Two-Level Attention With Two-Stage Multi-Task Learning For Facial Emotion Recognition
JOURNAL OF VISUAL COMMUNICATION AND IMAGE REPRESENTATION(2019)
Abstract
Compared with facial emotion estimation on categorical model, dimensional emotion estimation can describe numerous emotions more accurately. Most prior works of dimensional emotion estimation only considered laboratory data and used video, speech or other multi-modal features. Compared with other modal data, static images has superiorities of accessibility, which is more conducive to the emotion estimation in real world. In this paper, a two-level attention with two-stage multi-task learning (2Att-2Mt) framework is proposed for facial emotion estimation on only static images. Firstly, the features of corresponding region (position level features) are extracted and enhanced automatically by first-level attention mechanism. Then, we utilize Bi-directional Recurrent Neural Network (Bi-RNN) with self-attention (second-level attention) to make full use of the relationship features of different layers (layer-level features) adaptively. And then, we propose a two-stage multi-task learning structure, which exploits categorical representations to ameliorate the dimensional representations and estimate valence and arousal simultaneously in view of the inherent complexity of dimensional representations and correlation of the two targets. The quantitative results conducted on AffectNet dataset show significant advancement on Concordance Correlation Coefficient(CCC) and Root Mean Square Error (RMSE), illustrating the superiority of the proposed framework. Besides, extensive comparative experiments have also fully demonstrated the effectiveness of different components (2Att and 2Mt) in our framework. (C) 2019 Published by Elsevier Inc.
MoreTranslated text
Key words
Facial emotion recognition,Attention mechanism,Multi-task learning,Valence-arousal dimension
AI Read Science
Must-Reading Tree
Example
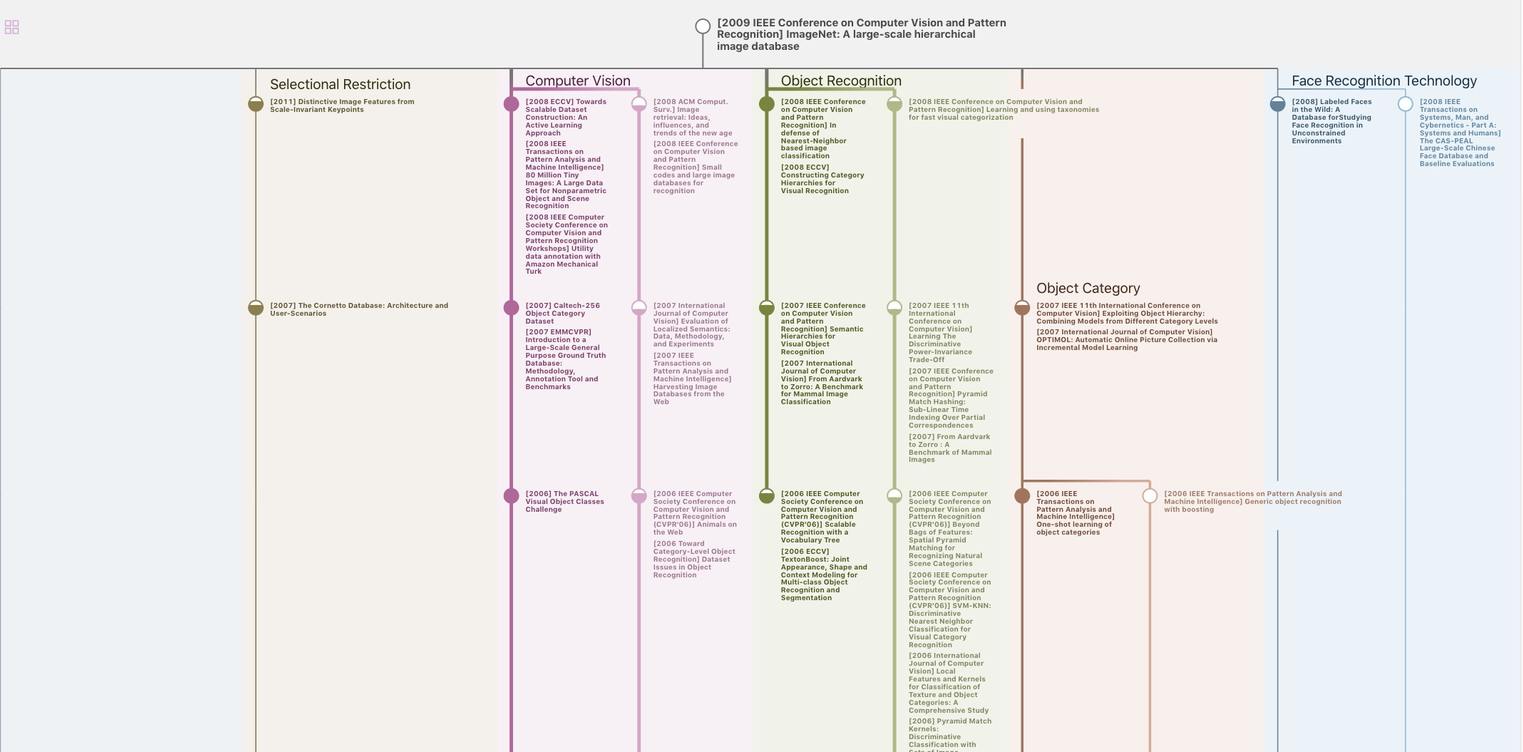
Generate MRT to find the research sequence of this paper
Chat Paper
Summary is being generated by the instructions you defined