Theoretically Improving Graph Neural Networks via Anonymous Walk Graph Kernels
International World Wide Web Conference(2021)
摘要
Graph neural networks (GNNs) have achieved tremendous success in graph mining. However, the inability of GNNs to model substructures in graphs remains a significant drawback. Specifically, message-passing GNNs (MPGNNs), as the prevailing type of GNNs, have been theoretically shown unable to distinguish, detect or count many graph substructures. While efforts have been paid to complement the inability, existing works either rely on pre-defined substructure sets, thus being less flexible, or are lacking in theoretical insights. In this paper, we propose GSKN1, a GNN model with a theoretically stronger ability to distinguish graph structures. Specifically, we design GSKN based on anonymous walks (AWs), flexible substructure units, and derive it upon feature mappings of graph kernels (GKs). We theoretically show that GSKN provably extends the 1-WL test, and hence the maximally powerful MPGNNs from both graph-level and node-level viewpoints. Correspondingly, various experiments are leveraged to evaluate GSKN, where GSKN outperforms a wide range of baselines, endorsing the analysis.
更多查看译文
关键词
Graph Convolutional Network,Structural Patterns,Graph Kernels
AI 理解论文
溯源树
样例
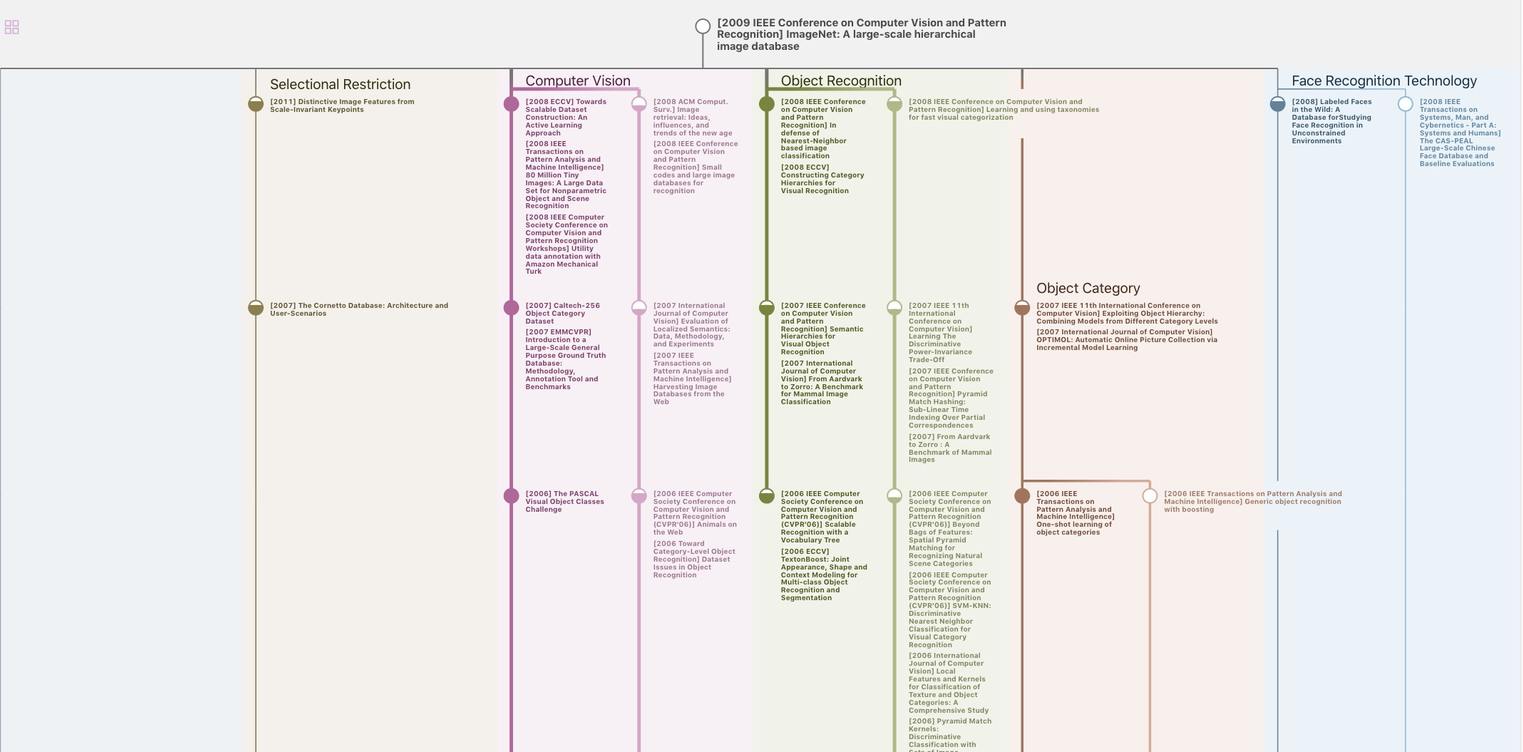
生成溯源树,研究论文发展脉络
Chat Paper
正在生成论文摘要