Author Response for "current and Future Deep Learning Algorithms for MS/MS‐based Small Molecule Structure Elucidation"
Rapid communications in mass spectrometry/RCM Rapid communications in mass spectrometry(2021)
摘要
RATIONALE:Structure elucidation of small molecules has been one of the cornerstone applications of mass spectrometry for decades. Despite the increasing availability of software tools, structure elucidation from tandem mass spectrometry (MS/MS) data remains a challenging task, leaving many spectra unidentified. However, as an increasing number of reference MS/MS spectra are being curated at a repository scale and shared on public servers, there is an exciting opportunity to develop powerful new deep learning (DL) models for automated structure elucidation.ARCHITECTURES:Recent early-stage DL frameworks mostly follow a "two-step approach" that translates MS/MS spectra to database structures after first predicting molecular descriptors. The related architectures could suffer from: (1) computational complexity because of the separate training of descriptor-specific classifiers, (2) the high dimensional nature of mass spectral data and information loss due to data preprocessing, (3) low substructure coverage and class imbalance problem of predefined molecular fingerprints. Inspired by successful DL frameworks employed in drug discovery fields, we have conceptualized and designed hypothetical DL architectures to tackle the above issues. For (1), we recommend multitask learning to achieve better performance with fewer classifiers by grouping structurally related descriptors. For (2) and (3), we introduce feature engineering to extract condensed and higher-order information from spectra and structure data. For instance, encoding spectra with subtrees and pre-calculated spectral patterns add peak interactions to the model input. Encoding structures with graph convolutional networks incorporates connectivity within a molecule. The joint embedding of spectra and structures can enable simultaneous spectral library and molecular database search.CONCLUSIONS:In principle, given enough training data, adapted DL architectures, optimal hyperparameters and computing power, DL frameworks can predict small molecule structures, completely or at least partially, from MS/MS spectra. However, their performance and general applicability should be fairly evaluated against classical machine learning frameworks.
更多查看译文
AI 理解论文
溯源树
样例
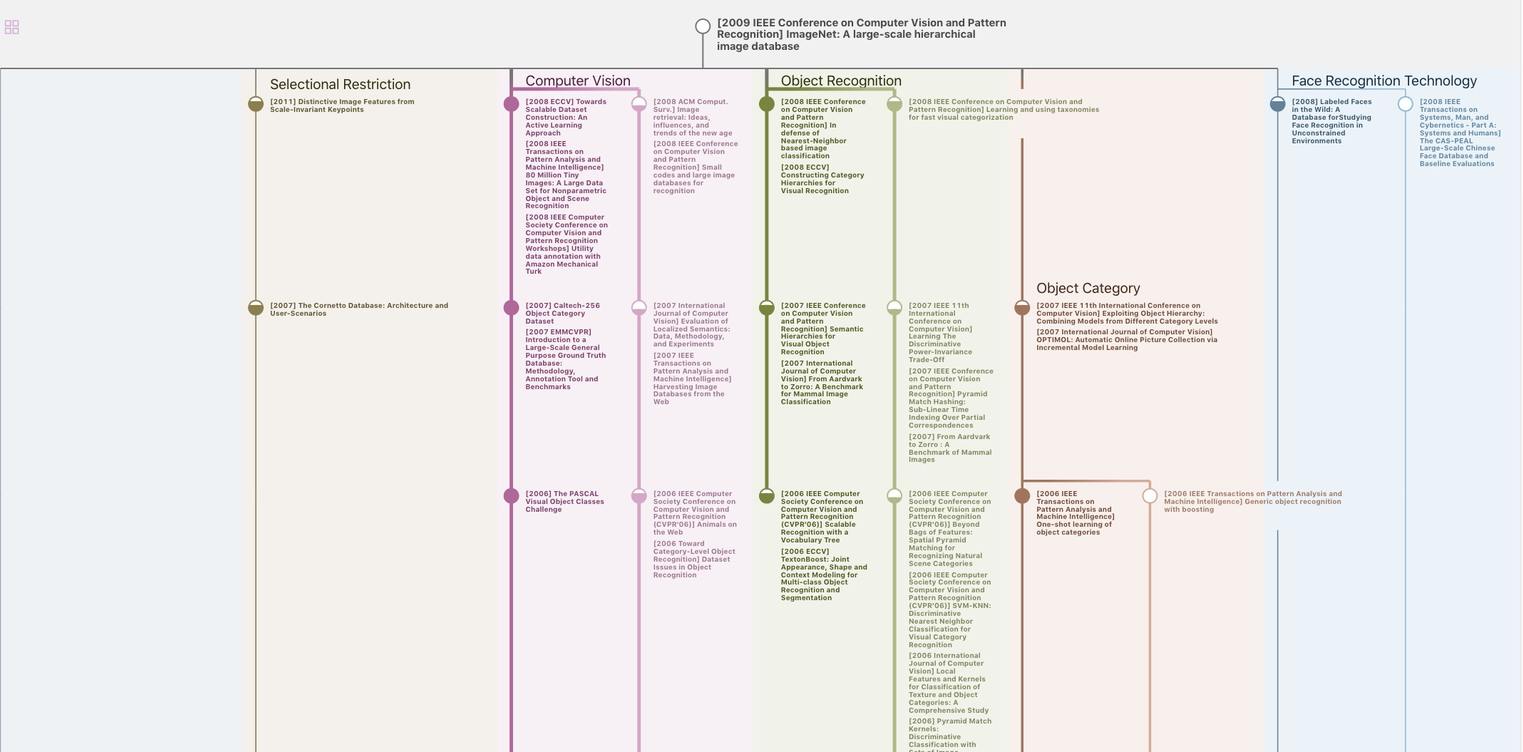
生成溯源树,研究论文发展脉络
Chat Paper
正在生成论文摘要