Prediction And Analysis Of Multiple Sites And Inhibitors Of Sars-Cov-2 Proteins
BIOPHYSICAL JOURNAL(2021)
摘要
In the current COVID-19 pandemic, it is critical to understand, as swiftly as possible, how the viral proteins function and how their function might be modulated. The machine learning method Partial Order Optimum Likelihood (POOL) is used to predict binding sites in protein structures from SARS-CoV-2, the virus that causes COVID-19. Using the 3D structure of each protein as input, POOL uses computed electrostatic and chemical properties to predict the amino acids that are biochemically active, including residues in catalytic sites, allosteric sites, and other secondary sites. Docking studies are then performed to predict ligands that bind to each of these predicted sites. For instance, for the x-ray crystal structures of the main protease, POOL predicts two sites: the known catalytic site containing the catalytic dyad His41 and Cys145 and a second nearby site on an adjacent face of the protein surface. The x-ray crystal structure of the SARS-CoV-2 2’-O-ribose RNA methyltransferase (NSP16) protein has been reported in complex with its activating partner NSP10 and with two bound ligands, S-adenosylmethionine (SAM) and β-D-fructopyranose (BDF). POOL predicts three binding sites, including the catalytic SAM-binding site, the BDF binding site on the opposite side, and a third site adjacent to the catalytic / SAM-binding site. Predicted binding ligands (including selected compounds from the ZINC and Enamine databases, Chemical Abstract Service database compounds, and COVID-specific libraries from Enamine and Life Chemicals) are reported for several SARS-CoV-2 proteins. Kinetics assays to test for catalytic activity of the main protease and of 2’-O-ribose RNA methyltransferase in the presence of predicted binding ligands with high scores are underway. Theoretical and experimental methods are aimed at identifying molecules having inhibitory effects on the function of viral proteins. Supported by NSF CHE-2030180.
更多查看译文
关键词
proteins,inhibitors,sars-cov
AI 理解论文
溯源树
样例
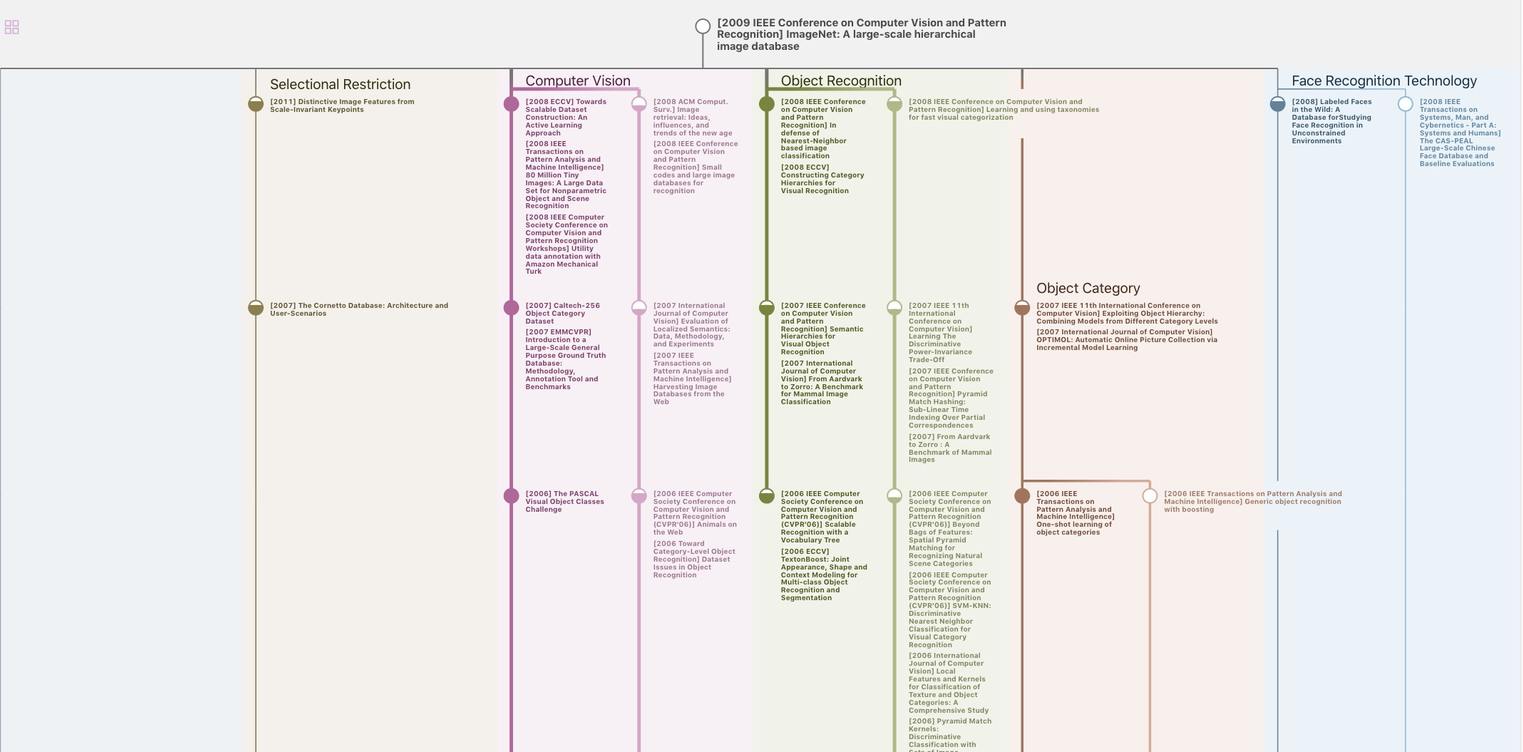
生成溯源树,研究论文发展脉络
Chat Paper
正在生成论文摘要