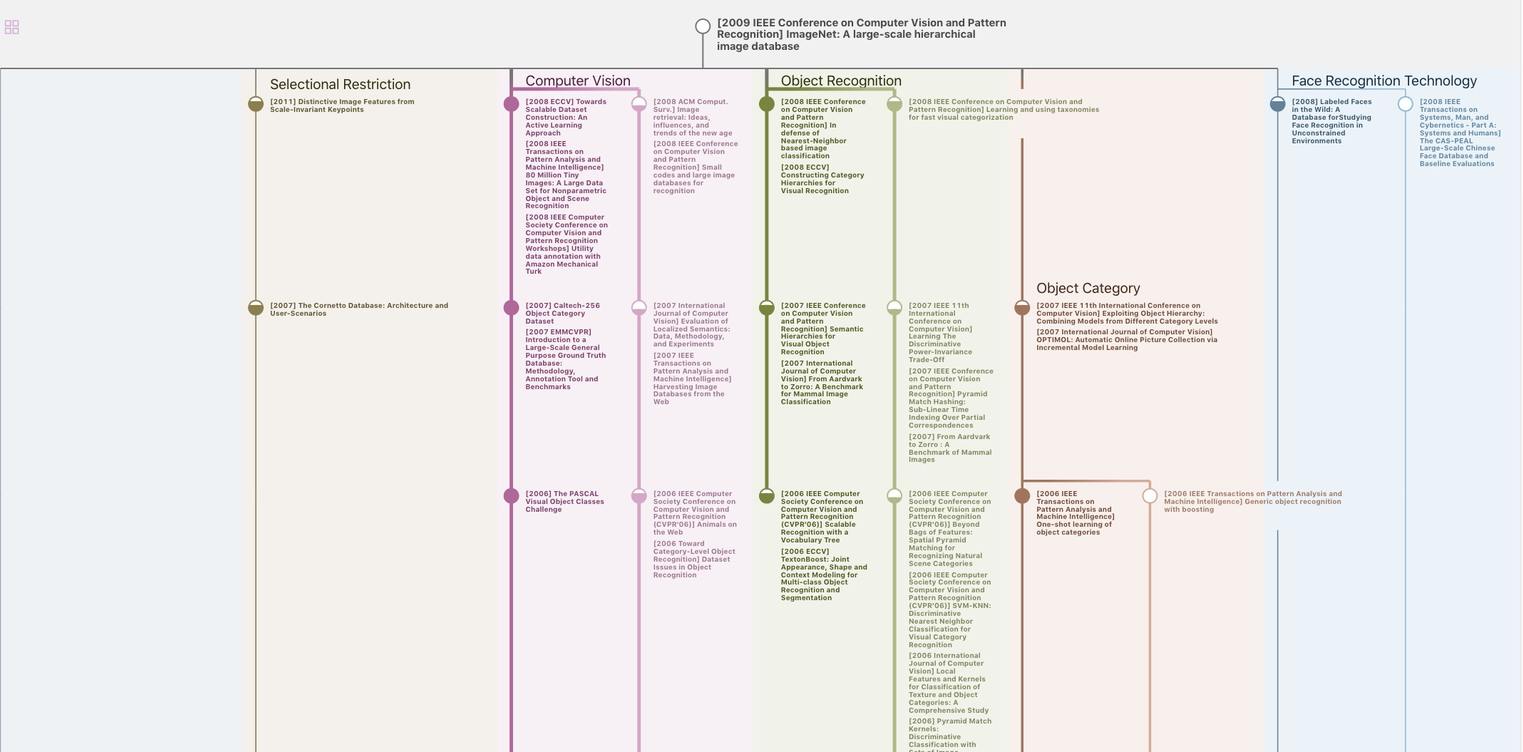
生成溯源树,研究论文发展脉络
Chat Paper
正在生成论文摘要