Credit Scoring Model Based on a Novel Group Feature Selection Method: the Case of Chinese Small-Sized Manufacturing Enterprises.
Journal of the Operational Research Society(2021)
摘要
In building a predictive credit scoring model, feature selection is an essential pre-processing step that can improve the predictive accuracy and comprehensibility of models. In this study, we select the optimal feature subset based on group feature selection in lieu of the individual feature selection method, to establish a credit scoring model for small manufacturing enterprises. In our methodology, we first select a group of features using the 0-1 programming method, with the objective function of maximising the Gini coefficient (GINI) of the credit score to identify the possibility of default. Then we introduce constraints to remove any redundant features in the same subset, provided they reflect the same information. Finally, we assign weights to different features according to the Gini coefficient, ensuring that the weight of the features reflects their discriminatory power. Our empirical results show that the selection of a set of features more effectively identifies default status than the individual feature selection approach. Moreover, a rating system with more features does not necessarily have better discriminatory power. As the number of features exceeds the optimum number of features selected, the system's discriminatory ability begins to decrease.
更多查看译文
关键词
Credit scoring,feature selection,default discrimination,Gini coefficient,0-1 programming
AI 理解论文
溯源树
样例
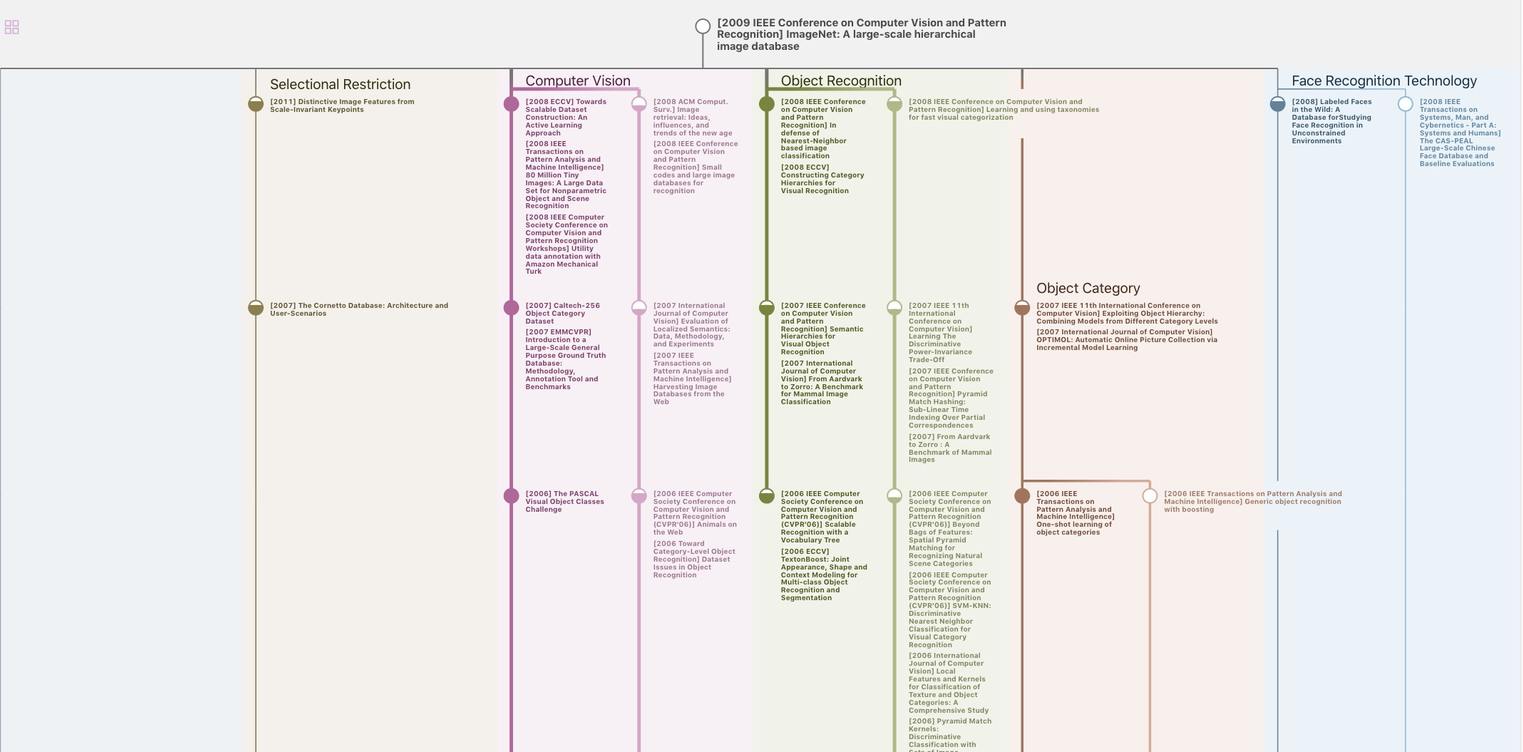
生成溯源树,研究论文发展脉络
Chat Paper
正在生成论文摘要