Generic BCI Classifiers for Discrimination of Motor Imagery of Left/Right Steps and Forward Walking.
2021 9TH IEEE INTERNATIONAL WINTER CONFERENCE ON BRAIN-COMPUTER INTERFACE (BCI)(2021)
摘要
Brain-computer interfaces (BCI) have been used to control the gait of a virtual self-avatar, using motor imagery of the feet, in order to restore motor control in gait rehabilitation. The considerable training time required to use such a BCI is an obstacle to their adoption in a clinical setting. One technique used to enhance BCI control and to shorten training time is to eliminate offline calibration using a generic classifier that is pre-trained over many participants, each performing many trials. This paper investigates the performance of generic models that were derived from 2 datasets, each containing the data of 20 participants. They participated in a sequential training to control the gait of an avatar when cued to imagine a single step forward using their left or right foot, or to start walking forward. The avatar moved in response to two calibrated RLDA classifiers that used the $\mu$ PSD over the foot area of the motor cortex as features. The generic models were tested on the offline and online data of the participants. The models performed as well as models obtained from participant-specific offline data with a mean performance of 86%. The results show the possibility of designing a participant-independent, zero-training lower-limb MI-BCI.
更多查看译文
关键词
Brain-computer interface,Virtual reality,EEG,Classification,Avatar,Gait,Generic classifier
AI 理解论文
溯源树
样例
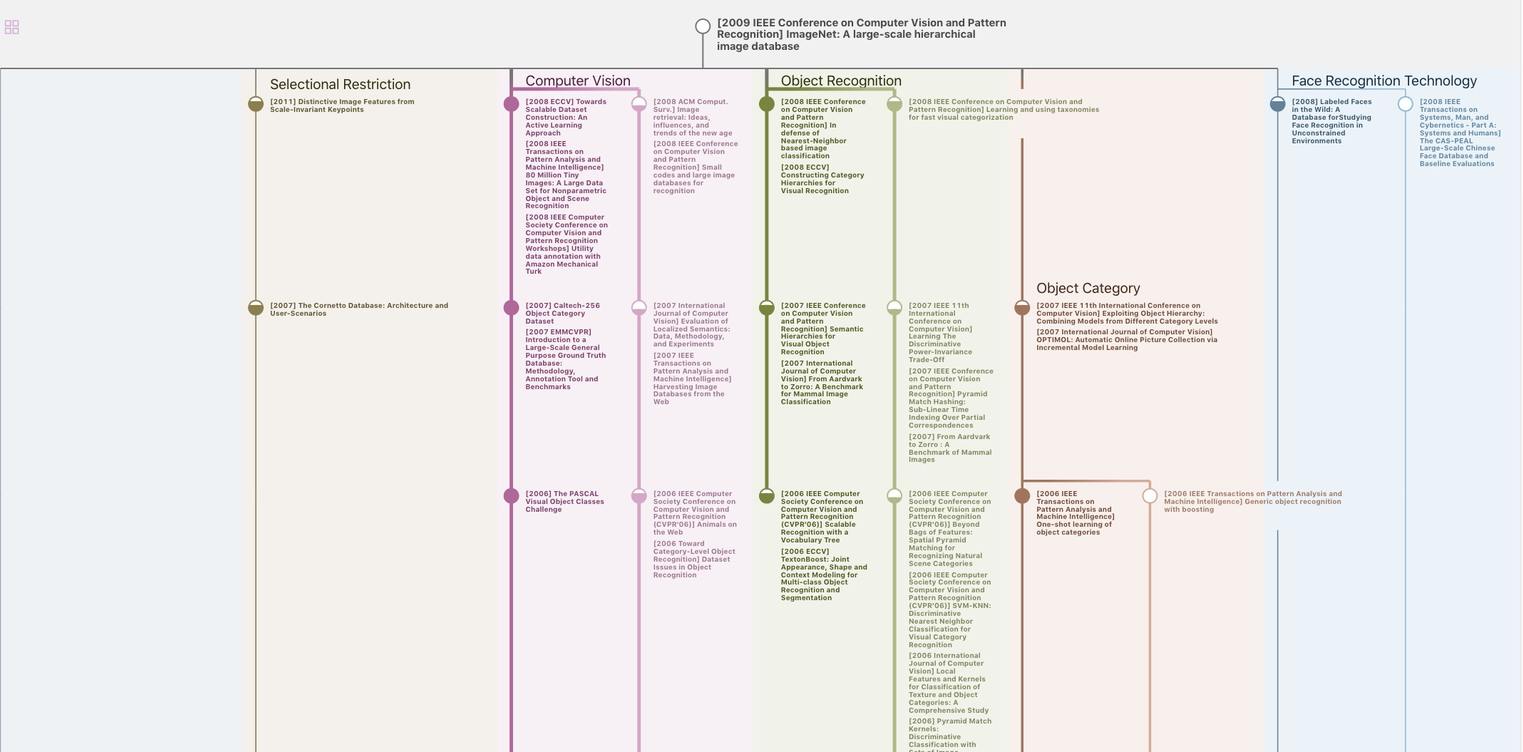
生成溯源树,研究论文发展脉络
Chat Paper
正在生成论文摘要